解释 branch1x1 = conv2d_bn(x, 64, 1, 1) branch5x5 = conv2d_bn(x, 48, 1, 1) branch5x5 = conv2d_bn(branch5x5, 64, 5, 5) branch3x3dbl = conv2d_bn(x, 64, 1, 1) branch3x3dbl = conv2d_bn(branch3x3dbl, 96, 3, 3) branch3x3dbl = conv2d_bn(branch3x3dbl, 96, 3, 3) branch_pool = AveragePooling2D((3, 3), strides=(1, 1), padding='same')(x) branch_pool = conv2d_bn(branch_pool, 32, 1, 1) # 64+64+96+32 = 256 x = layers.concatenate( [branch1x1, branch5x5, branch3x3dbl, branch_pool], axis=3, name='mixed0')
时间: 2023-09-22 09:08:23 浏览: 39
这段代码是在构建一个Inception module,也就是Google Inception V1网络中使用的模块。该模块主要由四个分支构成,每个分支都有不同的卷积核大小和数量,最终将四个分支的输出在通道维度上进行拼接。
具体来说,第一个分支是一个1x1的卷积层,输出通道数为64。第二个分支也是一个1x1的卷积层,输出通道数为48,然后接一个5x5的卷积层,输出通道数为64。第三个分支是一个1x1的卷积层,输出通道数为64,然后接一个3x3的卷积层,输出通道数为96,最后再接一个3x3的卷积层,输出通道数为96。第四个分支是一个3x3的平均池化层,步长为1,填充方式为same,输出通道数为32,然后再接一个1x1的卷积层。
最后,将四个分支的输出在通道维度上进行拼接,形成一个维度为256的输出。
相关问题
branch1x1 = conv2d_bn(x, 32, 1, 1)后面加BN层
在使用conv2d_bn函数之后可以添加Batch Normalization(BN)层。Batch Normalization是一种常用的正则化技术,用于加速深度神经网络的训练过程并提升模型的性能。
可以在conv2d_bn函数的输出上添加BN层,代码如下所示:
```python
branch1x1 = conv2d_bn(x, 32, 1, 1)
branch1x1 = BatchNormalization()(branch1x1)
```
这样就在conv2d_bn函数的输出上添加了一个BN层。BatchNormalization()(branch1x1)表示对branch1x1的输出进行BN操作,并将结果赋值给branch1x1变量。
branch1x1 = conv2d_bn(x, 32, 1, 1)用ghostmodule代替1*1卷积
可以使用GhostModule来代替1x1卷积。GhostModule是一种轻量级的模块,可以减少计算量和参数数量。它通过在输入特征图上进行随机采样,生成多个子特征图,然后将它们进行拼接和卷积操作来实现类似于1x1卷积的效果。
下面是使用GhostModule替代1x1卷积的代码示例:
```python
import torch
import torch.nn as nn
class GhostModule(nn.Module):
def __init__(self, in_channels, out_channels, kernel_size=1, ratio=2):
super(GhostModule, self).__init__()
self.primary_conv = nn.Conv2d(in_channels, out_channels // ratio, kernel_size, stride=1, padding=0, bias=False)
self.cheap_operation = nn.Conv2d(out_channels // ratio, out_channels, 1, stride=1, padding=0, bias=False)
def forward(self, x):
x1 = self.primary_conv(x)
x2 = self.cheap_operation(x1)
out = torch.cat([x1, x2], dim=1)
return out
# 使用GhostModule替代1x1卷积
branch1x1 = GhostModule(in_channels, 32, kernel_size=1)
```
请注意,上述代码中的`in_channels`是输入特征图的通道数,可以根据实际情况进行调整。
相关推荐
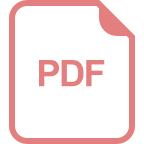












