pytorch如何实现feature transpose
时间: 2023-09-11 07:05:07 浏览: 49
在PyTorch中,可以使用`torch.transpose()`函数来实现特征转置。该函数的语法如下:
```python
torch.transpose(input, dim0, dim1)
```
其中,`input`是要进行转置操作的张量,`dim0`和`dim1`是需要交换的维度的索引。
例如,对于一个形状为`(batch_size, channels, height, width)`的输入张量,如果我们想要将其特征维度(即`channels`维)与高度维进行交换,可以使用以下代码:
```python
import torch
x = torch.randn(2, 3, 4, 5) # shape: (batch_size, channels, height, width)
x_transposed = torch.transpose(x, 1, 2) # swap channels and height dimensions
print(x_transposed.shape) # output: torch.Size([2, 4, 3, 5])
```
这里,`dim0=1`表示要交换的第一个维度是特征维度,`dim1=2`表示要交换的第二个维度是高度维。函数的输出将是一个形状为`(batch_size, height, channels, width)`的张量。
相关问题
用pytorch实现Dense Feature Fusion模块
以下是使用 PyTorch 实现 Dense Feature Fusion 模块的示例代码:
```python
import torch
import torch.nn as nn
import torch.nn.functional as F
class DFF(nn.Module):
def __init__(self, in_channels, out_channels):
super(DFF, self).__init__()
self.conv1 = nn.Conv2d(in_channels, out_channels, kernel_size=1)
self.conv2 = nn.Conv2d(out_channels, out_channels, kernel_size=1)
self.conv3 = nn.Conv2d(out_channels, out_channels, kernel_size=1)
self.conv4 = nn.Conv2d(out_channels, out_channels, kernel_size=1)
self.softmax = nn.Softmax(dim=-1)
def forward(self, x):
# 对特征映射进行特征对齐操作
B, C, H, W = x.shape
x = x.view(B, C, -1)
x = x - x.mean(dim=-1, keepdim=True)
cov = torch.bmm(x, x.transpose(1, 2)) / (H * W - 1)
u, s, v = torch.svd(cov)
x = torch.bmm(torch.bmm(u, self.softmax(s).unsqueeze(-1) * v.transpose(1, 2)), x).view(B, C, H, W)
# 动态滤波操作
x1 = F.relu(self.conv1(x))
x2 = F.relu(self.conv2(x1))
x3 = F.relu(self.conv3(x2))
x4 = self.conv4(x3)
x = x1 + x2 + x3 + x4
return x
```
在上述代码中,我们定义了一个名为 DFF 的 PyTorch 模块,该模块包含了两个主要的操作:特征映射对齐和动态滤波。在特征映射对齐操作中,我们使用了 SVD 分解来实现特征映射的对齐,具体来说,我们先将特征映射展开为一个二维矩阵,然后对该矩阵进行中心化和协方差矩阵的计算,最后使用 SVD 分解将特征映射对齐到一个相同的几何形状。在动态滤波操作中,我们使用了四个卷积层来实现动态滤波,并使用了 relu 激活函数来增强模型的非线性拟合能力。最后,我们将四个卷积层的输出相加得到最终的输出特征映射。
需要注意的是,上述代码中的实现仅是 DFF 模块的一种实现方式,实际上 DFF 模块的具体实现方式可能会因任务和数据集的不同而有所变化。因此,在实际使用时需要根据具体情况进行调整和优化。
pytorch实现PointNet深度学习网络
可以使用以下代码实现PointNet深度学习网络:
```
import torch
import torch.nn as nn
import torch.nn.functional as F
class TNet(nn.Module):
def __init__(self, k=3):
super(TNet, self).__init__()
self.k = k
self.conv1 = nn.Conv1d(k, 64, 1)
self.conv2 = nn.Conv1d(64, 128, 1)
self.conv3 = nn.Conv1d(128, 1024, 1)
self.fc1 = nn.Linear(1024, 512)
self.fc2 = nn.Linear(512, 256)
self.fc3 = nn.Linear(256, k*k)
self.bn1 = nn.BatchNorm1d(64)
self.bn2 = nn.BatchNorm1d(128)
self.bn3 = nn.BatchNorm1d(1024)
self.bn4 = nn.BatchNorm1d(512)
self.bn5 = nn.BatchNorm1d(256)
self.transform = nn.Parameter(torch.eye(k).unsqueeze(0))
def forward(self, x):
batchsize = x.size()[0]
x = F.relu(self.bn1(self.conv1(x)))
x = F.relu(self.bn2(self.conv2(x)))
x = F.relu(self.bn3(self.conv3(x)))
x = torch.max(x, 2, keepdim=True)[0]
x = x.view(-1, 1024)
x = F.relu(self.bn4(self.fc1(x)))
x = F.relu(self.bn5(self.fc2(x)))
x = self.fc3(x)
iden = torch.eye(self.k).view(1, self.k*self.k).repeat(batchsize, 1)
if x.is_cuda:
iden = iden.cuda()
x = x + iden
x = x.view(-1, self.k, self.k)
return x
class STN3d(nn.Module):
def __init__(self, k=3):
super(STN3d, self).__init__()
self.k = k
self.conv1 = nn.Conv1d(k, 64, 1)
self.conv2 = nn.Conv1d(64, 128, 1)
self.conv3 = nn.Conv1d(128, 1024, 1)
self.fc1 = nn.Linear(1024, 512)
self.fc2 = nn.Linear(512, 256)
self.fc3 = nn.Linear(256, k*k)
self.bn1 = nn.BatchNorm1d(64)
self.bn2 = nn.BatchNorm1d(128)
self.bn3 = nn.BatchNorm1d(1024)
self.bn4 = nn.BatchNorm1d(512)
self.bn5 = nn.BatchNorm1d(256)
self.transform = nn.Parameter(torch.zeros(batchsize, self.k, self.k))
nn.init.constant_(self.transform, 0)
def forward(self, x):
batchsize = x.size()[0]
x = F.relu(self.bn1(self.conv1(x)))
x = F.relu(self.bn2(self.conv2(x)))
x = F.relu(self.bn3(self.conv3(x)))
x = torch.max(x, 2, keepdim=True)[0]
x = x.view(-1, 1024)
x = F.relu(self.bn4(self.fc1(x)))
x = F.relu(self.bn5(self.fc2(x)))
x = self.fc3(x)
iden = torch.eye(self.k).view(1, self.k*self.k).repeat(batchsize, 1)
if x.is_cuda:
iden = iden.cuda()
x = x + iden
x = x.view(-1, self.k, self.k)
return x
class PointNetEncoder(nn.Module):
def __init__(self, global_feat=True, feature_transform=False):
super(PointNetEncoder, self).__init__()
self.stn = STN3d()
self.conv1 = nn.Conv1d(3, 64, 1)
self.conv2 = nn.Conv1d(64, 128, 1)
self.conv3 = nn.Conv1d(128, 1024, 1)
self.bn1 = nn.BatchNorm1d(64)
self.bn2 = nn.BatchNorm1d(128)
self.bn3 = nn.BatchNorm1d(1024)
self.global_feat = global_feat
self.feature_transform = feature_transform
if self.feature_transform:
self.fstn = TNet(k=64)
def forward(self, x):
n_pts = x.size()[2]
trans = self.stn(x)
x = x.transpose(2, 1)
x = torch.bmm(x, trans)
x = x.transpose(2, 1)
x = F.relu(self.bn1(self.conv1(x)))
if self.feature_transform:
trans_feat = self.fstn(x)
x = x.transpose(2,1)
x = torch.bmm(x, trans_feat)
x = x.transpose(2,1)
else:
trans_feat = None
x = F.relu(self.bn2(self.conv2(x)))
x = self.bn3(self.conv3(x))
x = torch.max(x, 2, keepdim=True)[0]
x = x.view(-1, 1024)
if self.global_feat:
return x, trans, trans_feat
else:
x = x.view(-1, 1024, 1).repeat(1, 1, n_pts)
return torch.cat([x, trans], 1), trans_feat
class PointNetDecoder(nn.Module):
def __init__(self, feature_transform=False):
super(PointNetDecoder, self).__init__()
self.feature_transform = feature_transform
if self.feature_transform:
self.fstn = TNet(k=128)
self.conv1 = nn.Conv1d(1088, 512, 1)
self.conv2 = nn.Conv1d(512, 256, 1)
self.conv3 = nn.Conv1d(256, 128, 1)
self.conv4 = nn.Conv1d(128, 3, 1)
self.bn1 = nn.BatchNorm1d(512)
self.bn2 = nn.BatchNorm1d(256)
self.bn3 = nn.BatchNorm1d(128)
def forward(self, x, trans, trans_feat):
n_pts = x.size()[2]
x = F.relu(self.bn1(self.conv1(x)))
if self.feature_transform:
x = x.transpose(2,1)
trans_feat = self.fstn(x)
x = x.transpose(2,1)
x = F.relu(self.bn2(self.conv2(x)))
x = F.relu(self.bn3(self.conv3(x)))
x = self.conv4(x)
x = x.transpose(2,1)
x = torch.bmm(x, trans)
x = x.transpose(2,1)
return x
class PointNet(nn.Module):
def __init__(self, feature_transform=False):
super(PointNet, self).__init__()
self.feature_transform = feature_transform
self.encoder = PointNetEncoder(global_feat=True, feature_transform=self.feature_transform)
self.decoder = PointNetDecoder(feature_transform=self.feature_transform)
def forward(self, x):
x, trans, trans_feat = self.encoder(x)
x = self.decoder(x, trans, trans_feat)
return x
```
这个代码实现了一个PointNet深度学习网络,可以用于点云分类、分割和重建等任务。
相关推荐
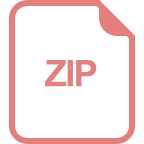
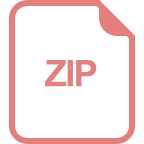













