代码解释:if not os.path.exists(txtpath): os.makedirs(txtpath, exist_ok=True)
时间: 2023-06-08 20:03:58 浏览: 189
这段代码的作用是先判断指定的文件路径是否存在,如果不存在则创建该路径,如果该路径已存在,则不进行任何操作。其中的os模块是Python的标准库之一,用于与操作系统进行交互。exists()函数用于判断指定路径是否存在,makedirs()函数则用于创建多层级的文件夹路径。exist_ok参数为True表示如果路径已经存在则不会抛出异常,否则会抛出OSError异常。
相关问题
将下面代码简洁化:def split_dataset(img_path, target_folder_path, output_path): filename = [] total_imgs = os.listdir(img_path) #for root, dirs, files in os.walk(img_path): for img in total_imgs: filename.append(img) np.random.shuffle(filename) train = filename[:int(len(filename) * 0.9)] test = filename[int(len(filename) * 0.9):] out_images = os.path.join(output_path, 'imgs') if not os.path.exists(out_images): os.makedirs(out_images) out_images_train = os.path.join(out_images, 'training') if not os.path.exists(out_images_train): os.makedirs(out_images_train) out_images_test = os.path.join(out_images, 'test') if not os.path.exists(out_images_test): os.makedirs(out_images_test) out_annotations = os.path.join(output_path, 'annotations') if not os.path.exists(out_annotations): os.makedirs(out_annotations) out_annotations_train = os.path.join(out_annotations, 'training') if not os.path.exists(out_annotations_train): os.makedirs(out_annotations_train) out_annotations_test = os.path.join(out_annotations, 'test') if not os.path.exists(out_annotations_test): os.makedirs(out_annotations_test) for i in train: print(os.path.join(img_path, i)) print(os.path.join(out_images_train, i)) shutil.copyfile(os.path.join(img_path, i), os.path.join(out_images_train, i)) annotations_name = "gt_" + i[:-3] + 'txt' shutil.copyfile(os.path.join(target_folder_path, annotations_name), os.path.join(out_annotations_train, annotations_name)) for i in test: shutil.copyfile(os.path.join(img_path, i), os.path.join(out_images_test, i)) annotations_name = "gt_" + i[:-3] + 'txt' shutil.copyfile(os.path.join(target_folder_path, annotations_name), os.path.join(out_annotations_test, annotations_name))
def split_dataset(img_path, target_folder_path, output_path):
filename = os.listdir(img_path)
np.random.shuffle(filename)
train = filename[:int(len(filename) * 0.9)]
test = filename[int(len(filename) * 0.9):]
out_images = os.path.join(output_path, 'imgs')
os.makedirs(out_images, exist_ok=True)
out_images_train = os.path.join(out_images, 'training')
os.makedirs(out_images_train, exist_ok=True)
out_images_test = os.path.join(out_images, 'test')
os.makedirs(out_images_test, exist_ok=True)
out_annotations = os.path.join(output_path, 'annotations')
os.makedirs(out_annotations, exist_ok=True)
out_annotations_train = os.path.join(out_annotations, 'training')
os.makedirs(out_annotations_train, exist_ok=True)
out_annotations_test = os.path.join(out_annotations, 'test')
os.makedirs(out_annotations_test, exist_ok=True)
for i in train:
img_src = os.path.join(img_path, i)
img_dst = os.path.join(out_images_train, i)
shutil.copyfile(img_src, img_dst)
annotations_name = "gt_" + i[:-3] + 'txt'
annotations_src = os.path.join(target_folder_path, annotations_name)
annotations_dst = os.path.join(out_annotations_train, annotations_name)
shutil.copyfile(annotations_src, annotations_dst)
for i in test:
img_src = os.path.join(img_path, i)
img_dst = os.path.join(out_images_test, i)
shutil.copyfile(img_src, img_dst)
annotations_name = "gt_" + i[:-3] + 'txt'
annotations_src = os.path.join(target_folder_path, annotations_name)
annotations_dst = os.path.join(out_annotations_test, annotations_name)
shutil.copyfile(annotations_src, annotations_dst)
代码解释:if __name__ == "__main__": postfix = 'jpg' imgpath = './VOCdevkit/JPEGImages' xmlpath = './VOCdevkit/Annotations' txtpath = './VOCdevkit/txt' if not os.path.exists(txtpath): os.makedirs(txtpath, exist_ok=True) list = os.listdir(xmlpath) error_file_list = [] for i in range(0, len(list)): try: path = os.path.join(xmlpath, list[i]) if ('.xml' in path) or ('.XML' in path): convert_annotation(path, list[i]) print(f'file {list[i]} convert success.') else: print(f'file {list[i]} is not xml format.') except Exception as e: print(f'file {list[i]} convert error.') print(f'error message:\n{e}') error_file_list.append(list[i]) print(f'this file convert failure\n{error_file_list}') print(f'Dataset Classes:{classes}')
这是一段 Python 代码,主要是将 VOC 数据集的 XML 标注文件转换为 txt 文件。如果没有 txt 文件夹,则会创建一个。程序将列出 XML 文件夹中的所有文件,遍历这些文件,并尝试将每个 XML 文件转换为对应的 txt 文件。如果遇到错误,程序将在控制台打印出错信息,并将错误文件名添加到 error_file_list 中输出。最后打印出转换失败的文件列表和数据集分类。
阅读全文
相关推荐
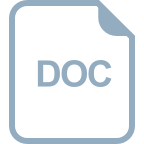
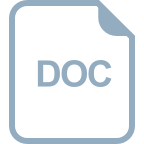
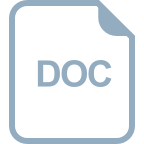














