figure path = path + repmat(S- [1 1] ,size(path,1),1); drawPath(path,G0,0)
时间: 2024-03-04 08:51:53 浏览: 47
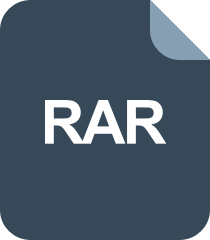
2AM_matlab_信号处理_matlab2fsk_closeqj1_
这部分代码中,首先在当前图形窗口中绘制从起点S到终点E,并经过点bestX的最优路径。接下来,将路径path中每个点的坐标都向左上角平移一个单位,再将其存储在变量path中。这个平移操作的目的是将路径从图像坐标系转换到网格坐标系。最后,使用函数drawPath()将路径path在网格G0上绘制出来,并显示在图像窗口中。
阅读全文
相关推荐
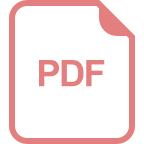
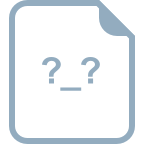
















