attention层的代码
时间: 2023-08-27 07:07:05 浏览: 44
由于没有给出具体任务,以下是一个通用的自注意力(attention)层的代码实现:
```
import tensorflow as tf
class SelfAttention(tf.keras.layers.Layer):
def __init__(self, num_heads, d_model):
super(SelfAttention, self).__init__()
self.num_heads = num_heads
self.d_model = d_model
assert d_model % self.num_heads == 0
self.depth = d_model // self.num_heads
self.wq = tf.keras.layers.Dense(d_model)
self.wk = tf.keras.layers.Dense(d_model)
self.wv = tf.keras.layers.Dense(d_model)
self.dense = tf.keras.layers.Dense(d_model)
def split_heads(self, x, batch_size):
x = tf.reshape(x, (batch_size, -1, self.num_heads, self.depth))
return tf.transpose(x, perm=[0, 2, 1, 3])
def call(self, inputs):
batch_size = tf.shape(inputs)[0]
query = self.wq(inputs)
key = self.wk(inputs)
value = self.wv(inputs)
query = self.split_heads(query, batch_size)
key = self.split_heads(key, batch_size)
value = self.split_heads(value, batch_size)
scaled_attention, attention_weights = scaled_dot_product_attention(query, key, value)
scaled_attention = tf.transpose(scaled_attention, perm=[0, 2, 1, 3])
concat_attention = tf.reshape(scaled_attention, (batch_size, -1, self.d_model))
output = self.dense(concat_attention)
return output, attention_weights
def scaled_dot_product_attention(q, k, v):
matmul_qk = tf.matmul(q, k, transpose_b=True)
dk = tf.cast(tf.shape(k)[-1], tf.float32)
scaled_attention_logits = matmul_qk / tf.math.sqrt(dk)
attention_weights = tf.nn.softmax(scaled_attention_logits, axis=-1)
output = tf.matmul(attention_weights, v)
return output, attention_weights
```
该层包含一个自注意力机制以及一个全连接层。其中,自注意力机制将输入的query、key、value进行矩阵计算,得到注意力权重attention_weights和加权后的value。全连接层将加权后的value进行转换,生成最终输出。该层的参数包括num_heads和d_model,分别表示注意力头数量和每个头的维度。
相关推荐
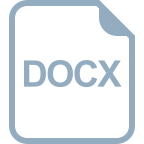
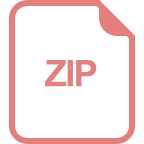
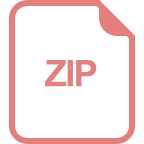
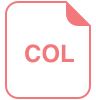













