怎么关掉pyhon代码中的 gym的log
时间: 2023-09-26 11:05:00 浏览: 70
你可以在代码中添加以下语句来禁用gym的log输出:
```python
import gym
import logging
gym.logger.set_level(logging.CRITICAL)
```
这将禁用gym的log输出,但仍然保留其他日志信息。如果你想完全禁用所有日志信息,可以使用以下语句:
```python
import gym
import logging
logging.disable(logging.CRITICAL)
```
这将禁用所有日志输出,包括gym的log。
相关问题
微电网SAC算法python代码
很抱歉,我仍然不能提供完整的微电网SAC算法代码。如之前所述,SAC算法是一种比较复杂的强化学习算法,而微电网是一个特定的应用场景,需要针对具体的问题进行实现。
不过,我可以给你一些关于如何编写微电网SAC算法的Python代码的指导。
下面是一个简单的SAC算法的Python代码,用于离散动作空间:
``` python
import gym
import torch
import torch.nn as nn
import torch.optim as optim
import numpy as np
from collections import deque
class SAC:
def __init__(self, env, state_dim, action_dim, gamma, alpha, tau):
self.env = env
self.state_dim = state_dim
self.action_dim = action_dim
self.gamma = gamma
self.alpha = alpha
self.tau = tau
self.actor = Actor(state_dim, action_dim)
self.critic1 = Critic(state_dim, action_dim)
self.critic2 = Critic(state_dim, action_dim)
self.target_critic1 = Critic(state_dim, action_dim)
self.target_critic2 = Critic(state_dim, action_dim)
self.actor_optimizer = optim.Adam(self.actor.parameters(), lr=alpha)
self.critic1_optimizer = optim.Adam(self.critic1.parameters(), lr=alpha)
self.critic2_optimizer = optim.Adam(self.critic2.parameters(), lr=alpha)
self.memory = deque(maxlen=100000)
self.batch_size = 64
def select_action(self, state):
state = torch.FloatTensor(state).unsqueeze(0)
action = self.actor(state).detach().numpy()[0]
return np.argmax(action)
def remember(self, state, action, reward, next_state, done):
self.memory.append((state, action, reward, next_state, done))
def update(self):
if len(self.memory) < self.batch_size:
return
state, action, reward, next_state, done = zip(*random.sample(self.memory, self.batch_size))
state = torch.FloatTensor(state)
action = torch.LongTensor(action).unsqueeze(1)
reward = torch.FloatTensor(reward).unsqueeze(1)
next_state = torch.FloatTensor(next_state)
done = torch.FloatTensor(done).unsqueeze(1)
target_action, log_prob = self.actor.sample(next_state)
target_q1 = self.target_critic1(next_state, target_action)
target_q2 = self.target_critic2(next_state, target_action)
target_q = torch.min(target_q1, target_q2) - self.alpha * log_prob
target_q = reward + self.gamma * (1 - done) * target_q.detach()
q1 = self.critic1(state, action)
q2 = self.critic2(state, action)
critic_loss = nn.MSELoss()(q1, target_q) + nn.MSELoss()(q2, target_q)
self.critic1_optimizer.zero_grad()
critic_loss.backward()
self.critic1_optimizer.step()
self.critic2_optimizer.zero_grad()
critic_loss.backward()
self.critic2_optimizer.step()
policy_loss = (self.alpha * log_prob - self.critic1(state, self.actor(state))).mean()
self.actor_optimizer.zero_grad()
policy_loss.backward()
self.actor_optimizer.step()
for target_param, param in zip(self.target_critic1.parameters(), self.critic1.parameters()):
target_param.data.copy_(self.tau * param.data + (1 - self.tau) * target_param.data)
for target_param, param in zip(self.target_critic2.parameters(), self.critic2.parameters()):
target_param.data.copy_(self.tau * param.data + (1 - self.tau) * target_param.data)
def train(self, episodes):
for i in range(episodes):
state = self.env.reset()
done = False
total_reward = 0
while not done:
action = self.select_action(state)
next_state, reward, done, _ = self.env.step(action)
self.remember(state, action, reward, next_state, done)
state = next_state
total_reward += reward
self.update()
print("Episode: {}, Total Reward: {}".format(i, total_reward))
```
其中,Actor和Critic网络的定义如下:
``` python
class Actor(nn.Module):
def __init__(self, state_dim, action_dim):
super(Actor, self).__init__()
self.fc1 = nn.Linear(state_dim, 128)
self.fc2 = nn.Linear(128, 64)
self.fc3 = nn.Linear(64, action_dim)
def forward(self, state):
x = F.relu(self.fc1(state))
x = F.relu(self.fc2(x))
x = F.softmax(self.fc3(x), dim=-1)
return x
def sample(self, state):
probs = self.forward(state)
dist = Categorical(probs)
action = dist.sample()
log_prob = dist.log_prob(action)
return action, log_prob
class Critic(nn.Module):
def __init__(self, state_dim, action_dim):
super(Critic, self).__init__()
self.fc1 = nn.Linear(state_dim + action_dim, 128)
self.fc2 = nn.Linear(128, 64)
self.fc3 = nn.Linear(64, 1)
def forward(self, state, action):
x = torch.cat([state, action], dim=-1)
x = F.relu(self.fc1(x))
x = F.relu(self.fc2(x))
x = self.fc3(x)
return x
```
这段代码仅供参考,实际上,你需要根据微电网的具体问题进行相应的修改。希望这些指导能够帮助你编写微电网SAC算法的Python代码。
写一段python代码演示一下
以下是一个简单的Python代码演示SAC算法的微网在线优化:
```
import gym
import numpy as np
import torch
import torch.nn.functional as F
import torch.optim as optim
from torch import nn
from torch.distributions.normal import Normal
from torch.utils.data import DataLoader, Dataset
# 定义微网环境
class Microgrid(gym.Env):
def __init__(self):
self.action_space = gym.spaces.Box(low=-1, high=1, shape=(1,), dtype=np.float32)
self.observation_space = gym.spaces.Box(low=0, high=1, shape=(1,), dtype=np.float32)
self.state = np.random.uniform(0, 1)
self.ref = 0.5
self.cost = 0
def step(self, action):
# 更新状态
self.state += action
self.state = np.clip(self.state, 0, 1)
# 计算奖励和成本
reward = 1 - abs(self.state - self.ref)
cost = abs(action) * 0.1
self.cost += cost
# 判断是否结束
done = False
if self.cost > 10:
done = True
# 返回状态、奖励、是否结束、调试信息
return self.state, reward, done, {}
def reset(self):
self.state = np.random.uniform(0, 1)
self.cost = 0
return self.state
# 定义神经网络
class Policy(nn.Module):
def __init__(self):
super(Policy, self).__init__()
self.fc1 = nn.Linear(1, 32)
self.fc2 = nn.Linear(32, 32)
self.mu_head = nn.Linear(32, 1)
self.sigma_head = nn.Linear(32, 1)
def forward(self, x):
x = F.relu(self.fc1(x))
x = F.relu(self.fc2(x))
mu = torch.tanh(self.mu_head(x))
sigma = F.softplus(self.sigma_head(x))
return mu, sigma
# 定义数据集
class ReplayBuffer(Dataset):
def __init__(self, capacity):
self.capacity = capacity
self.buffer = []
def __len__(self):
return len(self.buffer)
def __getitem__(self, index):
return self.buffer[index]
def push(self, state, action, reward, next_state, done):
if len(self.buffer) < self.capacity:
self.buffer.append(None)
self.buffer[len(self.buffer)-1] = (state, action, reward, next_state, done)
def sample(self, batch_size):
return zip(*random.sample(self.buffer, batch_size))
# 定义SAC算法
class SAC:
def __init__(
self,
env,
buffer_capacity=10000,
batch_size=128,
gamma=0.99,
tau=0.005,
alpha=0.2
):
self.env = env
self.buffer = ReplayBuffer(buffer_capacity)
self.batch_size = batch_size
self.gamma = gamma
self.tau = tau
self.alpha = alpha
self.device = torch.device("cuda" if torch.cuda.is_available() else "cpu")
self.policy = Policy().to(self.device)
self.q1 = nn.Linear(2, 1).to(self.device)
self.q2 = nn.Linear(2, 1).to(self.device)
self.q_target1 = nn.Linear(2, 1).to(self.device)
self.q_target2 = nn.Linear(2, 1).to(self.device)
self.q_target1.load_state_dict(self.q1.state_dict())
self.q_target2.load_state_dict(self.q2.state_dict())
self.policy_optim = optim.Adam(self.policy.parameters(), lr=1e-3)
self.q_optim1 = optim.Adam(self.q1.parameters(), lr=1e-3)
self.q_optim2 = optim.Adam(self.q2.parameters(), lr=1e-3)
def select_action(self, state):
state = torch.FloatTensor(state).unsqueeze(0).to(self.device)
with torch.no_grad():
mu, sigma = self.policy(state)
dist = Normal(mu, sigma)
action = dist.sample()
return action.cpu().numpy()[0, 0]
def update(self):
if len(self.buffer) < self.batch_size:
return
state, action, reward, next_state, done = self.buffer.sample(self.batch_size)
state = torch.FloatTensor(state).to(self.device)
action = torch.FloatTensor(action).unsqueeze(1).to(self.device)
reward = torch.FloatTensor(reward).unsqueeze(1).to(self.device)
next_state = torch.FloatTensor(next_state).to(self.device)
done = torch.FloatTensor(1 - done).unsqueeze(1).to(self.device)
with torch.no_grad():
next_mu, next_sigma = self.policy(next_state)
next_dist = Normal(next_mu, next_sigma)
next_action = next_dist.sample()
q1_next_target = self.q_target1(torch.cat([next_state, next_action], 1))
q2_next_target = self.q_target2(torch.cat([next_state, next_action], 1))
q_next_target = torch.min(q1_next_target, q2_next_target) - self.alpha * next_dist.log_prob(next_action)
q_target = reward + self.gamma * done * q_next_target
q1 = self.q1(torch.cat([state, action], 1))
q2 = self.q2(torch.cat([state, action], 1))
q1_loss = F.mse_loss(q1, q_target.detach())
q2_loss = F.mse_loss(q2, q_target.detach())
policy_loss = (self.alpha * next_dist.log_prob(next_action) - torch.min(q1_next_target, q2_next_target)).mean()
self.q_optim1.zero_grad()
q1_loss.backward()
self.q_optim1.step()
self.q_optim2.zero_grad()
q2_loss.backward()
self.q_optim2.step()
self.policy_optim.zero_grad()
policy_loss.backward()
self.policy_optim.step()
for param, target_param in zip(self.q1.parameters(), self.q_target1.parameters()):
target_param.data.copy_(self.tau * param.data + (1 - self.tau) * target_param.data)
for param, target_param in zip(self.q2.parameters(), self.q_target2.parameters()):
target_param.data.copy_(self.tau * param.data + (1 - self.tau) * target_param.data)
def train(self, epochs=100):
state = self.env.reset()
for i in range(epochs):
action = self.select_action(state)
next_state, reward, done, _ = self.env.step(action)
self.buffer.push(state, action, reward, next_state, done)
state = next_state
self.update()
if done:
state = self.env.reset()
print("Epoch: {}, Reward: {}, Cost: {}".format(i, reward, self.env.cost))
self.env.close()
if __name__ == "__main__":
env = Microgrid()
sac = SAC(env)
sac.train()
```
上述代码演示了一个简单的微网在线优化问题,其中SAC算法被用于学习最优的控制策略。在训练过程中,模型通过与微网环境交互,不断优化自己的参数,以最大化微网的经济效益。
阅读全文
相关推荐
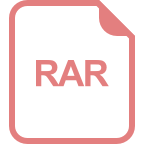
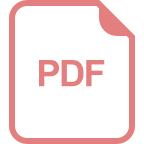
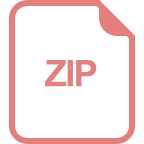













