self.layer1 = nn.Sequential( nn.Conv1d(1, 4, kernel_size=3, padding=1), nn.BatchNorm1d(4), nn.ReLU()) #nn.MaxPool2d(2))
时间: 2023-12-22 12:04:05 浏览: 41
这行代码定义了一个名为`layer1`的神经网络层。它是一个由三个子层组成的序列(`nn.Sequential`),分别是一个1维卷积层(`nn.Conv1d`)、一个批量归一化层(`nn.BatchNorm1d`)和一个ReLU激活函数层(`nn.ReLU`)。其中,`nn.Conv1d`的输入通道数为1,输出通道数为4,卷积核大小为3,填充为1。`nn.BatchNorm1d`是一种对卷积层输出进行标准化的方法,有助于提高模型的收敛速度和稳定性。`nn.ReLU`是一个非线性激活函数,可以引入非线性特征,提高模型的表达能力。这个`layer1`的输出大小将会是`(batch_size, 4, seq_len)`,其中`seq_len`是输入序列的长度。最后一行的`#nn.MaxPool2d(2)`是一行注释,表示这里还可以加上一个2维最大池化层,但是这行代码被注释掉了,因此不会执行。
相关问题
self.layer1 = nn.Sequential( nn.Conv1d(1, 4, kernel_size=3, padding=1), nn.BatchNorm1d(4), nn.ReLU()) self.layer2 = nn.Sequential( nn.Conv1d(4, 8, kernel_size=3, padding=1), nn.BatchNorm1d(8), nn.ReLU()) self.layer3 = nn.Sequential( nn.Conv1d(8, 8, kernel_size=3, padding=1), nn.BatchNorm1d(8), nn.ReLU()) #nn.Dropout(p=dropout), #nn.MaxPool1d(2)) self.layer4 = nn.Sequential( nn.Conv1d(16, 32, kernel_size=3, padding=1), nn.BatchNorm1d(32), nn.ReLU(), nn.Dropout(p=dropout), nn.MaxPool1d(2)) self.conv_last = nn.Conv1d(8, 1, kernel_size=1, padding=0) self.fc = nn.Linear(10, 1) #self.gamma = torch.nn.Parameter(torch.zeros(1))
这些代码定义了一个名为`CNN`的神经网络类,这个类继承自PyTorch的`nn.Module`类。这个类包含了四个卷积层和一个全连接层。
- `layer1`是一个由三个子层组成的序列,它的输入大小为`(batch_size, 1, seq_len)`,输出大小为`(batch_size, 4, seq_len)`。
- `layer2`是一个由三个子层组成的序列,它的输入大小为`(batch_size, 4, seq_len)`,输出大小为`(batch_size, 8, seq_len)`。
- `layer3`是一个由三个子层组成的序列,它的输入大小为`(batch_size, 8, seq_len)`,输出大小为`(batch_size, 8, seq_len)`。
- `layer4`是一个由四个子层组成的序列,它的输入大小为`(batch_size, 16, seq_len)`,输出大小为`(batch_size, 32, seq_len/2)`。
这些卷积层的输出都是1维张量。其中,`nn.Conv1d`表示1维卷积层,`nn.BatchNorm1d`表示1维批量归一化层,`nn.ReLU`表示ReLU激活函数层,`nn.Dropout`表示随机失活层,`nn.MaxPool1d`表示1维最大池化层。这些层的作用分别是提取特征、标准化特征、引入非线性、随机失活以防止过拟合和下采样。
接下来,`conv_last`定义了一个1维卷积层,它的输入大小为`(batch_size, 8, seq_len/2)`,输出大小为`(batch_size, 1, seq_len/2)`。这个层用于将卷积层的输出转化为一个单一的值。
最后,`fc`定义了一个全连接层,它的输入大小为10,输出大小为1。`gamma`是一个可学习的参数,用于控制模型的输出。
class ResidualBlock(nn.Module): def init(self, in_channels, out_channels, dilation): super(ResidualBlock, self).init() self.conv = nn.Sequential( nn.Conv1d(in_channels, out_channels, kernel_size=3, padding=dilation, dilation=dilation), nn.BatchNorm1d(out_channels), nn.ReLU(), nn.Conv1d(out_channels, out_channels, kernel_size=3, padding=dilation, dilation=dilation), nn.BatchNorm1d(out_channels), nn.ReLU() ) self.attention = nn.Sequential( nn.Conv1d(out_channels, out_channels, kernel_size=1), nn.Sigmoid() ) self.downsample = nn.Conv1d(in_channels, out_channels, kernel_size=1) if in_channels != out_channels else None def forward(self, x): residual = x out = self.conv(x) attention = self.attention(out) out = out * attention if self.downsample: residual = self.downsample(residual) out += residual return out class VMD_TCN(nn.Module): def init(self, input_size, output_size, n_k=1, num_channels=16, dropout=0.2): super(VMD_TCN, self).init() self.input_size = input_size self.nk = n_k if isinstance(num_channels, int): num_channels = [num_channels*(2**i) for i in range(4)] self.layers = nn.ModuleList() self.layers.append(nn.utils.weight_norm(nn.Conv1d(input_size, num_channels[0], kernel_size=1))) for i in range(len(num_channels)): dilation_size = 2 ** i in_channels = num_channels[i-1] if i > 0 else num_channels[0] out_channels = num_channels[i] self.layers.append(ResidualBlock(in_channels, out_channels, dilation_size)) self.pool = nn.AdaptiveMaxPool1d(1) self.fc = nn.Linear(num_channels[-1], output_size) self.w = nn.Sequential(nn.Conv1d(num_channels[-1], num_channels[-1], kernel_size=1), nn.Sigmoid()) # 特征融合 门控系统 # self.fc1 = nn.Linear(output_size * (n_k + 1), output_size) # 全部融合 self.fc1 = nn.Linear(output_size * 2, output_size) # 只选择其中两个融合 self.dropout = nn.Dropout(dropout) # self.weight_fc = nn.Linear(num_channels[-1] * (n_k + 1), n_k + 1) # 置信度系数,对各个结果加权平均 软投票思路 def vmd(self, x): x_imfs = [] signal = np.array(x).flatten() # flatten()必须加上 否则最后一个batch报错size不匹配! u, u_hat, omega = VMD(signal, alpha=512, tau=0, K=self.nk, DC=0, init=1, tol=1e-7) for i in range(u.shape[0]): imf = torch.tensor(u[i], dtype=torch.float32) imf = imf.reshape(-1, 1, self.input_size) x_imfs.append(imf) x_imfs.append(x) return x_imfs def forward(self, x): x_imfs = self.vmd(x) total_out = [] # for data in x_imfs: for data in [x_imfs[0], x_imfs[-1]]: out = data.transpose(1, 2) for layer in self.layers: out = layer(out) out = self.pool(out) # torch.Size([96, 56, 1]) w = self.w(out) out = w * out # torch.Size([96, 56, 1]) out = out.view(out.size(0), -1) out = self.dropout(out) out = self.fc(out) total_out.append(out) total_out = torch.cat(total_out, dim=1) # 考虑w1total_out[0]+ w2total_out[1],在第一维,权重相加得到最终结果,不用cat total_out = self.dropout(total_out) output = self.fc1(total_out) return output优化代码
1. 代码中的注释最好用英文,这样可以方便其他国家的程序员阅读和理解。
2. 在ResidualBlock类中,应该将init()改为__init__(),这是Python中的一个特殊方法,用于初始化类的实例变量。
3. 对于VMD_TCN类中的layers部分,可以使用一个for循环来代替多次重复的代码。例如:
```
for i in range(len(num_channels)):
dilation_size = 2 ** i
in_channels = num_channels[i-1] if i > 0 else num_channels[0]
out_channels = num_channels[i]
self.layers.append(ResidualBlock(in_channels, out_channels, dilation_size))
```
4. 不建议在forward()函数中使用numpy数组,应该使用PyTorch张量来保证代码的可重复性和GPU加速。例如,将self.vmd(x)中的signal变量改为torch.tensor(signal, dtype=torch.float32)。
5. 对于全连接层的输入尺寸,可以使用num_channels[-1] * self.nk代替output_size * (self.nk + 1),这样可以避免使用self.nk + 1这个魔数。
6. 在vmd()函数中,x_imfs可以使用PyTorch张量来存储,而不是使用Python列表。例如,可以使用torch.zeros((self.nk+1, self.input_size))来创建一个张量,并将每个u[i]复制到对应的张量中。这样可以避免在循环中多次创建张量,提高代码的效率。
7. 在forward()函数中,可以使用torch.cat()函数来将所有输出张量连接起来,而不是使用Python列表。例如,可以将total_out定义为一个空的张量,然后在每次迭代中使用torch.cat()函数将输出张量连接到total_out中。这样可以避免在循环中多次分配内存,提高代码的效率。
相关推荐
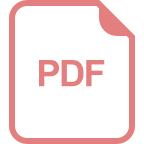
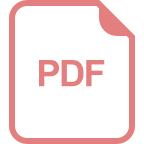













