解释最优:0.8204777025809096 使用{'n_estimators': 17} 0.580502 (0.199044) with {'n_estimators': 1} 0.681356 (0.194808) with {'n_estimators': 2} 0.746717 (0.098332) with {'n_estimators': 3} 0.729439 (0.148258) with {'n_estimators': 4} 0.795942 (0.074020) with {'n_estimators': 5} 0.792932 (0.074212) with {'n_estimators': 6} 0.790831 (0.081197) with {'n_estimators': 7} 0.806563 (0.069099) with {'n_estimators': 8} 0.804305 (0.089146) with {'n_estimators': 9} 0.796618 (0.099442) with {'n_estimators': 10} 0.796024 (0.084307) with {'n_estimators': 11} 0.810367 (0.092068) with {'n_estimators': 12} 0.813586 (0.082389) with {'n_estimators': 13} 0.801124 (0.096973) with {'n_estimators': 14} 0.816928 (0.086265) with {'n_estimators': 15} 0.806749 (0.087071) with {'n_estimators': 16} 0.820478 (0.090866) with {'n_estimators': 17} 0.814466 (0.081672) with {'n_estimators': 18} 0.799598 (0.105779) with {'n_estimators': 19} 0.814981 (0.087164) with {'n_estimators': 20} 0.816971 (0.085249) with {'n_estimators': 21}
时间: 2024-04-01 22:33:19 浏览: 227
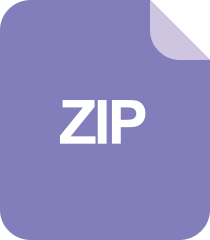
Python库 | region_estimators-0.1.28-py3-none-any.whl
这段文字是关于使用随机森林回归算法在数据集上进行参数调优的结果。其中,最优的表现为0.8204777025809096,相应的参数为{'n_estimators': 17},表示随机森林中树的数量为17。接下来,列出了不同树的数量时随机森林回归器的表现。可以看出,随着树的数量的增加,算法的表现会先上升再下降。因此,在这个数据集上,随机森林中17个决策树的表现最优。然而,需要注意的是,这个结果只是在这个特定数据集上得到的,对于其他数据集,最优的参数可能会有所不同。
阅读全文
相关推荐
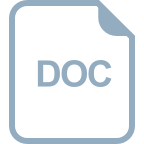
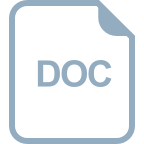
















