data.shape:2,64,96; self,norm(96)或self.norm(64) 是什么意思
时间: 2024-05-21 18:18:02 浏览: 24
`data.shape` 表示数据的维度,其中 2 表示 batch size,64 表示数据的通道数,96 表示数据的宽度。
`self.norm(96)` 或 `self.norm(64)` 是指对数据进行归一化处理的方法,其中的参数 96 或 64 表示归一化方法中需要用到的维度。一般来说,归一化方法会对每个维度上的数据进行独立的归一化处理,因此需要指定归一化处理需要用到的维度。在这里,使用 `self.norm` 对数据进行处理,其中的参数可以根据实际情况进行选择。
相关问题
for batch_idx, (data, target) in enumerate(self.train_loader): data = data[..., :self.args.input_dim] label = target[..., :self.args.output_dim] # (..., 1) self.optimizer.zero_grad() #teacher_forcing for RNN encoder-decoder model #if teacher_forcing_ratio = 1: use label as input in the decoder for all steps if self.args.teacher_forcing: global_step = (epoch - 1) * self.train_per_epoch + batch_idx teacher_forcing_ratio = self._compute_sampling_threshold(global_step, self.args.tf_decay_steps) else: teacher_forcing_ratio = 1. # data and target shape: B, T, N, F; output shape: B, T, N, F data, target维度均为64,12,307,1 output = self.model(data, target, teacher_forcing_ratio=teacher_forcing_ratio) if self.args.real_value: label = self.scaler.inverse_transform(label) loss = self.loss(output, label) loss.backward() # add max grad clipping if self.args.grad_norm: torch.nn.utils.clip_grad_norm_(self.model.parameters(), self.args.max_grad_norm) self.optimizer.step() total_loss += loss.item()
这段代码是一个训练循环的一部分,用于对批次数据进行训练。代码中使用`enumerate(self.train_loader)`来遍历训练数据集,并在每个批次中进行以下操作:
1. 首先,通过`data[..., :self.args.input_dim]`和`target[..., :self.args.output_dim]`对输入数据和标签进行切片,以获取指定维度的子集。这是为了确保输入和标签的维度匹配。
2. 然后,调用`self.optimizer.zero_grad()`来清零模型参数的梯度。
3. 接下来,根据`self.args.teacher_forcing`的值来确定是否使用"teacher forcing"的方法。如果`self.args.teacher_forcing`为真,则计算当前批次的全局步数,并使用`self._compute_sampling_threshold()`方法计算出"teacher forcing"的比例。否则,将"teacher forcing"比例设置为1.0,表示在解码器中的所有步骤都使用标签作为输入。
4. 调用`self.model(data, target, teacher_forcing_ratio=teacher_forcing_ratio)`来获取模型的输出。如果`self.args.real_value`为真,则通过`self.scaler.inverse_transform(label)`将标签逆转换为原始值。
5. 计算模型输出和标签之间的损失,并将损失值添加到总损失`total_loss`中。
6. 调用`loss.backward()`计算梯度,并使用`torch.nn.utils.clip_grad_norm_()`对梯度进行最大梯度裁剪。
7. 最后,调用`self.optimizer.step()`来更新模型参数。
这个循环会遍历整个训练数据集,并在每个批次中计算和更新模型的损失。
class LayerNorm(nn.Module): def __init__(self, normalized_shape, eps=1e-6, data_format="channels_last"): super(LayerNorm, self).__init__() self.weight = nn.Parameter(torch.ones(normalized_shape), requires_grad=True) self.bias = nn.Parameter(torch.zeros(normalized_shape), requires_grad=True) self.eps = eps self.data_format = data_format if self.data_format not in ['channels_last', 'channels_first']: raise ValueError(f"not support data format'{self.data_format}'") self.normalized_shape = (normalized_shape,) def forward(self, x: torch.Tensor) -> torch.Tensor: if self.data_format == 'channels_last': return F.layer_norm(x, self.normalized_shape, self.weight, self.bias, self.eps) elif self.data_format == 'channels_first': # [B,C,H,W] mean = x.mean(1, keepdim=True) var = (x - mean).pow(2).mean(1, keepdim=True) x = (x - mean) / torch.sqrt(var + self.eps) x = self.weight[:, None, None] * x + self.bias[:, None, None] return x
这段代码实现了Layer Normalization(层归一化)的功能。Layer Normalization是一种用于神经网络的归一化方法,可以在训练过程中稳定神经网络的学习。
在代码中,LayerNorm类继承自nn.Module,并实现了初始化方法和前向传播方法。
在初始化方法中,normalized_shape参数指定了归一化的维度大小,eps参数用于防止除零错误,data_format参数用于指定输入数据的格式('channels_last'或'channels_first')。
前向传播方法中,根据输入数据的格式进行不同的处理。当data_format为'channels_last'时,调用了PyTorch中的F.layer_norm函数进行归一化操作。当data_format为'channels_first'时,首先计算输入数据的均值和方差,然后进行归一化操作,并使用参数weight和bias进行缩放和偏移。
最后返回归一化后的结果。
阅读全文
相关推荐
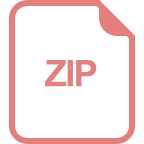
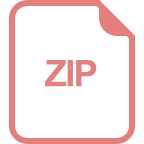
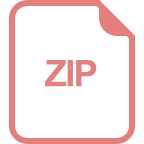
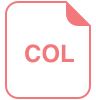
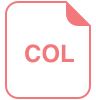
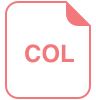
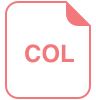









