Ceres的求解器都有哪些
时间: 2024-04-28 13:26:12 浏览: 89
Ceres提供了多种求解器,可以根据具体的问题和需求选择合适的求解器。主要的求解器包括:
1. 非线性最小二乘问题求解器
- Levenberg-Marquardt
- Dogleg
2. 非线性最小二乘问题的稀疏求解器
- Schur
- Schur + PCG
3. 非线性最小二乘问题的大规模稀疏求解器
- 非线性共轭梯度(Nonlinear Conjugate Gradient)
- L-BFGS(Limited-memory Broyden-Fletcher-Goldfarb-Shanno)
- Trust Region Reflective
4. 非线性最小二乘问题的混合求解器
- MinimizerType::TRUST_REGION
- MinimizerType::LINE_SEARCH
5. 非线性最小二乘问题的可扩展求解器
- GenericSolver
- DENSE_QR
- DENSE_SCHUR
- SPARSE_NORMAL_CHOLESKY
- SPARSE_SCHUR
- ITERATIVE_SCHUR
- CGNR
以上是Ceres主要的求解器,每个求解器都有其特点和适用范围。在使用时需要根据具体情况进行选择。
相关问题
用ceres solver求解二维变换矩阵
Ceres Solver是一个用于非线性最小二乘问题的开源C++库,它可以用于求解各种优化问题,包括二维变换矩阵的求解。
以下是一个使用Ceres Solver求解二维变换矩阵的示例代码:
```c++
#include <ceres/ceres.h>
#include <ceres/rotation.h>
#include <iostream>
// 定义二维点的结构体
struct Point2D {
double x;
double y;
};
// 定义二维变换矩阵的参数结构体
struct TransformParams {
double angle; // 旋转角度
double scale; // 缩放因子
double tx; // 水平平移
double ty; // 垂直平移
};
// 定义Ceres Solver的Cost Function
struct TransformCostFunctor {
TransformCostFunctor(const Point2D& from, const Point2D& to) : from_(from), to_(to) {}
template<typename T>
bool operator()(const T* const params, T* residual) const {
T rotated_x = cos(params[0]) * from_.x - sin(params[0]) * from_.y;
T rotated_y = sin(params[0]) * from_.x + cos(params[0]) * from_.y;
T scaled_x = params[1] * rotated_x;
T scaled_y = params[1] * rotated_y;
residual[0] = scaled_x + params[2] - to_.x;
residual[1] = scaled_y + params[3] - to_.y;
return true;
}
private:
const Point2D from_;
const Point2D to_;
};
int main() {
// 定义源点和目标点
Point2D from = { 2.0, 3.0 };
Point2D to = { 4.0, 5.0 };
// 定义Ceres Solver的Problem和参数块
ceres::Problem problem;
double params[4] = { 0.0, 1.0, 0.0, 0.0 };
ceres::ParameterBlock* param_block = problem.AddParameterBlock(params, 4);
// 定义Cost Function并添加到Problem中
ceres::CostFunction* cost_function = new ceres::AutoDiffCostFunction<TransformCostFunctor, 2, 4>(new TransformCostFunctor(from, to));
problem.AddResidualBlock(cost_function, nullptr, param_block);
// 设置Solver的选项并求解
ceres::Solver::Options options;
options.minimizer_progress_to_stdout = true;
ceres::Solver::Summary summary;
ceres::Solve(options, &problem, &summary);
// 输出结果
std::cout << summary.FullReport() << std::endl;
std::cout << "Transform matrix: " << std::endl;
std::cout << params[1] * cos(params[0]) << " " << -params[1] * sin(params[0]) << " " << params[2] << std::endl;
std::cout << params[1] * sin(params[0]) << " " << params[1] * cos(params[0]) << " " << params[3] << std::endl;
std::cout << "Residual: " << summary.final_cost << std::endl;
return 0;
}
```
在上述代码中,我们首先定义了一个二维点的结构体`Point2D`和一个二维变换矩阵的参数结构体`TransformParams`。然后我们定义了一个Ceres Solver的Cost Function`TransformCostFunctor`,它的作用是计算从源点变换到目标点需要的变换矩阵,并计算该变换矩阵下源点和目标点之间的残差。在`TransformCostFunctor`的`operator()`函数中,我们首先根据旋转角度将源点旋转,然后根据缩放因子进行缩放,并根据平移量进行平移,最后计算残差。
在`main()`函数中,我们首先定义了源点和目标点,并初始化了参数块。然后我们定义了一个Cost Function并将其添加到Problem中。最后我们设置了Solver的选项并调用`ceres::Solve`函数求解问题。求解完成后,我们可以通过`ceres::Solver::Summary`结构体中的信息输出求解结果和残差。
需要注意的是,上述代码只是求解了一个二维变换矩阵的例子,实际应用中可能需要更复杂的Cost Function和更多的参数。Ceres Solver提供了丰富的工具和接口,可以用于各种非线性优化问题的求解。
用ceres-solver求解二维变换矩阵
Ceres Solver 是一个用于非线性最小二乘问题的开源C++库。要使用 Ceres Solver 求解二维变换矩阵,需要定义一个误差函数,并用 Ceres Solver 最小化这个误差函数。
假设我们有一组原始点 $(x_i, y_i)$ 和目标点 $(u_i, v_i)$,要求一个二维变换矩阵 $H$,使得原始点通过 $H$ 变换后的坐标 $(x'_i, y'_i)$ 尽可能接近目标点 $(u_i, v_i)$。可以定义一个误差函数为:
$$
E(H) = \sum_{i=1}^n \left\| \begin{bmatrix} u_i \\ v_i \\ 1 \end{bmatrix} - H \begin{bmatrix} x_i \\ y_i \\ 1 \end{bmatrix} \right\|^2
$$
其中 $\left\| \cdot \right\|$ 表示向量的欧几里得范数。接下来,使用 Ceres Solver 最小化这个误差函数。Ceres Solver 需要提供一个初始的变换矩阵 $H_0$,可以使用单位矩阵作为初始值。
下面是一个使用 Ceres Solver 求解二维变换矩阵的例子:
```c++
#include <ceres/ceres.h>
#include <ceres/rotation.h>
struct TransformCostFunctor
{
TransformCostFunctor(double u, double v, double x, double y)
: u(u), v(v), x(x), y(y) {}
template <typename T>
bool operator()(const T* const h, T* residual) const
{
T x_transformed = h[0] * T(x) + h[1] * T(y) + h[2];
T y_transformed = h[3] * T(x) + h[4] * T(y) + h[5];
T w_transformed = h[6] * T(x) + h[7] * T(y) + h[8];
// 将齐次坐标转换为非齐次坐标
x_transformed /= w_transformed;
y_transformed /= w_transformed;
// 计算残差
residual[0] = T(u) - x_transformed;
residual[1] = T(v) - y_transformed;
return true;
}
const double u, v, x, y;
};
int main()
{
std::vector<double> x = { 1, 2, 3, 4 };
std::vector<double> y = { 1, 3, 4, 2 };
std::vector<double> u = { 2, 4, 6, 8 };
std::vector<double> v = { 2, 6, 8, 4 };
double h[9] = { 1, 0, 0, 0, 1, 0, 0, 0, 1 }; // 初始变换矩阵
ceres::Problem problem;
for (int i = 0; i < x.size(); i++)
{
ceres::CostFunction* cost_function =
new ceres::AutoDiffCostFunction<TransformCostFunctor, 2, 9>(
new TransformCostFunctor(u[i], v[i], x[i], y[i]));
problem.AddResidualBlock(cost_function, nullptr, h);
}
ceres::Solver::Options options;
options.max_num_iterations = 1000;
options.linear_solver_type = ceres::DENSE_QR;
options.minimizer_progress_to_stdout = true;
ceres::Solver::Summary summary;
ceres::Solve(options, &problem, &summary);
std::cout << summary.FullReport() << std::endl;
std::cout << "Final transformation matrix: " << std::endl;
for (int i = 0; i < 3; i++)
{
for (int j = 0; j < 3; j++)
{
std::cout << h[i * 3 + j] << " ";
}
std::cout << std::endl;
}
return 0;
}
```
在上面的代码中,我们定义了一个 `TransformCostFunctor` 类,用来计算每个点的误差。这个类重载了 `()` 运算符,接受一个变换矩阵 $H$ 和一个原始点 $(x_i, y_i)$,并计算变换后的坐标 $(x'_i, y'_i)$。然后,将变换后的坐标 $(x'_i, y'_i)$ 和目标点 $(u_i, v_i)$ 的差作为残差返回。
在 `main()` 函数中,我们首先定义了原始点和目标点的坐标。然后,定义了一个初始变换矩阵 `h`。接下来,使用 Ceres Solver 将每个点的误差加入到问题中,并设置求解器的参数。最后调用 `ceres::Solve()` 函数求解变换矩阵,并输出求解结果。
注意,这个例子中使用的是自动微分(AutoDiff),因此需要在 `ceres::AutoDiffCostFunction` 中指定 `TransformCostFunctor` 类的模板参数。如果你想使用其他的求导方法,可以使用 `ceres::NumericDiffCostFunction` 或自己实现 `ceres::CostFunction` 类。
阅读全文
相关推荐
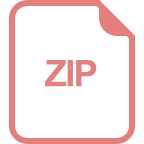
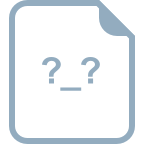
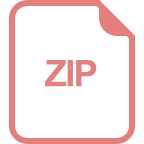
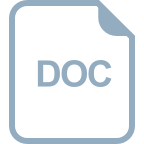
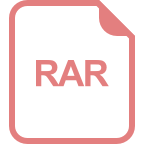
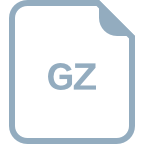
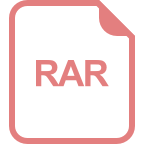










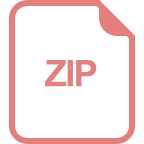