写一段CNN和LSTM并联的网络,其中CNN有3次二维卷积,输入数据的batch_size是4,in_channels=1,每个通道的图像尺寸为(22,751)
时间: 2024-03-01 10:55:44 浏览: 24
好的,下面是一段CNN和LSTM并联的网络代码,满足你的要求:
```
import torch
import torch.nn as nn
class CNN_LSTM(nn.Module):
def __init__(self):
super(CNN_LSTM, self).__init__()
# 定义CNN部分
self.cnn = nn.Sequential(
nn.Conv2d(in_channels=1, out_channels=16, kernel_size=(3, 3), padding=(1, 1)),
nn.ReLU(),
nn.Conv2d(in_channels=16, out_channels=32, kernel_size=(3, 3), padding=(1, 1)),
nn.ReLU(),
nn.Conv2d(in_channels=32, out_channels=64, kernel_size=(3, 3), padding=(1, 1)),
nn.ReLU()
)
# 定义LSTM部分
self.lstm = nn.LSTM(input_size=64 * 22, hidden_size=128, num_layers=2, batch_first=True)
def forward(self, x):
# x的shape为(batch_size, in_channels, 22, 751)
batch_size = x.shape[0]
x = self.cnn(x) # 经过CNN后的shape为(batch_size, 64, 22, 751)
x = x.view(batch_size, -1, 64 * 22) # reshape成(batch_size, 751, 64 * 22)
x, _ = self.lstm(x) # 经过LSTM后的shape为(batch_size, 751, 128)
return x
```
这段代码定义了一个名为`CNN_LSTM`的类,继承自`nn.Module`。其中,`cnn`部分定义了一个3层的CNN,每层都是由一个二维卷积层和一个ReLU激活函数构成。`lstm`部分定义了一个2层的LSTM,输入的特征维度为64*22,隐藏层大小为128。在前向传播过程中,先将输入数据经过CNN,将输出的张量reshape成(batch_size, 751, 64 * 22)的形状,再经过LSTM,最终输出(batch_size, 751, 128)的张量。
相关推荐
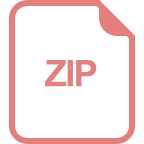
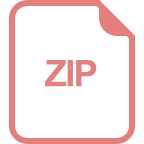
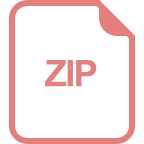














