pts_4d = torch.cat([points, points.new_ones(size=(num_points, 1))], dim=-1) pts_2d = pts_4d @ lidar2img_rt[view_idx].t() # pts_2d = pts_4d @ lidar2img_rt.t() pts_2d[:, 2] = torch.clamp(pts_2d[:, 2], min=1e-5) pts_2d[:, 0] /= pts_2d[:, 2] pts_2d[:, 1] /= pts_2d[:, 2]
时间: 2023-06-27 09:07:08 浏览: 150
这段代码是将点云数据从激光雷达坐标系转换到图像坐标系。首先,将点云数据的每个点的坐标添加一维1,变成4D张量。然后,将4D张量与激光雷达到图像的变换矩阵相乘,得到对应的2D点的坐标。这里注意到深度值可能为0或负数,因此使用 `clamp` 函数将深度值限制在一个较小的正数范围内。最后,将2D坐标的x、y坐标分别除以深度值,得到在图像上的真实位置。
相关问题
这段代码是啥意思:list_pts_blue = [[0, h / 2], [0, h / 2 + 20], [w, h / 2 + 20], [w, h / 2]] ndarray_pts_blue = np.array(list_pts_blue, np.int32) polygon_blue_value_1 = cv2.fillPoly(mask_image_temp, [ndarray_pts_blue], color=1) polygon_blue_value_1 = polygon_blue_value_1[:, :, np.newaxis] # 填充第二个撞线polygon(黄色) mask_image_temp = np.zeros((height, width), dtype=np.uint8) list_pts_yellow = [[0, h / 2 + 70], [0, h / 2 + 90], [w, h / 2 + 90], [w, h / 2 + 70]] ndarray_pts_yellow = np.array(list_pts_yellow, np.int32) polygon_yellow_value_2 = cv2.fillPoly(mask_image_temp, [ndarray_pts_yellow], color=2) polygon_yellow_value_2 = polygon_yellow_value_2[:, :, np.newaxis]
这段代码是使用 OpenCV 库对图像进行多边形填充的操作。首先,创建一个空的掩膜图像 `mask_image_temp`,大小为 `(height, width)`,数据类型为 `np.uint8`。然后,定义了两个多边形的顶点坐标列表 `list_pts_blue` 和 `list_pts_yellow`。
对于第一个多边形(蓝色),将顶点坐标列表转换为 NumPy 数组 `ndarray_pts_blue`,数据类型为 `np.int32`。然后使用 `cv2.fillPoly()` 函数将这个多边形填充到 `mask_image_temp` 中,颜色值为 1。最后,将填充后的结果 `polygon_blue_value_1` 添加一个额外的维度,变成三维数组。
对于第二个多边形(黄色),类似地,将顶点坐标列表转换为 NumPy 数组 `ndarray_pts_yellow`,数据类型为 `np.int32`。然后使用 `cv2.fillPoly()` 函数将这个多边形填充到另一个新的 `mask_image_temp` 中,颜色值为 2。同样,将填充后的结果 `polygon_yellow_value_2` 添加一个额外的维度,变成三维数组。
这段代码的作用是创建两个特定形状的多边形掩膜,并将其用不同的颜色值填充到相应的掩膜图像中。这样可以在后续的图像处理中使用这些掩膜来提取或操作特定区域的图像。
pts_2d_ori = contour_info["pts_2d"] pts_3d_ori = pm[pts_2d_ori[:, 0], pts_2d_ori[:, 1], :] pts_3d = pts_3d_ori[np.where(~np.isnan(pts_3d_ori[:, 0]))] pts_2d = pts_2d_ori[np.where(~np.isnan(pts_3d_ori[:, 0]))] pts_2d_ori = contour_info["pts_2d"] pts_3d_ori = pm[pts_2d_ori[:, 0], pts_2d_ori[:, 1], :] pts_3d = pts_3d_ori[np.where(~np.isnan(pts_3d_ori[:, 0]))] pts_2d = pts_2d_ori[np.where(~np.isnan(pts_3d_ori[:, 0]))]
这段代码的功能是将一个三维点云中的 NaN 值去除,并将点云的像素坐标和三维坐标分别保存到两个数组 pts_2d 和 pts_3d 中。
具体来说,首先从 contour_info 中取出轮廓的像素坐标 pts_2d_ori。然后通过 pm 数组和 pts_2d_ori 计算出每个像素点对应的三维坐标 pts_3d_ori。接着,使用 np.where 函数找到 pts_3d_ori 中不包含 NaN 值的索引位置,并将这些位置对应的三维坐标保存到 pts_3d 数组中。同时,将这些位置对应的像素坐标保存到 pts_2d 数组中。
最后,pts_2d 和 pts_3d 分别保存了点云的像素坐标和三维坐标,但是这些点云的数量可能会比原始的轮廓点数少,因为去除了 NaN 值。
阅读全文
相关推荐
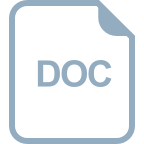
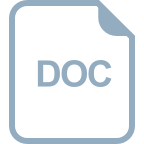
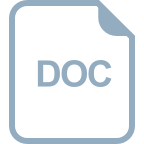













