为什么我的numpy没有gradient函数:AttributeError: 'numpy.ndarray' object has no attribute 'gradient'
时间: 2023-10-01 18:09:28 浏览: 112
`numpy.ndarray` 对象并没有 `gradient` 这个方法。如果你想要计算一个数组的梯度,你可以使用 `numpy.gradient()` 函数。这个函数接受一个数组作为参数,并返回一个元组,其中每个元素对应一个维度的梯度。
例如,如果你想计算一个二维数组的梯度,可以这样做:
```python
import numpy as np
arr = np.array([[1, 2, 3], [4, 5, 6], [7, 8, 9]])
grad_x, grad_y = np.gradient(arr)
```
这个例子中,`grad_x` 和 `grad_y` 分别是 `arr` 在 x 和 y 方向上的梯度。
相关问题
解决AttributeError: module 'tensorflow._api.v2.compat.v1.nn.rnn_cell' has no attribute 'seq2seq'
在 TensorFlow 2.0 中,`tf.nn.rnn_cell` 模块已经被移除了,因此 `tf.nn.rnn_cell.seq2seq` 也不再可用。相反,您可以使用 `tf.keras.layers` 中的相应函数来构建您的模型。下面是一个使用 `tf.keras` 实现 seq2seq 模型的示例:
``` python
import tensorflow as tf
# 定义编码器
class Encoder(tf.keras.Model):
def __init__(self, vocab_size, embedding_dim, enc_units, batch_sz):
super(Encoder, self).__init__()
self.batch_sz = batch_sz
self.enc_units = enc_units
self.embedding = tf.keras.layers.Embedding(vocab_size, embedding_dim)
self.gru = tf.keras.layers.GRU(self.enc_units, return_sequences=True, return_state=True, recurrent_initializer='glorot_uniform')
def call(self, x, hidden):
x = self.embedding(x)
output, state = self.gru(x, initial_state = hidden)
return output, state
def initialize_hidden_state(self):
return tf.zeros((self.batch_sz, self.enc_units))
# 定义注意力层
class BahdanauAttention(tf.keras.layers.Layer):
def __init__(self, units):
super(BahdanauAttention, self).__init__()
self.W1 = tf.keras.layers.Dense(units)
self.W2 = tf.keras.layers.Dense(units)
self.V = tf.keras.layers.Dense(1)
def call(self, query, values):
# query: 上一时间步的隐藏状态,shape=(batch_size, hidden_size)
# values: 编码器的输出,shape=(batch_size, max_length, hidden_size)
hidden_with_time_axis = tf.expand_dims(query, 1)
score = self.V(tf.nn.tanh(
self.W1(values) + self.W2(hidden_with_time_axis)))
# attention_weights shape == (batch_size, max_length, 1)
attention_weights = tf.nn.softmax(score, axis=1)
# context_vector shape after sum == (batch_size, hidden_size)
context_vector = attention_weights * values
context_vector = tf.reduce_sum(context_vector, axis=1)
return context_vector, attention_weights
# 定义解码器
class Decoder(tf.keras.Model):
def __init__(self, vocab_size, embedding_dim, dec_units, batch_sz):
super(Decoder, self).__init__()
self.batch_sz = batch_sz
self.dec_units = dec_units
self.embedding = tf.keras.layers.Embedding(vocab_size, embedding_dim)
self.gru = tf.keras.layers.GRU(self.dec_units, return_sequences=True, return_state=True, recurrent_initializer='glorot_uniform')
self.fc = tf.keras.layers.Dense(vocab_size)
# 用于注意力
self.attention = BahdanauAttention(self.dec_units)
def call(self, x, hidden, enc_output):
# enc_output shape == (batch_size, max_length, hidden_size)
context_vector, attention_weights = self.attention(hidden, enc_output)
# x shape after passing through embedding == (batch_size, 1, embedding_dim)
x = self.embedding(x)
# 将上一时间步的隐藏状态和注意力向量拼接起来作为输入传给 GRU
x = tf.concat([tf.expand_dims(context_vector, 1), x], axis=-1)
# 将拼接后的向量传给 GRU
output, state = self.gru(x)
# output shape == (batch_size * 1, hidden_size)
output = tf.reshape(output, (-1, output.shape[2]))
# output shape == (batch_size, vocab)
x = self.fc(output)
return x, state, attention_weights
# 定义损失函数和优化器
optimizer = tf.keras.optimizers.Adam()
loss_object = tf.keras.losses.SparseCategoricalCrossentropy(from_logits=True, reduction='none')
def loss_function(real, pred):
mask = tf.math.logical_not(tf.math.equal(real, 0))
loss_ = loss_object(real, pred)
mask = tf.cast(mask, dtype=loss_.dtype)
loss_ *= mask
return tf.reduce_mean(loss_)
# 定义训练步骤
@tf.function
def train_step(inp, targ, enc_hidden):
loss = 0
with tf.GradientTape() as tape:
enc_output, enc_hidden = encoder(inp, enc_hidden)
dec_hidden = enc_hidden
dec_input = tf.expand_dims([tokenizer.word_index['<start>']] * BATCH_SIZE, 1)
# teacher forcing - 将目标词作为下一个输入传给解码器
for t in range(1, targ.shape[1]):
# 将编码器的输出和上一时间步的隐藏状态传给解码器
predictions, dec_hidden, _ = decoder(dec_input, dec_hidden, enc_output)
loss += loss_function(targ[:, t], predictions)
# 使用 teacher forcing
dec_input = tf.expand_dims(targ[:, t], 1)
batch_loss = (loss / int(targ.shape[1]))
variables = encoder.trainable_variables + decoder.trainable_variables
gradients = tape.gradient(loss, variables)
optimizer.apply_gradients(zip(gradients, variables))
return batch_loss
# 定义预测函数
def evaluate(sentence):
attention_plot = np.zeros((max_length_targ, max_length_inp))
sentence = preprocess_sentence(sentence)
inputs = [tokenizer.word_index[i] for i in sentence.split(' ')]
inputs = tf.keras.preprocessing.sequence.pad_sequences([inputs], maxlen=max_length_inp, padding='post')
inputs = tf.convert_to_tensor(inputs)
result = ''
hidden = [tf.zeros((1, units))]
enc_out, enc_hidden = encoder(inputs, hidden)
dec_hidden = enc_hidden
dec_input = tf.expand_dims([tokenizer.word_index['<start>']], 0)
for t in range(max_length_targ):
predictions, dec_hidden, attention_weights = decoder(dec_input, dec_hidden, enc_out)
# 存储注意力权重以便后面制图
attention_weights = tf.reshape(attention_weights, (-1, ))
attention_plot[t] = attention_weights.numpy()
predicted_id = tf.argmax(predictions[0]).numpy()
result += tokenizer.index_word[predicted_id] + ' '
if tokenizer.index_word[predicted_id] == '<end>':
return result, sentence, attention_plot
# 将预测的 ID 作为下一个解码器输入的 ID
dec_input = tf.expand_dims([predicted_id], 0)
return result, sentence, attention_plot
```
在上面的代码中,我们使用了 `tf.keras.layers` 中的 `Embedding`、`GRU` 和 `Dense` 层来构建编码器和解码器,使用 `tf.keras.optimizers.Adam` 作为优化器,使用 `tf.keras.losses.SparseCategoricalCrossentropy` 作为损失函数。同时,我们还定义了一个 `BahdanauAttention` 层来实现注意力机制。
修改代码: File "D:\python\lib\site-packages\mpl_toolkits\mplot3d\axes3d.py", line 1569, in plot_surface if Z.ndim != 2: AttributeError: 'list' object has no attribute 'ndim'
这个错误通常发生在Z的维度不符合要求时,可以通过查看Z的类型和维度来确认问题。根据错误信息,Z被认为是一个list对象,而list对象没有ndim属性,因此导致了错误。
可以检查一下代码中生成Z的那一行,确认Z的类型和维度是否正确。也可以在生成Z之前,打印出相关的变量,检查是否符合预期。如果Z的类型不是numpy数组,可以使用np.array()将其转换为数组。
以下是修改后的代码:
```python
import numpy as np
import matplotlib.pyplot as plt
from mpl_toolkits.mplot3d import Axes3D
# 定义函数
def f(x, y):
return x**2 * np.exp(-x**2 + y)
# 定义 x, y 的取值范围和步长
x_min, x_max, y_min, y_max = -2, 2, -2, 2
step = 0.1
# 生成网格点坐标
x_values = np.arange(x_min, x_max, step)
y_values = np.arange(y_min, y_max, step)
X, Y = np.meshgrid(x_values, y_values)
# 计算曲面的曲率值
Z = np.gradient(np.gradient(f(X, Y)))
# 将Z转换为数组
Z = np.array(Z)
# 绘制曲面的曲率值图像
fig = plt.figure()
ax = fig.add_subplot(111, projection='3d')
ax.plot_surface(X, Y, Z, cmap='coolwarm', rstride=1, cstride=1, alpha=0.8)
ax.set_xlabel('x')
ax.set_ylabel('y')
ax.set_zlabel('curvature')
plt.show()
```
在生成Z之后,加入了将Z转换为数组的代码,确保Z的类型符合要求。这样就可以正常绘制曲率图像了。
阅读全文
相关推荐
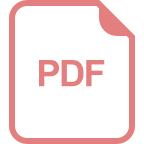
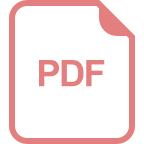
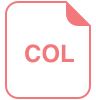
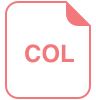
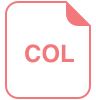
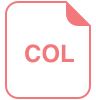
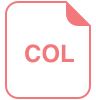
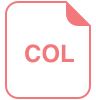

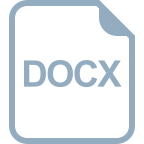
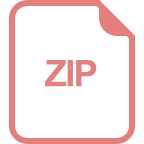