torch GNN实现 增加测试、训练代码
时间: 2023-12-09 11:03:39 浏览: 86
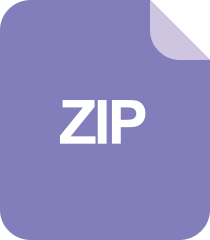
torch-GPU-PyG(torch-geometric)-相关工具包-2023.2.12
好的,我可以给你提供一个简单的 PyTorch GNN 实现,并且附带训练和测试代码。这个实现是基于 GCN (Graph Convolutional Network)的,你可以根据需要进行改进。
首先,我们需要安装 PyTorch 和 DGL(Deep Graph Library):
```bash
pip install torch dgl
```
然后,我们可以定义一个简单的 GNN 模型:
```python
import torch
import torch.nn as nn
import dgl.function as fn
from dgl.nn.pytorch import GraphConv
class GNN(nn.Module):
def __init__(self, in_feats, hidden_feats, out_feats):
super(GNN, self).__init__()
self.conv1 = GraphConv(in_feats, hidden_feats)
self.conv2 = GraphConv(hidden_feats, out_feats)
def forward(self, g, features):
h = self.conv1(g, features)
h = torch.relu(h)
h = self.conv2(g, h)
return h
```
这个 GNN 模型包含两个 GraphConv 层,每个层都是由输入特征到输出特征的线性变换,然后通过 ReLU 激活函数进行非线性变换。在这个例子中,我们使用了两个 GraphConv 层,但你可以根据需要添加更多层。
接下来,我们可以定义一个简单的训练循环:
```python
import dgl
import torch.optim as optim
def train(model, g, features, labels, train_mask, epochs):
optimizer = optim.Adam(model.parameters())
criterion = nn.CrossEntropyLoss()
for epoch in range(epochs):
model.train()
logits = model(g, features)
loss = criterion(logits[train_mask], labels[train_mask])
optimizer.zero_grad()
loss.backward()
optimizer.step()
print('Epoch %d | Loss: %.4f' % (epoch, loss.item()))
```
在这个训练循环中,我们使用 Adam 优化器和交叉熵损失函数对 GNN 进行训练。训练过程中,我们计算模型的预测值(logits),然后根据训练集上的标签和掩码计算交叉熵损失。最后,我们通过反向传播和优化器来更新模型参数。
最后,我们可以定义一个简单的测试函数:
```python
import torch.nn.functional as F
def test(model, g, features, labels, test_mask):
model.eval()
with torch.no_grad():
logits = model(g, features)
pred = logits.argmax(1)
acc = F.accuracy(logits[test_mask], labels[test_mask])
print('Accuracy: %.4f' % acc.item())
```
在这个测试函数中,我们首先将模型设置为评估模式,然后使用模型对测试集进行预测。最后,我们计算准确率并输出结果。
现在,我们可以使用这些代码来训练和测试我们的 GNN 模型:
```python
import dgl.data
dataset = dgl.data.CoraGraphDataset()
g = dataset[0]
features = g.ndata['feat']
labels = g.ndata['label']
train_mask = g.ndata['train_mask']
test_mask = g.ndata['test_mask']
model = GNN(features.shape[1], 16, dataset.num_classes)
train(model, g, features, labels, train_mask, epochs=100)
test(model, g, features, labels, test_mask)
```
在这个例子中,我们使用 Cora 数据集来测试我们的 GNN 模型。训练和测试代码将使用上述函数进行训练和测试,最后输出测试准确率。
阅读全文
相关推荐
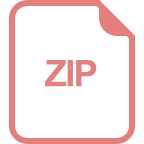
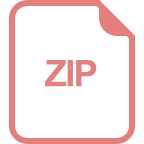
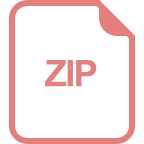
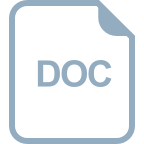
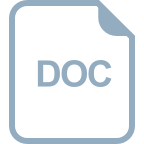
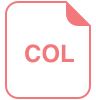
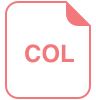










