pytorch求幂次方函数
时间: 2023-12-20 17:55:41 浏览: 33
PyTorch中可以使用torch.pow函数来求幂次方。它的用法如下:
```python
torch.pow(input, exponent, out=None) -> Tensor
```
其中,input是输入的tensor,exponent是幂次方的指数,out是可选的输出tensor。
举个例子,如果要计算2的3次方,可以这样写:
```python
import torch
x = torch.tensor(2.0)
y = torch.pow(x, 3)
print(y)
```
输出结果为:
```
tensor(8.)
```
如果要计算一个矩阵的幂次方,可以这样写:
```python
import torch
x = torch.tensor([[1, 2], [3, 4]], dtype=torch.float32)
y = torch.pow(x, 2)
print(y)
```
输出结果为:
```
tensor([[ 1., 4.],
[ 9., 16.]])
```
相关问题
python幂函数pytorch
在Pytorch中,可以使用torch.pow()函数来计算幂函数。该函数的作用是将输入张量的每个元素都进行幂运算,并返回一个新的张量。
使用方法如下:
torch.pow(input, exponent, out=None)
其中,input是待求幂的张量,exponent是幂指数。可以通过设置out参数来指定输出的张量。
以下是一个简单的示例,说明如何使用torch.pow()函数:
import torch
x = torch.tensor([2, 3, 4])
exponent = 2
result = torch.pow(x, exponent)
print(result)
输出结果为:
tensor([ 4, 9, 16])
pytorch中的阶跃函数
在PyTorch中,阶跃函数也称为单位阶跃函数(unit step function),是一种常见的激活函数。它的数学公式如下:
$$f(x) = \begin{cases} 0, & \text{if } x<0 \\ 1, & \text{if } x\geq 0 \end{cases}$$
可以看到,当输入$x$小于0时,输出为0;当输入$x$大于等于0时,输出为1。因此,阶跃函数可以将输入值转换为二进制信号,常用于二元分类问题中。
在PyTorch中,阶跃函数可以通过torch.nn.functional模块中的step函数实现。其使用方式如下:
```python
import torch.nn.functional as F
x = torch.randn(3)
out = F.step(x)
print(out)
```
上述代码中,首先导入torch.nn.functional模块中的step函数。然后,创建一个随机张量x,并使用step函数对其进行操作,最后输出结果。
相关推荐
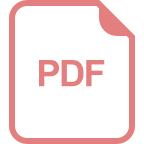
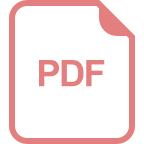












