if not train: # Load Pretrained Weights model_spot.load_weights(path) else: model_spot.set_weights(weight_reset_spot) history_spot = model_spot.fit( X_train, np.array(y_train), batch_size=batch_size, epochs=epochs_spot, verbose=0, validation_data = (X_test, np.array(y_test)), shuffle=True, callbacks=[keras.callbacks.ModelCheckpoint( filepath = path, save_weights_only=True )], )
时间: 2024-02-10 17:32:54 浏览: 142
从这段代码中可以看出,如果train为False,则会加载预训练的权重;如果train为True,则会使用重置后的权重进行训练。在训练时,使用了批处理大小为batch_size,训练轮数为epochs_spot,同时设置了验证集为(X_test, np.array(y_test)),并打乱数据顺序。此外,还使用了回调函数keras.callbacks.ModelCheckpoint,用于在每个epoch结束时保存模型权重。保存的路径为path。
相关问题
if train: model_spot_recog.save_weights(path) # Save Weights else: model_spot_recog.load_weights(path) # Load Pretrained Weights results = model_spot_recog.predict( X_test, verbose=1 报错AttributeError: 'NoneType' object has no attribute 'save_weights'
根据你提供的代码,错误是由于 `model_spot_recog` 是一个空对象,即 NoneType,而不是一个有效的模型对象。因此,它没有 `save_weights` 方法。
你需要检查 `model_spot_recog` 对象的初始化和赋值过程,确保它被正确地创建并赋值为一个具有 `save_weights` 方法的模型对象。
可能的原因包括:
1. 模型对象未正确初始化或创建。请确保在调用 `model_spot_recog.save_weights()` 之前,你已经正确地创建了一个模型对象,并将其赋值给了 `model_spot_recog`。
2. 在之前的代码中,可能存在错误导致 `model_spot_recog` 被重新赋值为 None。请检查之前的代码,确保没有对 `model_spot_recog` 进行了重新赋值。
如果你能提供更多关于模型初始化和赋值部分的代码,我可以提供更具体的帮助。
weight_reset_spot = model_spot.get_weights()
这段代码的作用是获取模型 `model_spot` 的所有权重,并将其存储在变量 `weight_reset_spot` 中。通常情况下,我们可以使用 `get_weights()` 方法获取模型的所有权重,然后使用 `set_weights()` 方法来设置新的权重。这在微调模型或恢复模型状态时非常有用。例如,在微调模型时,我们可能需要先保存模型的初始权重,并在训练过程中使用 `set_weights()` 方法来恢复初始状态。
阅读全文
相关推荐
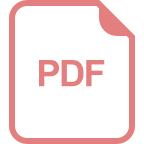
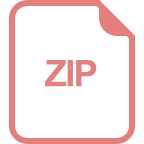













