def random_list(list): for i in range(list): c = random.randint(0, 100) print(c,end=' ') random_list(9)
时间: 2024-03-22 14:42:14 浏览: 171
这段代码存在一些问题,需要进行修改。首先,函数中的参数名`list`与Python内置的数据类型`list`重名,可能会导致混淆。建议修改为其他名称。
其次,函数中的循环只在生成随机数时起作用,而没有将随机数存储到列表中,导致函数无法返回生成的随机数列表。建议在循环中使用`append`方法将生成的随机数添加到列表中。
最后,调用函数时需要传入一个参数,表示要生成几个随机数。目前代码中的`random_list(9)`表示要生成9个随机数,但是函数中并没有使用该参数,因此需要将其修改为函数的参数。
综上所述,以下是修改后的代码:
```python
import random
def generate_random_list(num):
rand_list = []
for i in range(num):
c = random.randint(0, 100)
rand_list.append(c)
return rand_list
print(generate_random_list(9))
```
该代码会生成一个包含9个随机数的列表,并将其输出到控制台。
相关问题
解释每条语句作用import random def roll_dice(): #模拟掷骰子 roll = random.randint(1, 6) return roll def main(): #主函数 total_time = 10000 # 初始化列表 result_list = [0] * 11 # 初始化点数列表 roll_list = list(range(2, 13)) roll_dict = dict(zip(roll_list, result_list)) for i in range(total_time): roll1 = roll_dice() roll2 = roll_dice() # 获取点数存储到对应次数位置 for j in range(2, 13): if (roll1 + roll2) == j: roll_dict[j] += 1 break for i, result in roll_dict.items(): print('点数{}的次数{},频率:{}'.format(i, result, result / total_time)) if __name__ == '__main__': main()
这段代码实现了一个模拟掷骰子的程序,并统计了相应点数出现的次数和频率。具体解释如下:
1. `import random`:导入 Python 的随机数模块 `random`。
2. `def roll_dice():`:定义一个名为 `roll_dice` 的函数,用于模拟掷骰子。
3. `roll = random.randint(1, 6)`:用 `random` 模块中的 `randint()` 函数生成一个 1 到 6 之间的随机整数,模拟掷骰子的结果。
4. `return roll`:返回掷骰子的结果。
5. `def main():`:定义一个名为 `main` 的函数,作为程序的主函数。
6. `total_time = 10000`:初始化掷骰子的总次数为 10000。
7. `result_list = [0] * 11`:初始化一个长度为 11 的列表,用于存储不同点数出现的次数。
8. `roll_list = list(range(2, 13))`:初始化一个长度为 11 的列表,用于存储所有可能出现的点数。
9. `roll_dict = dict(zip(roll_list, result_list))`:创建一个字典,将点数列表和次数列表对应起来,用于后续的统计。
10. `for i in range(total_time):`:循环执行掷骰子的总次数次。
11. `roll1 = roll_dice()`:调用 `roll_dice()` 函数模拟掷骰子,获取第一个骰子的点数。
12. `roll2 = roll_dice()`:调用 `roll_dice()` 函数模拟掷骰子,获取第二个骰子的点数。
13. `for j in range(2, 13):`:循环遍历所有可能出现的点数。
14. `if (roll1 + roll2) == j:`:如果两个骰子的点数之和等于当前遍历的点数。
15. `roll_dict[j] += 1`:将当前点数出现的次数加 1。
16. `break`:跳出当前循环,继续下一次掷骰子。
17. `for i, result in roll_dict.items():`:循环遍历字典中的所有项。
18. `print('点数{}的次数{},频率:{}'.format(i, result, result / total_time))`:输出每种点数出现的次数和频率。
19. `if __name__ == '__main__':`:如果当前文件作为主程序运行。
20. `main()`:调用 `main()` 函数,执行程序的主体部分。
解析class UnionFind: def __init__(self, n): self.parent = list(range(n)) self.size = [1] * n def find(self, i): if self.parent[i] != i: self.parent[i] = self.find(self.parent[i]) return self.parent[i] def union(self, i, j): pi, pj = self.find(i), self.find(j) if pi != pj: if self.size[pi] < self.size[pj]: pi, pj = pj, pi self.parent[pj] = pi self.size[pi] += self.size[pj]# 示例import randomn = 10uf = UnionFind(n)print("初始集合:", [uf.find(i) for i in range(n)])for _ in range(5): i, j = random.randint(0, n-1), random.randint(0, n-1) uf.union(i, j) print("执行 union({}, {}) 后集合:".format(i, j), [uf.find(k) for k in range(n)])for _ in range(5): i, j = random.randint(0, n-1), random.randint(0, n-1) print("执行 find({}, {}) 的结果:{}, {}".format(i, j, uf.find(i), uf.find(j)))
这段代码实现了一个并查集(Union-Find)数据结构。具体解析如下:
- 首先定义了一个 UnionFind 类,其中包含三个方法:__init__()、find() 和 union()。
- __init__() 方法初始化了并查集,其中包含两个列表:parent 和 size。parent 用于存储每个元素所属的集合编号,初始时每个元素的编号都是自己本身,即 parent[i] = i。size 用于记录每个集合的大小,初始时每个集合只包含一个元素,即 size[i] = 1。
- find() 方法用于查找元素所属的集合编号。它首先通过递归方式找到元素所在集合的根节点,然后将所有经过的节点都直接连接到根节点上,以便下次查询时可以更快地找到根节点。最后返回根节点的编号即可。
- union() 方法用于将两个元素所在的集合合并成一个集合。它首先分别找到这两个元素所在的集合的根节点,然后将其中一个根节点的 parent 修改为另一个根节点的编号,以实现合并操作。同时还需要更新 size 列表,将较小的集合并入较大的集合中,以便后续查询时可以更快地找到根节点。
- 示例代码中创建了一个大小为 10 的并查集对象 uf,并进行了 5 次随机的 union 操作,输出每次操作后每个元素所在的集合编号。然后进行了 5 次随机的 find 操作,输出每次操作的结果。
总的来说,这段代码实现了一个经典的并查集数据结构,可以用于解决一些图论和优化算法中的问题。
阅读全文
相关推荐
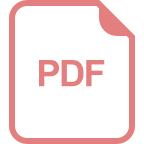















