import torch.nn as nn net = nn.Sequential(nn.Linear(2, 1))
时间: 2024-06-15 10:09:52 浏览: 138
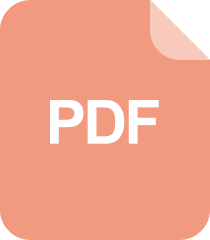
浅析PyTorch中nn.Linear的使用
`import torch.nn as nn` 是用于导入 PyTorch 库中的神经网络模块的命令。PyTorch 是一个开源的深度学习框架,用于构建和训练神经网络。
`nn.Sequential` 是 PyTorch 中的一种容器,用于将多个神经网络层按照顺序排列在一起。在这个例子中,`nn.Sequential(nn.Linear(2, 1))` 创建了一个包含一个线性层的序列。这意味着输入有 2 个特征,经过这个线性层后,输出为 1 个特征。
所以,这段代码的意思是创建了一个简单的神经网络模型,该模型有一个输入层(具有 2 个特征),经过一个线性层后,输出只有一个特征。
注意:在实际使用中,你可能需要添加更多的层(如激活函数、池化层等)来构建更复杂的网络结构。此外,你可能还需要定义损失函数和优化器来训练这个模型。
阅读全文
相关推荐
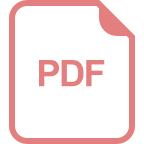
















