Four Types of Integral Transforms in Partial Differential Equations: Fourier, Laplace, and Hankel Transforms, Solved with Ease
发布时间: 2024-09-14 08:52:07 阅读量: 20 订阅数: 22 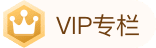
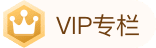
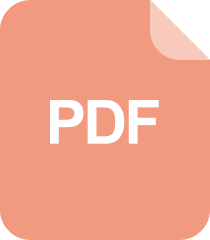
Forward-backward doubly stochastic differential equations with random jumps and stochastic partial differential-integral equations
# 1. Overview of Integral Transforms for Partial Differential Equations
Partial differential equations (PDEs) are widely used in physics, engineering, and mathematics to describe the rate of change of functions with multiple independent variables. Integral transforms are mathematical tools that can transform PDEs into simpler equations, thereby simplifying the solution process.
The basic idea of integral transf***mon integral transforms include Fourier, Laplace, and Hankel transforms, which are suitable for different types of PDEs and boundary conditions.
# 2. Fourier Transform
### 2.1 Definition and Properties of Fourier Transform
#### 2.1.1 Definition of Fourier Transform
A Fourier transform is an integral transform that converts a time-domain function into a frequency-domain function. For a real-valued function $f(t)$, its Fourier transform $F(\omega)$ is defined as:
```
F(\omega) = \int_{-\infty}^{\infty} f(t) e^{-i\omega t} dt
```
where $\omega$ is the angular frequency.
#### 2.1.2 Properties of Fourier Transform
The Fourier transform has the following properties:
***Linearity:** If $a$ and $b$ are constants, and $f(t)$ and $g(t)$ are time-domain functions, then:
```
F(a f(t) + bg(t)) = a F(f(t)) + b F(g(t))
```
***Time shifting:** If $f(t)$ is shifted by $\tau$ in the time domain, its Fourier transform $F(\omega)$ is shifted by $-2\pi\tau$ in the frequency domain:
```
F(f(t-\tau)) = e^{-i2\pi\tau\omega} F(\omega)
```
***Frequency modulation:** If $f(t)$ is multiplied by $e^{i\omega_0 t}$ in the time domain, then its Fourier transform $F(\omega)$ is shifted by $\omega_0$ in the frequency domain:
```
F(f(t) e^{i\omega_0 t}) = F(\omega - \omega_0)
```
***Parseval's Theorem:** The energy of the time-domain function $f(t)$ is equal to the energy of the frequency-domain function $F(\omega)$:
```
\int_{-\infty}^{\infty} |f(t)|^2 dt = \int_{-\infty}^{\infty} |F(\omega)|^2 d\omega
```
### 2.2 Applications of Fourier Transform in Solving PDEs
The Fourier transform is widely used in solving partial differential equations because it can transform PDEs into algebraic equations.
#### 2.2.1 Fourier Transform Solution of Linear PDEs
For linear PDEs, the Fourier transform can convert a PDE into a constant-coefficient algebraic equation. For example, for a one-dimensional heat conduction equation:
```
\frac{\partial u}{\partial t} = \alpha \frac{\partial^2 u}{\partial x^2}
```
Its Fourier transform is:
```
\frac{dU}{d\omega} = -i\alpha \omega^2 U
```
where $U(\omega)$ is the Fourier transform of $u(x, t)$. After solving the algebraic equation, $U(\omega)$ can be obtained, and then the inverse Fourier transform can be performed to obtain the analytical solution for $u(x, t)$.
#### 2.2.2 Fourier Transform Solution of Nonlinear PDEs
For nonlinear PDEs, the Fourier transform can convert nonlinear terms into algebraic terms, thus simplifying the solution process. For example, for a one-dimensional nonlinear wave equation:
```
\frac{\partial^2 u}{\partial t^2} = c^2 \frac{\partial^2 u}{\partial x^2} + \beta u^3
```
Its Fourier transform is:
```
-\omega^2 U = -c^2 \omega^2 U - \beta U^3
```
After solving the algebraic equation, $U(\omega)$ can be obtained, and then the inverse Fourier transform can be performed to obtain the approximate solution for $u(x, t)$.
# 3. Laplace Transform
A Laplace transform is a type of integral transform that converts a function from the time domain into the complex frequency domain. It is widely used in solving partial differential equations, especially in solving linear PDEs with constant coefficients.
### 3.1 Definition and Properties of Laplace Transform
#### 3.1.1 Definition of Laplace Transform
For a function $f(t)$ defined on the set of real numbers, its Laplace transform is defined as:
$$F(s) = \mathcal{L}[f(t)] = \int_0^\infty e^{-st} f(t) dt$$
where $s$ is a complex variable, and its real part $\Re(s)$ must be greater than zero.
#### 3.1.2 Properties of Laplace Transform
The Laplace transform has the following properties:
***Linearity:** For any constants $a$ and $b$, and functions $f(t)$ and $g(t)$, it holds that:
$$\mathcal{L}[af(t) + bg(t)] = a\mathcal{L}[f(t)] + b\mathcal{L}[g(t)]$$
***Time shifting:** For any constant $a$, it holds that:
$$\mathcal{L}[f(t-a)u(t-a)] = e^{-as}F(s)$$
where $u(t)$ is the unit step function.
***Differentiation:** For the $n$-th derivative $f^{(n)}(t)$ of the function $f(t)$, it holds that:
$$\mathcal{L}[f^{(n)}(t)] = s^nF(s) - s^{n-1}f(0^+) - s^{n-2}f'(0^+) - \cdots - f^{(n-1)}(0^+)$$
***Integration:** For the integral $\int_0^t f(\tau) d\tau$ of the function $f(t)$, it holds that:
$$\mathcal{L}\left[\int_0^t f(\tau) d\tau\right] = \frac{F(s)}{s}$$
### 3.2 Applications of Laplace Transform in Solving PDEs
#### 3.2.1 Laplace Transform Solution of Linear PDEs
Consider a linear P
0
0
相关推荐
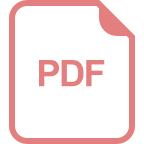
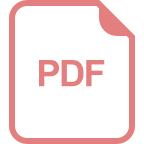
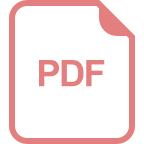
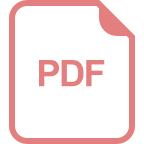
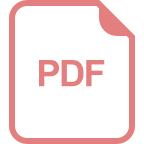
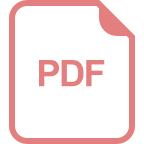
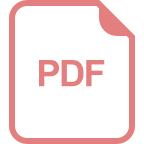
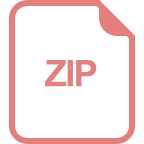