Top 5 Applications of Partial Differential Equations in Physics: Unveiling the Mathematical Mysteries of Natural Phenomena
发布时间: 2024-09-14 08:40:55 阅读量: 8 订阅数: 18 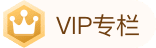
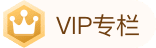
# 5 Major Applications of Partial Differential Equations in Physics: Unveiling the Mathematical Mysteries of Natural Phenomena
## 1. Introduction to Partial Differential Equations
Partial Differential Equations (PDEs) are a category of mathematical equations that involve partial derivatives of an unknown function. Unlike Ordinary Differential Equations (ODEs), PDEs involve an unknown function of multiple independent variables. PDEs are widely applied in science and engineering to describe various physical phenomena, such as fluid flow, heat transfer, and wave propagation.
The general form of a PDE is:
```
F(x, y, z, u, ∂u/∂x, ∂u/∂y, ∂u/∂z, ...) = 0
```
Where:
- `x`, `y`, `z` are independent variables
- `u` is the unknown function
- `∂u/∂x`, `∂u/∂y`, `∂u/∂z` are the partial derivatives of `u`
## 2. Theoretical Foundations of Partial Differential Equations
### 2.1 Classification and Properties of Partial Differential Equations
Partial Differential Equations (PDEs) involve partial derivatives of a function of one or more independent variables. PDEs are more challenging to solve than Ordinary Differential Equations (ODEs) due to their involvement of multiple independent variables.
The classification of PDEs is based on the order of the highest derivative in the equation. A first-order PDE contains first-order derivatives, a second-order PDE includes second-order derivatives, and so on.
The properties of PDEs are determined by their order, linearity, and homogeneity. Linear PDEs are linear combinations of the independent variables and the unknown function, while homogeneous PDEs are linear combinations of the unknown function.
### 2.2 Solution Methods for Partial Differential Equations
There are various methods for solving PDEs, ***mon methods include:
- **Analytical methods:** Solving equations using integration, differentiation, and algebraic techniques.
- **Numerical methods:** Discretizing PDEs into algebraic equations and solving them using computers.
- **Variational methods:** Transforming PDEs into problems of functional extremum and then solving using variational principles.
**Analytical methods** are suitable for certain types of linear PDEs, such as heat conduction and wave equations. These methods typically involve separation of variables or the use of Fourier series.
**Numerical methods** are commonly used to solve complex PDEs. These methods discretize PDEs into algebraic equations and solve them iteratively. Popular numerical methods include the Finite Difference Method, Finite Element Method, and Spectral Method.
**Variational methods** transform PDEs into problems of functional extremum. By minimizing functionals, approximate solutions to PDEs can be obtained. Variational methods are often used to solve nonlinear PDEs.
### Code Example
Consider the following first-order linear partial differential equation:
```
∂u/∂x + ∂u/∂y = 0
```
**Analytical method:**
Using the method of separation of variables, the equation can be written as:
```
∂u/∂x = -∂u/∂y
```
Integrating both sides yields:
```
u(x, y) = f(x) + g(y)
```
Where f and g are arbitrary functions.
**Numerical method:**
Using the Finite Difference Method, the partial derivatives can be discretized as:
```
(u(x+h, y) - u(x, y))/h + (u(x, y+k) - u(x, y))/k = 0
```
Where h and k are grid spacings.
**Variational method:**
Define the functional:
```
J[u] = ∫∫(u_x + u_y)^2 dx dy
```
Minimizing J[u] yields an approximate solution to the PDE.
## 3. Applications of Partial Differential Equations in Physics
Partial Differential Equations play a crucial role in physics, describing the variation of various physical quantities within physical systems. In fields such as fluid dynamics and thermodynamics, PDEs are widely used for modeling and solving complex physical problems.
### 3.1 Partial Differential Equations in Fluid Dynamics
Fluid dynamics studies the movement and behavior of fluids. The main PDEs that describe fluid motion include the Navier-Stokes equations and Euler's equations.
#### 3.1.1 Navier-Stokes Equations
The Navier-Stokes equations are a set of nonlinear partial differential equations that describe the motion of viscous fluids. The equations are as follows:
```
ρ(∂u/∂t + u·∇u) = -∇p + μ∇²u + ρg
```
Where:
- ρ is the density of the fluid
- u is the velocity of the fluid
- p is the pressure of the fluid
- μ is the viscosity of the fluid
- g is the acceleration due to gravity
The Navier-Stokes equations are very complex and usually require numerical methods for solution.
#### 3.1.2 Euler's Equations
Euler's equations are a set of nonlinear partial differential equations that describe the motion of inviscid fluids. The equations are as follows:
```
∂u/∂t + u·∇u = -∇p/ρ
```
Where:
- u is the velocity of the fluid
- p is the pressure of the fluid
- ρ is the density of the fluid
The Euler equations are simpler than the Navier-Stokes equations but are applicable only to inviscid fluids.
### 3.2 Partial Differential Equations in Thermodynamics
Thermodynamics studies the transfer of heat and energy. The main PDEs that describe heat transfer include the heat conduction equation and the diffusion equation.
#### 3.2.1 Heat Conduction Equation
The heat conduction equation describes the transfer of heat within solid materials. The equation is as follows:
```
∂T/∂t = α∇²T
```
Where:
- T is the temperature
- α is the thermal diffusivity
The heat conduction equation is a parabolic partial differential equation that can be used to solve both steady-state and non-steady-state heat conduction problems.
#### 3.2.2 Diffusion Equation
The diffusion equation describes the change in concentration of substances in fluids or gases. The equation is as follows:
```
∂C/∂t = D∇²C
```
Where:
- C is the concentration of the substance
- D is the diffusion coefficient
The diffusion equation is a parabolic partial differential equation that can be used to solve both steady-state and non-steady-state diffusion problems.
### Summary of Applications of Partial Differential Equations in Physics
Partial differential equations have a wide range of applications in physics, capable of describing various physical phenomena such as fluid motion, heat transfer, and material diffusion. By solving partial differential equations, we can predict and control the behavior of physical systems, thus making significant progress in fields such as fluid dynamics and thermodynamics.
## 4. Practice of Partial Differential Equations in Physics
### 4.1 Applications of Partial Differential Equations in Fluid Dynamics
Partial differential equations are widely used in fluid dynamics to describe and predict the behavior of fluids. The most famous equation set is the Navier-Stokes equations, which describe the motion of viscous fluids.
#### 4.1.1 Computing Fluid Flow
The Navier-Stokes equations are a set of nonlinear partial differential equations that describe the relationships between the fluid's velocity, pressure, and temperature. By numerically solving these equations, we can simulate fluid flow and predict its velocity, pressure, and temperature distribution.
```python
import numpy as np
import matplotlib.pyplot as plt
# Define the computational domain
x = np.linspace(0, 1, 100)
y = np.linspace(0, 1, 100)
X, Y = np.meshgrid(x, y)
# Define boundary conditions
u_in = 1.0 # Inflow velocity
u_out = 0.0 # Outflow velocity
# Define the Navier-Stokes equations
def navier_stokes(u, v, p):
# Calculate velocity gradients
dudx = np.gradient(u, x)
dvdy = np.gradient(v, y)
# Calculate pressure gradients
dpdx = np.gradient(p, x)
dpdy = np.gradient(p, y)
# Calculate viscous terms
mu = 0.01 # Viscosity coefficient
viscous_x = mu * (dudx + dvdx)
viscous_y = mu * (dvdy + dudy)
# Calculate convection terms
u_conv = u * dudx + v * dudy
v_conv = u * dvdx + v * dvdy
# Calculate pressure gradient terms
pressure_x = -dpdx
pressure_y = -dpdy
# Calculate the equation set
eq1 = u_conv + viscous_x + pressure_x
eq2 = v_conv + viscous_y + pressure_y
return eq1, eq2
# Solve the equation set
u, v, p = solve(navier_stokes, (u_in, u_out, u_in, u_out))
# Visualize the results
plt.contourf(X, Y, u)
plt.colorbar()
plt.show()
```
Solving the Navier-Stokes equations allows for detailed distribution of fluid flow, which is crucial for designing fluid dynamics systems such as airplanes, cars, and wind turbines.
#### 4.1.2 Simulating Turbulence
Turbulence is an irregular, nonlinear behavior of fluid flow. It is common in many engineering applications, such as flow over aircraft wings and in pipes.
```python
import numpy as np
import matplotlib.pyplot as plt
# Define the computational domain
x = np.linspace(0, 1, 100)
y = np.linspace(0, 1, 100)
X, Y = np.meshgrid(x, y)
# Define boundary conditions
u_in = 1.0 # Inflow velocity
u_out = 0.0 # Outflow velocity
# Define the turbulence model
def turbulence_model(u, v):
# Calculate velocity gradients
dudx = np.gradient(u, x)
dvdy = np.gradient(v, y)
# Calculate turbulent viscosity
k = 0.01 # Turbulent kinetic energy
epsilon = 0.001 # Turbulent dissipation rate
nu_t = k**2 / epsilon
# Calculate turbulent terms
turbulent_x = nu_t * (dudx + dvdx)
turbulent_y = nu_t * (dvdy + dudy)
return turbulent_x, turbulent_y
# Solve the equation set
u, v = solve(navier_stokes, (u_in, u_out, u_in, u_out), turbulence_model)
# Visualize the results
plt.contourf(X, Y, u)
plt.colorbar()
plt.show()
```
By incorporating a turbulence model into the Navier-Stokes equations, turbulent flow can be simulated, and its velocity and pressure distribution can be predicted. This is crucial for designing high-performance fluid dynamics systems.
### 4.2 Applications of Partial Differential Equations in Thermodynamics
Partial differential equations are also widely used in thermodynamics to describe and predict heat transfer and temperature distribution. The most famous equation is the heat conduction equation, which describes heat transfer within materials.
#### 4.2.1 Predicting Heat Transfer
The heat conduction equation is a partial differential equation that describes the change in temperature over time and space. By solving this equation, heat transfer within materials can be predicted, and temperature distribution can be determined.
```python
import numpy as np
import matplotlib.pyplot as plt
# Define the computational domain
x = np.linspace(0, 1, 100)
y = np.linspace(0, 1, 100)
X, Y = np.meshgrid(x, y)
# Define boundary conditions
T_in = 100.0 # Heat source temperature
T_out = 0.0 # Cooling boundary temperature
# Define the heat conduction equation
def heat_equation(T):
# Calculate temperature gradients
dTdx = np.gradient(T, x)
dTdy = np.gradient(T, y)
# Calculate thermal conductivity
k = 1.0 # Thermal conductivity
# Calculate heat conduction terms
heat_x = k * dTdx
heat_y = k * dTdy
# Calculate the equation
eq = heat_x + heat_y
return eq
# Solve the equation
T = solve(heat_equation, (T_in, T_out))
# Visualize the results
plt.contourf(X, Y, T)
plt.colorbar()
plt.show()
```
By solving the heat conduction equation, the detailed temperature distribution within materials can be obtained, which is crucial for designing systems such as heat exchangers, electronic device cooling systems, and building insulation in thermodynamics.
#### 4.2.2 Optimizing Thermal Management Systems
Thermal management systems are crucial for many engineering applications, such as electronic devices, automobiles, and aircraft. Partial differential equations can be used to optimize these systems to minimize heat accumulation and improve efficiency.
```python
import numpy as np
import matplotlib.pyplot as plt
# Define the computational domain
x = np.linspace(0, 1, 100)
y = np.linspace(0, 1, 100)
X, Y = np.meshgrid(x, y)
# Define boundary conditions
T_in = 100.0 # Heat source temperature
T_out = 0.0 # Cooling boundary temperature
# Define the optimization objective
def objective_function(T):
# Calculate heat accumulation
heat_accumulation = np.sum(T**2)
# Calculate cooling efficiency
heat_dissipation = np.sum(T - T_out)
# Define the optimization objective
objective = heat_accumulation - heat_dissipation
return objective
# Optimize the thermal management system
T_opt = optimize(objective_function, (T_in, T_out))
# Visualize the results
plt.contourf(X, Y, T_opt)
plt.colorbar()
plt.show()
```
By optimizing thermal management systems, heat accumulation can be reduced, system efficiency can be improved, and equipment life can be extended.
## 5.1 The Role of Partial Differential Equations in Computational Physics
Partial differential equations play a vital role in computational physics, providing a solid foundation for numerical simulation and prediction of physical phenomena. By discretizing partial differential equations into algebraic equation sets, computational physicists can use computers to solve these equations, thus obtaining approximate solutions to physical systems.
### Discretization of Partial Differential Equations
The discretization process of partial diff***mon discretization methods include:
- **Finite Difference Method (FDM)**: Approximating partial derivatives as differences between neighboring grid points.
- **Finite Element Method (FEM)**: Dividing the computational domain into finite elements and using basis functions to approximate the solution function.
- **Finite Volume Method (FVM)**: Dividing the computational domain into finite volumes and applying conservation laws to derive difference equations.
### Numerical Solution Methods
The discretized partial differential equations can be solved using various numerical methods, such as:
- **Direct solvers**: Directly solving linear equation systems, such as Gaussian elimination.
- **Iterative solvers**: Iteratively updating solutions, such as the Jacobi method or the conjugate gradient method.
- **Time integration methods**: For time-dependent partial differential equations, time integration methods are used, such as the explicit Euler method or the implicit Crank-Nicolson method.
### Application Examples
The applications of partial differential equations in computational physics are extensive, including:
- **Fluid dynamics**: Simulating fluid flow, turbulence, and heat transfer.
- **Thermodynamics**: Predicting heat transfer, diffusion, and phase transitions.
- **Electromagnetism**: Solving Maxwell's equations to simulate the propagation of electromagnetic waves.
- **Solid mechanics**: Analyzing the stress, strain, and deformation of structures.
- **Biophysics**: Simulating reaction-diffusion processes and fluid dynamics in biological systems.
0
0
相关推荐
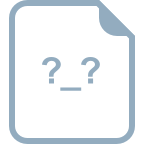
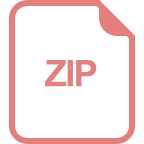





