Kafka Message Queue Hands-On: From Beginner to Expert
发布时间: 2024-09-13 20:18:31 阅读量: 12 订阅数: 18 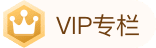
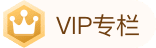
# Kafka Message Queue Practical: From Beginner to Expert
## 1. Overview of Kafka Message Queue
Kafka is a distributed streaming platform designed for building real-time data pipelines and applications. It offers a high-throughput, low-latency messaging queue capable of handling vast amounts of data. The architecture and features of Kafka make it an ideal choice for constructing reliable, scalable, and fault-tolerant streaming systems.
The key components of Kafka include producers, consumers, topics, and partitions. Producers publish messages to topics, ***ics are divided into partitions for parallel processing and scalability. Kafka also provides features for persistence, replication, and fault tolerance, ensuring reliable message delivery.
## 2.1 Kafka Architecture and Components
### Kafka Cluster Architecture
Kafka is a distributed streaming platform, and its architecture consists of the following components:
- **Broker:** Server nodes in the Kafka cluster responsible for storing and managing messages.
- **Topic:** A logical grouping of messages used for organizing and managing different types of messages.
- **Partition:** Physical subdivisions of a topic, each partition consists of a Leader and multiple Followers.
- **Producer:** Applications or components that send messages to the Kafka cluster.
- **Consumer:** Applications or components that receive messages from the Kafka cluster.
- **ZooKeeper:** A distributed coordination service used for coordinating and managing the Kafka cluster.
### Kafka Message Stream Processing Flow
The Kafka message stream processing flow is as follows:
1. **Producer sends messages to Topic:** The Producer sends messages to a specific Topic, which consists of one or more Partitions.
2. **Partition Leader receives messages:** Each Partition has a Leader responsible for receiving and replicating messages.
3. **Followers replicate messages:** Followers replicate messages from the Leader to ensure redundancy and availability.
4. **Consumer reads messages from Partition:** Consumers subscribe to specific Topics and read messages from Partitions.
### Component Interaction
Components within a Kafka cluster interact to process messages:
- **Producer and Broker:** Producers send messages to Brokers, which store messages in Partitions.
- **Broker and ZooKeeper:** Brokers communicate with ZooKeeper to coordinate metadata information within the cluster, such as Topics, Partitions, and Leader assignments.
- **Consumer and Broker:** Consumers subscribe to Topics from Brokers and pull messages from Partitions.
- **Follower and Leader:** Followers periodically replicate messages from Leaders to keep replicas synchronized.
### Component Responsibilities
Each component in the Kafka cluster has specific responsibilities:
- **Producer:** Responsible for generating and sending messages.
- **Broker:** Responsible for storing and managing messages and coordinating metadata information within the cluster.
- **Consumer:** Responsible for receiving and processing messages from the Kafka cluster.
- **ZooKeeper:** Responsible for coordinating and managing the Kafka cluster and storing cluster metadata information.
- **Partition:** Responsible for storing and managing messages within a Topic and ensuring message reliability and availability.
## 3.1 Implementation of Message Production and Consumption
### Message Production
Message producers are responsible for publishing messages to the Kafka cluster. Kafka provides two types of producer APIs: synchronous and asynchronous producers.
### Synchronous Producer
Synchronous producers block after sending messages until they receive confirmation from the Kafka cluster. This method ensures messages are successfully written to Kafka but reduces throughput.
```java
import org.apache.kafka.clients.producer.KafkaProducer;
import org.apache.kafka.clients.producer.ProducerConfig;
import org.apache.kafka.clients.producer.ProducerRecord;
import java.util.Properties;
public class KafkaProducerExample {
public static void main(String[] args) {
// Configure producer properties
Properties properties = new Properties();
properties.put(ProducerConfig.BOOTSTRAP_SERVERS_CONFIG, "localhost:9092");
properties.put(ProducerConfig.KEY_SERIALIZER_CLASS_CONFIG, "***mon.serialization.StringSerializer");
properties.put(ProducerConfig.VALUE_SERIALIZER_CLASS_CONFIG, "***mon.serialization.StringSerializer");
// Create a producer
KafkaProducer<String, String> producer = new KafkaProducer<>(properties);
// Create a message record
ProducerRecord<String, String> record = new ProducerRecord<>("my-topic", "Hello, Kafka!");
// Synchronously send the message
producer.send(record).get();
// Close the producer
producer.close();
}
}
```
### Parameters Explanation:
- `BOOTSTRAP_SERVERS_CONFIG`: The bootstrap server address of the Kafka cluster.
- `KEY_SERIALIZER_CLASS_CONFIG`: The serializer class used for serializing the message key.
- `VALUE_SERIALIZER_CLASS_CONFIG`: The serializer class used for serializing the message value.
### Logical Analysis:
1. Configure producer properties, including bootstrap server address, serializer classes, etc.
2. Create a KafkaProducer instance.
3. Create a message record with specified topic and message content.
4. Synchronously send the message and block until confirmation from the Kafka cluster is received.
5. Close the producer.
### Asynchronous Producer
Asynchronous producers do not block after sending messages but instead place messages into a buffer and continue sending other messages. This method increases throughput but may result in message loss.
```java
import org.apache.kafka.clients.producer.KafkaProducer;
import org.apache.kafka.clients.producer.ProducerConfig;
import org.apache.kafka.clients.producer.ProducerRecord;
import java.util.Properties;
public class KafkaAsyncProducerExample {
public static void main(String[] args) {
// Configure producer properties
Properties properties = new Properties();
properties.put(ProducerConfig.BOOTSTRAP_SERVERS_CONFIG, "localhost:9092");
properties.put(ProducerConfig.KEY_SERIALIZER_CLASS_CONFIG, "***mon.serialization.StringSerializer");
properties.put(ProducerConfig.VALUE_SERIALIZER_CLASS_CONFIG, "***mon.serialization.StringSerializer");
// Create a producer
KafkaProducer<String, String> producer = n
```
0
0
相关推荐
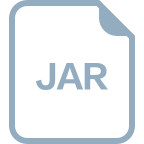
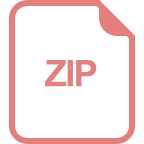
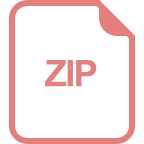





