MATLAB Performance Optimization for Reading Excel Data: 3 Secrets to Speed Up Data Import
发布时间: 2024-09-13 19:37:50 阅读量: 28 订阅数: 23 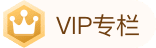
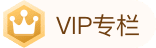
# Overview of MATLAB Reading Excel Data
MATLAB is a programming language widely used for scientific computation and data analysis. It offers various functions to read and process Excel data, including `xlsread`, `importdata`, and `readtable`. These functions can extract data from Excel files and convert it into MATLAB data structures such as arrays, tables, or structs.
When reading Excel data, MATLAB needs to parse the file format, convert data types, and store them in memory. This process can be time-consuming, especially for large or complex datasets. Therefore, it is crucial to understand the performance bottlenecks when MATLAB reads Excel data to take measures for optimization.
# Performance Bottleneck Analysis of MATLAB Reading Excel Data
### 2.1 Data Scale and Complexity
**Issue:**
The scale and complexity of data are key factors affecting performance when MATLAB reads Excel data. Large datasets and complex data structures (such as nested tables, formulas, and charts) can slow down the reading process.
**Analysis:**
***Data Scale:** The larger the dataset, the longer the reading time.
***Data Complexity:** Complex data structures require more parsing and conversion, increasing processing time.
### 2.2 Data Type Conversion
**Issue:**
When MATLAB reads Excel data, it needs to convert Excel data types into MATLAB data types. This process can be time-consuming, especially when there are data type mismatches.
**Analysis:**
***Data Type Mismatch:** For example, converting Excel's date and time data into MATLAB's numeric arrays requires complex conversions.
***Data Type Conversion Efficiency:** Different data type conversions have different efficiencies, for example, converting from text to numbers is faster than converting from text to dates.
### 2.3 Memory Management
**Issue:**
MATLAB needs to allocate memory to store data when reading Excel data. Improper memory management can lead to performance issues such as insufficient memory or fragmentation.
**Analysis:**
***Memory Allocation:** MATLAB needs to allocate enough memory to store the read data. If memory is insufficient, the reading process may fail.
***Memory Fragmentation:** When MATLAB allocates and frees memory multiple times, it can lead to memory fragmentation, reducing reading performance.
**Code Block 1:**
```matlab
% Read Excel data
data = xlsread('data.xlsx');
% Analyze memory usage
memory_info = memory;
disp(['Memory usage: ', num2str(memory_info.MemUsedBytes)]);
```
**Logical Analysis:**
This code reads Excel data and analyzes memory usage. The `xlsread` function reads the data, and the `memory` function obtains memory usage information.
**Parameter Explanation:**
* `data`: MATLAB variable that stores the read data.
* `memory_info`: Structure that contains memory usage information.
* `MemUsedBytes`: Number of bytes of memory used.
# 3.1 Use Appropriate Data Types
When MATLAB reads Excel data, data type conversion can significantly affect performance. By default, MATLAB imports Excel data as double-precision floating-point numbers, which can lead to unnecessary memory consumption and computational overhead.
To optimize performance, appropriate data types should be used based on the actual data types. For example, if the data is integers, it should be imported as `int32` or `int64`; if the data is boolean values, it should be imported as `logical`.
The following code example demonstrates how to import Excel data using appropriate data types:
```matlab
% Read Excel data
data = readtable('data.xlsx');
% Convert numeric columns to integers
data.Age = int32(data.Age);
data.Salary = int64(data.Salary);
% Convert boolean columns to logical values
data.IsEmployed = logical(data.IsEmployed);
```
### 3.2 Reduce Data Conversion
Data conversion is another common performance bottleneck when MATLAB reads Excel data. When there is a data type mismatch, MATLAB needs to convert the data before importing it.
To reduce data conversion, ensure that the data types in the Excel data match the expected data types in MATLAB. If there is a data type mismatch, explicitly convert the data before importing.
The following code example demonstrates how to reduce data conversion:
```matlab
% Read Excel data
data = readtable('data.xlsx', 'ReadVariableNames', false);
% Determine data types
dataTypes = cellfun(@class, data{1, :});
% Convert data types
for i = 1:numel(dataTypes)
switch dataTypes{i}
case 'double'
data{1, i} = double(data{1, i});
case 'int32'
data{1, i} = int32(data{1, i});
case 'int64'
data{1, i} = int64(data{1, i});
case 'logical'
data{1, i} = logical(data{1, i});
end
end
```
### 3.3 Optimize Memory Management
Memory management is another important performance factor when MATLAB reads Excel data. When MATLAB imports large datasets, it needs to allocate a significant amount of memory to store the data. If there is insufficient memory, MATLAB may experience performance issues or even crash.
To optimize memory management, use the `PreserveVariableNames` and `ReadVariableNames` options of the `readtable` function. These options allow you to control how MATLAB stores data, reducing memory consumption.
The following code example demonstrates how to optimize memory management:
```matlab
% Read Excel data without preserving variable names
data = readtable('data.xlsx', 'PreserveVariableNames', false);
% Read Excel data, only read specified variables
data = readtable('data.xlsx', 'ReadVariableNames', {'Age', 'Salary', 'IsEmployed'});
```
# 4. Advanced Performance Optimization for MATLAB Reading Excel Data
This chapter will delve into more advanced optimization techniques to further enhance the performance when MATLAB reads Excel data.
### 4.1 Parallelizing Data Import
Parallelizing data import can significantly increase the reading speed of large Excel datasets. MATLAB provides the `parfor` loop, which allows tasks to be executed in parallel on multiple processor cores.
**Code Block:**
```matlab
% Create a large Excel dataset
data = rand(100000, 1000);
xlswrite('large_data.xlsx', data);
% Parallel read Excel data
parfor i = 1:size(data, 1)
data_row = xlsread('large_data.xlsx', i, 1:size(data, 2));
% Process each row of data
end
```
**Logical Analysis:**
The `parfor` loop distributes the data import tasks across multiple processor cores. Each row of data is processed by a different core, achieving parallelization.
### 4.2 Using External Libraries
The MATLAB community offers many external libraries that can optimize Excel data reading performance. Examples include:
- **readxl:** A fast and memory-efficient Excel reading library.
- **xlwings:** A library that allows direct interaction with Excel workbooks in MATLAB.
**Code Block:**
```matlab
% Use readxl to read Excel data
data = readxl('large_data.xlsx');
% Use xlwings to read Excel data
app = xlwings.App();
wb = app.books.open('large_data.xlsx');
data = wb.sheets(1).range('A1:J100000').value;
```
**Logical Analysis:**
The `readxl` library reads Excel data using efficient algorithms, while the `xlwings` library allows direct interaction with Excel objects, enhancing flexibility.
### 4.3 Optimizing Code Structure
Optimizing the code structure can reduce unnecessary computation and memory overhead. Here are some suggestions:
- Avoid using nested loops.
- Use pre-allocated arrays.
- Avoid unnecessary variable creation and destruction.
**Code Block:**
```matlab
% Optimize code structure
data = xlsread('large_data.xlsx');
% Pre-allocate arrays
data_optimized = zeros(size(data));
% Avoid nested loops
for i = 1:size(data, 1)
for j = 1:size(data, 2)
data_optimized(i, j) = data(i, j);
end
end
```
**Logical Analysis:**
By pre-allocating arrays and avoiding nested loops, unnecessary memory allocation and computation are reduced.
# 5.1 Importing Large Excel Datasets
When dealing with large Excel datasets, MATLAB's performance can be affected. To optimize import speed, the following tips can be used:
**1. Use Chunk Importing**
Chunk importing divides large datasets into smaller blocks and imports them into MATLAB one by one. This reduces the amount of data loaded into memory at once, improving performance.
```matlab
% Import large Excel dataset
data = readtable('large_dataset.xlsx', 'Sheet', 'Sheet1', 'Range', 'A1:Z10000');
% Chunk importing
chunkSize = 1000;
for i = 1:chunkSize:size(data, 1)
chunk = data(i:min(i+chunkSize-1, size(data, 1)), :);
% Process the data chunk
end
```
**2. Use Parallel Importing**
MATLAB supports parallelization, which can use multiple processors to import data simultaneously. This can significantly improve the import speed of large datasets.
```matlab
% Parallel import large Excel dataset
data = parallel.import('large_dataset.xlsx', 'Sheet', 'Sheet1', 'Range', 'A1:Z10000');
% Wait for import to complete
wait(data);
% Get imported data
data = data.Value;
```
**3. Use External Libraries**
The MATLAB community offers many external libraries for reading Excel data, which are often optimized for performance. For example, the `readxl` library can import large Excel datasets faster than MATLAB's built-in functions.
```matlab
% Use the readxl library to import large Excel data
data = readxl('large_dataset.xlsx', 'Sheet', 'Sheet1', 'Range', 'A1:Z10000');
```
## 5.2 Optimizing Data Type Conversions
When MATLAB imports Excel data, it automatically converts the data into MATLAB data types. However, this conversion can lead to performance degradation, especially when data types do not match.
**1. Specify Data Types**
When importing data, you can use the `DataType` option to specify the data type to be converted. This can avoid unnecessary conversions, improving performance.
```matlab
% Specify data types
data = readtable('data.xlsx', 'DataType', 'double');
```
**2. Use Appropriate Data Types**
MATLAB offers a variety of data types, and choosing the appropriate one can optimize performance. For example, for numerical data, using the `double` type is more efficient than the `string` type.
```matlab
% Choose appropriate data types
data = readtable('data.xlsx', 'DataType', {'double', 'string', 'logical'});
```
## 5.3 Reducing Memory Consumption
When MATLAB imports Excel data, it stores the data in memory. For large datasets, this can lead to insufficient memory. The following tips can be used to reduce memory consumption:
**1. Avoid Creating Unnecessary Variables**
When processing Excel data, avoid creating unnecessary variables. For example, if you only need data from specific columns, import only those columns instead of the entire dataset.
```matlab
% Avoid creating unnecessary variables
data = readtable('data.xlsx', 'Range', 'A1:C10000');
```
**2. Use Sparse Matrices**
For sparse data containing many zero values, using sparse matrices can reduce memory consumption. Sparse matrices only store non-zero elements, saving space.
```matlab
% Use sparse matrices
data = sparse(readtable('data.xlsx', 'Range', 'A1:C10000'));
```
**3. Use External Storage**
For very large datasets, using external storage (such as databases or files) to store data can reduce memory consumption in MATLAB.
```matlab
% Use external storage
conn = database('database_name', 'username', 'password');
data = fetch(conn, 'SELECT * FROM table_name');
```
# 6. Summary of MATLAB Reading Excel Data Performance Optimization**
When optimizing MATLAB reading Excel data performance, multiple factors need to be considered, including data scale, data types, memory management, parallelization, external libraries, and code structure.
By using appropriate data types, reducing data conversion, and optimizing memory management, data import speed can be significantly improved. In addition, advanced optimization techniques such as parallel data importing, using external libraries, and optimizing code structure can further enhance performance.
In practice, these optimization techniques can be combined and adjusted according to specific datasets and application scenarios. For example, for large datasets, parallel data importing can significantly shorten import time; for scenarios with frequent data type conversions, using external libraries can provide faster conversion speeds; for complex code structures, optimizing the code structure can reduce unnecessary computation and memory consumption.
Through in-depth understanding and optimization of MATLAB reading Excel data performance, data processing efficiency can be significantly improved, meeting the needs of various application scenarios.
0
0
相关推荐
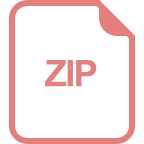
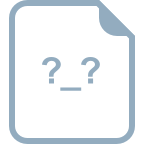
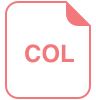
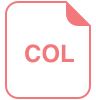
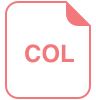
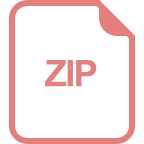
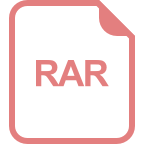
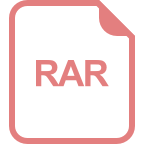
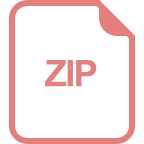