Introduction to Common Data Science Tools in Jupyter Notebook
发布时间: 2024-09-15 17:46:44 阅读量: 14 订阅数: 18 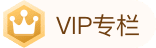
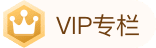
# 1. Introduction to Jupyter Notebook
Jupyter Notebook is an open-source interactive computing tool that is widely used for data cleaning and transformation, numerical simulation, statistical modeling, data visualization, and machine learning, among other fields. It supports over 40 programming languages, including Python, R, and Julia. The flexibility of Jupyter Notebook allows data scientists to write, experiment, and present results in one place.
## What is Jupyter Notebook?
Jupyter Notebook is an interactive computing environment based on an open-source web application that allows users to write and run code in a notebook format. Users can write executable code in the notebook, combining rich text, formulas, images, and charts.
## Advantages of Jupyter Notebook
- **Interactive Computing**: Ability to run code in real-time, view results, and quickly iterate for improvements.
- **Documented**: Supports Markdown format, integrating code, text, and charts into a single document.
- **Easy Sharing**: Can be exported to formats such as HTML and PDF for easy sharing with others.
- **Support for Multiple Programming Languages**: In addition to Python, it supports R, Julia, and other mainstream programming languages.
- **Rich Extensions**: Boasts a plethora of plugins and extension libraries to meet various needs.
- **Graphical Interface**: Facilitates visualization of data and results, aiding in data analysis and presentation.
## How to Install Jupyter Notebook
Installing Jupyter Notebook is straightforward and can be done using the pip package manager:
```bash
pip install jupyterlab
```
After installation, start Jupyter Notebook:
```bash
jupyter notebook
```
You can then open the Jupyter Notebook interface in your browser and begin coding and documenting.
# 2. Data Processing Tools
Data processing is a crucial part of data science projects, and common data processing tools in Jupyter Notebook include the Pandas and Numpy libraries. We will now delve into their usage.
#### 2.1 Introduction to Pandas Library
Pandas is a library in Python for data processing and analysis, which provides a data structure known as DataFrame, making data manipulation simpler and more efficient. Here are some of Pandas' commonly used features:
- Data Reading: Can read from various data sources, such as CSV files, SQL databases, Excel files, etc.
- Data Cleaning: Handles missing values, duplicates, outliers, etc., to make data cleaner.
- Data Filtering: Filters data based on conditions to extract the necessary parts.
- Data Aggregation: Performs statistical analysis and aggregates data.
Below is a simple example of Pandas code that reads a CSV file and displays the first few rows:
```python
import pandas as pd
# Reading CSV file
data = pd.read_csv('data.csv')
# Displaying the first five rows of data
print(data.head())
```
#### 2.2 Introduction to Numpy Library
Numpy is a library in Python for scientific computing, primarily used for handling multidimensional arrays and matrix operations. Numpy provides efficient mathematical functions and tools suitable for processing large-scale data. Here are some of Numpy's features:
- Array Operations: Numpy arrays perform fast vectorized operations, enhancing computational efficiency.
- Logical Operations: Capable of logical operations and boolean indexing.
- Mathematical Functions: Provides a wide range of mathematical functions such as sin, cos, exp, etc.
- Linear Algebra Operations: Supports matrix multiplication, inversion, and other linear algebra operations.
Below is a simple example of Numpy code that creates a two-dimensional array and performs matrix multiplication:
```python
import numpy as np
# Creating arrays
arr1 = np.array([[1, 2], [3, 4]])
arr2 = np.array([[2, 0], [1, 3]])
# Matrix multiplication operation
result = np.dot(arr1, arr2)
print(result)
```
#### 2.3 Data Cleaning and Processing Tips
In actual data processing, it is often necessary to clean and process data to ensure data quality. Some commonly used data cleaning and processing tips include:
- Handling Missing Values: Use the `dropna()` or `fillna()` functions in the Pandas library to address missing values.
- Removing Duplicates: Utilize the `drop_duplicates()` function in the Pandas library to eliminate duplicates.
- Dealing with Outliers: Identify and handle outliers using statistical methods or visualization techniques.
- Data Transformation: Perform standardization, normalization, discretization, and other data transformations.
- Feature Engineering: Create new features or combine features to extract more useful information.
By mastering these data processing tools and techniques, data can be processed more efficiently, laying a foundation for subsequent analysis and modeling work.
# 3. Data Visualization Tools
Data visualization plays a vital role in data science projects because intuitive and clear charts help us better understand data, discover trends, and patterns. In Jupyter Notebook, there are many commonly used data visualization tools, including Matplotlib, Seaborn, and Plotly, among others. We will now discuss their characteristics and how to apply these tools for data visualization in projects.
#### 3.1 Introduction to Matplotlib Library
Matplotlib is one of the most widely used plotting libraries in Python. It offers a rich set of plotting features and is capable of creating various types of charts, such as line charts, scatter plots, bar charts, etc. Below are some of the features of Matplotlib:
- Supports multiple chart styles
- Highly flexible with the ability to customize various parts of the chart in detail
- User-friendly with comprehensive documentation and an active community
#### 3.2 Introduction to Seaborn Library
Seaborn is a high-level plotting library built on top of Matplotlib, focusing on statistical visualization. It allows for the creation of beautiful statistical cha
0
0
相关推荐
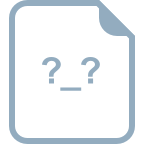
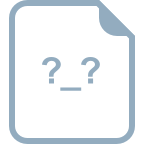
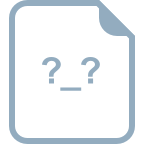





