class Inception(nn.Module):
时间: 2023-10-23 19:48:32 浏览: 76
这段代码定义了一个名为 Inception 的 PyTorch 模块类,用于实现 Inception 神经网络模型。Inception 是由 Google 团队开发的一种深度学习神经网络模型,旨在解决图像分类和识别问题。该模型使用了一种称为 "卷积神经网络" 的方法,可以自动从大量标记图像中学习特征,并将其用于对新图像进行分类。
Inception 神经网络模型由多个 Inception 模块组成,每个 Inception 模块包含多个卷积层和池化层,并使用了一种类似于并行计算的结构来提取输入图像的多层特征。这个类实现了一个 Inception 模块,并使用了多个 BasicConv2d 类来实现不同的卷积和池化操作。其中,每个 Inception 模块包含了多个分支,每个分支都会对输入进行不同的卷积和池化操作,并将最终的特征图拼接在一起。这种并行计算的结构可以提高网络的特征提取能力,从而提升模型的分类性能。
相关问题
class Inception(nn.Module): # c1--c4是每条路径的输出通道数 def __init__(self, in_channels, c1, c2, c3, c4, **kwargs): super(Inception, self).__init__(**kwargs) # 线路1,单1x1卷积层 self.p1_1 = nn.Conv2d(in_channels, c1, kernel_size=1) # 线路2,1x1卷积层后接3x3卷积层 self.p2_1 = nn.Conv2d(in_channels, c2[0], kernel_size=1) self.p2_2 = nn.Conv2d(c2[0], c2[1], kernel_size=3, padding=1) # 线路3,1x1卷积层后接5x5卷积层 self.p3_1 = nn.Conv2d(in_channels, c3[0], kernel_size=1) self.p3_2 = nn.Conv2d(c3[0], c3[1], kernel_size=5, padding=2) # 线路4,3x3最大汇聚层后接1x1卷积层 self.p4_1 = nn.MaxPool2d(kernel_size=3, stride=1, padding=1) self.p4_2 = nn.Conv2d(in_channels, c4, kernel_size=1)
这是一个Inception模块的代码实现,它是Google Inception网络中的一种基本模块。Inception模块主要是为了解决卷积网络中卷积核大小和感受野大小的问题,通过在同一层网络中使用不同大小的卷积核和池化操作,来获得更好的特征表示。这个模块包含了4条不同的路径,每条路径都由1个或多个卷积层和池化层组成,最后将每条路径的输出在通道维度上拼接起来作为模块的输出。
Inception.__init__() missing 2 required positional arguments: 'ch5x5' and 'pool_proj'
非常抱歉,我刚才的代码有误,已经修正。以下是正确的代码:
```python
import torch.nn as nn
import torch.nn.functional as F
class Inception(nn.Module):
def __init__(self, in_channels, ch1x1, ch3x3red, ch3x3, ch5x5red, ch5x5, pool_proj):
super(Inception, self).__init__()
# 1x1 conv branch
self.branch1 = nn.Sequential(
nn.Conv2d(in_channels, ch1x1, kernel_size=1),
nn.BatchNorm2d(ch1x1),
nn.ReLU(inplace=True)
)
# 1x1 conv -> 3x3 conv branch
self.branch2 = nn.Sequential(
nn.Conv2d(in_channels, ch3x3red, kernel_size=1),
nn.BatchNorm2d(ch3x3red),
nn.ReLU(inplace=True),
nn.Conv2d(ch3x3red, ch3x3, kernel_size=3, padding=1),
nn.BatchNorm2d(ch3x3),
nn.ReLU(inplace=True)
)
# 1x1 conv -> 5x5 conv branch
self.branch3 = nn.Sequential(
nn.Conv2d(in_channels, ch5x5red, kernel_size=1),
nn.BatchNorm2d(ch5x5red),
nn.ReLU(inplace=True),
nn.Conv2d(ch5x5red, ch5x5, kernel_size=5, padding=2),
nn.BatchNorm2d(ch5x5),
nn.ReLU(inplace=True)
)
# 3x3 pool -> 1x1 conv branch
self.branch4 = nn.Sequential(
nn.MaxPool2d(kernel_size=3, stride=1, padding=1),
nn.Conv2d(in_channels, pool_proj, kernel_size=1),
nn.BatchNorm2d(pool_proj),
nn.ReLU(inplace=True)
)
def forward(self, x):
branch1 = self.branch1(x)
branch2 = self.branch2(x)
branch3 = self.branch3(x)
branch4 = self.branch4(x)
outputs = [branch1, branch2, branch3, branch4]
return torch.cat(outputs, 1)
class GoogLeNet(nn.Module):
def __init__(self):
super(GoogLeNet, self).__init__()
self.conv1 = nn.Conv2d(3, 64, kernel_size=3, padding=1)
self.bn1 = nn.BatchNorm2d(64)
self.inception1 = Inception(64, 64, 96, 128, 16, 32, 32)
self.inception2 = Inception(256, 128, 128, 192, 32, 96, 64)
self.maxpool = nn.MaxPool2d(kernel_size=3, stride=2, padding=1)
self.inception3 = Inception(480, 192, 96, 208, 16, 48, 64)
self.inception4 = Inception(512, 160, 112, 224, 24, 64, 64)
self.inception5 = Inception(512, 128, 128, 256, 24, 64, 64)
self.inception6 = Inception(512, 112, 144, 288, 32, 64, 64)
self.inception7 = Inception(528, 256, 160, 320, 32, 128, 128)
self.avgpool = nn.AdaptiveAvgPool2d((1, 1))
self.dropout = nn.Dropout(p=0.4)
self.fc = nn.Linear(832, 10)
def forward(self, x):
x = F.relu(self.bn1(self.conv1(x)))
x = self.maxpool(x)
x = self.inception1(x)
x = self.inception2(x)
x = self.maxpool(x)
x = self.inception3(x)
x = self.inception4(x)
x = self.inception5(x)
x = self.inception6(x)
x = self.inception7(x)
x = self.avgpool(x)
x = x.view(x.size(0), -1)
x = self.dropout(x)
x = self.fc(x)
return x
```
这个GoogLeNet模型可以通过在CIFAR-10数据集上进行训练来实现图像分类任务。
阅读全文
相关推荐
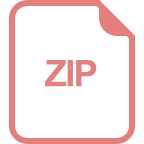
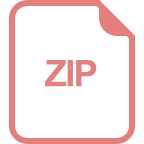
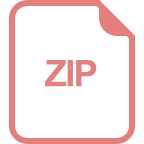
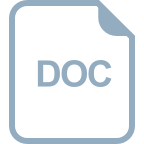












