prediction = dict() prediction["MultinomialNB"] = NB_model.predict(x_test_df)
时间: 2024-02-29 14:54:50 浏览: 19
你提供的代码使用MultinomialNB模型对象NB_model对测试数据x_test_df进行预测,即使用训练好的模型来预测测试数据的标签。预测的结果存储在一个名为prediction的字典对象中,其中"MultinomialNB"表示使用的模型名称,NB_model.predict(x_test_df)表示对测试数据进行预测的结果。
相关问题
建立贝叶斯模型 NB_model = MultinomialNB(alpha=0.1, class_prior=None, fit_prior=True) 1 贝叶斯模型训练 NB_model.fit(x_train_df,y_train) 1 贝叶斯模型保存 from sklearn.externals import joblib joblib.dump(NB_model, "nb_model.m") 1 2 贝叶斯模型预测 prediction = dict() prediction["MultinomialNB"] = NB_model.predict(x_test_df) 1 2 3 贝叶斯模型评估 from sklearn.metrics import accuracy_score,f1_score,confusion_matrix,classification_report print(classification_report(y_test,prediction["MultinomialNB"],target_names=["Ham","Spam"]))
你提供的代码实现了一个基于MultinomialNB算法的朴素贝叶斯分类器的建立、训练、保存、预测和评估。具体说明如下:
1. 建立贝叶斯模型:使用MultinomialNB类创建一个名为NB_model的贝叶斯模型对象,其中alpha=0.1表示平滑参数的值,class_prior=None表示使用统一的先验概率,fit_prior=True表示学习类先验概率。
2. 贝叶斯模型训练:使用训练数据x_train_df和标签y_train对贝叶斯模型进行训练,即使用这些数据来学习如何根据输入的特征来预测相应的输出标签。
3. 贝叶斯模型保存:使用joblib库的dump函数将训练好的贝叶斯模型保存在名为nb_model.m的文件中,以备后续使用。
4. 贝叶斯模型预测:使用训练好的贝叶斯模型对测试数据x_test_df进行预测,即使用模型来预测测试数据的标签,预测的结果存储在一个名为prediction的字典对象中,其中"MultinomialNB"表示使用的模型名称,NB_model.predict(x_test_df)表示对测试数据进行预测的结果。
5. 贝叶斯模型评估:使用sklearn.metrics库中的函数来计算贝叶斯模型的性能指标,包括准确率、F1值、混淆矩阵和分类报告。其中,classification_report函数计算并打印模型的分类报告,其中包括每个类别的精确度、召回率、F1值和支持数。y_test是测试数据的真实标签,prediction["MultinomialNB"]是使用MultinomialNB模型预测出的标签,target_names=["Ham","Spam"]表示两个类别的名称。
def generate_midi(generator, output_file, start_sequence): # 加载模型参数 generator.load_weights('weights.hdf5') # 计算音符和和弦的数量 notes = load_midi(start_sequence) pitchnames = sorted(set(notes)) n_vocab = len(set(notes)) # 准备输入序列 sequence_length = 100 note_to_int = dict((note, number) for number, note in enumerate(pitchnames)) network_input = [] for i in range(0, len(notes) - sequence_length, 1): sequence_in = notes[i:i + sequence_length] network_input.append([note_to_int[char] for char in sequence_in]) # 生成 MIDI 文件 start = np.random.randint(0, len(network_input)-1) int_to_note = dict((number, note) for number, note in enumerate(pitchnames)) pattern = network_input[start] prediction_output = [] for note_index in range(500): prediction_input = np.reshape(pattern, (1, len(pattern), 1)) prediction_input = prediction_input / float(n_vocab) prediction = generator.predict(prediction_input, verbose=0) index = np.argmax(prediction) result = int_to_note[index] prediction_output.append(result) pattern.append(index) pattern = pattern[1:len(pattern)] offset = 0 output_notes = [] # 创建音符和和弦对象 for pattern in prediction_output: # 如果是和弦 if ('.' in pattern) or pattern.isdigit(): notes_in_chord = pattern.split('.') notes = [] for current_note in notes_in_chord: new_note = note.Note(int(current_note)) new_note.storedInstrument = instrument.Piano() notes.append(new_note) new_chord = chord.Chord(notes) new_chord.offset = offset output_notes.append(new_chord) # 如果是音符 else: new_note = note.Note(pattern) new_note.offset = offset new_note.storedInstrument = instrument.Piano() output_notes.append(new_note) # 增加偏移量 offset += 0.5 # 创建 MIDI 流对象 midi_stream = stream.Stream(output_notes) # 保存 MIDI 文件 midi_stream.write('midi', fp=output_file)
这段代码是用来生成 MIDI 音乐的,其中使用了一个生成器模型来生成音乐。在生成 MIDI 音乐之前,先加载模型参数,并准备输入序列。接下来,从输入序列中随机选择一个起始点,然后使用生成器模型来预测下一个音符或和弦。生成的音符或和弦会被添加到预测输出列表中,同时也会更新当前输入序列,以便用于下一个预测。最后,将预测输出转换成音符和和弦对象,并将它们添加到 MIDI 流对象中,最终生成 MIDI 文件。
相关推荐
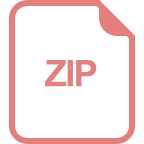
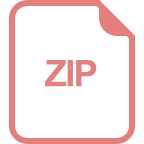












