attention maps
时间: 2023-09-07 22:15:29 浏览: 78
注意力图(attention maps)是在深度学习中常用的一种可视化工具,用于表示模型在处理输入数据时对不同位置的关注程度或权重分配。在许多任务中,特别是在自然语言处理(NLP)领域中,注意力机制被广泛应用于提高模型的性能和理解能力。
对于文本数据,注意力图能够显示模型在生成每个单词或字符时关注的输入单词或字符的位置。通过注意力图,我们可以直观地观察模型在生成输出时的决策过程,了解模型关注哪些部分的输入信息更多。
注意力图通常以矩阵的形式表示,其中每个元素表示模型在生成某个输出时对应输入位置的权重或关注度。这些权重可以通过不同的机制计算得到,例如,使用点积注意力、加性注意力等。
注意力图在机器翻译、文本摘要、问答系统等任务中被广泛使用,它不仅能够提高模型的性能,还可以帮助解释和理解模型的决策过程。
相关问题
Vgg16 attention
VGG16 is a convolutional neural network architecture that consists of 16 layers. Attention mechanisms can be added to this architecture to improve its performance in tasks that require focusing on specific regions or features of input images.
One way to add attention to VGG16 is to use spatial attention, which involves selectively weighting different regions of the image based on their importance for the task at hand. This can be achieved by adding an attention layer after the convolutional layers that computes a spatial attention map based on the input image. The attention map is then used to weight the output of the convolutional layers before passing it to the fully connected layers for classification.
Another way to add attention to VGG16 is to use channel attention, which involves selectively weighting different channels of the feature maps based on their importance for the task at hand. This can be achieved by adding a channel attention layer after each convolutional layer that computes a channel attention vector based on the feature maps. The channel attention vector is then used to weight the feature maps before passing them to the next convolutional layer. This helps to enhance the most important features and suppress irrelevant ones.
Overall, adding attention mechanisms to VGG16 can help improve its performance in tasks that require selective focus on specific regions or features of input images.
DenseNet-Attention
DenseNet-Attention is a variant of the DenseNet architecture that incorporates attention mechanisms to enhance feature selection and improve model performance. In this architecture, attention is applied to the feature maps produced by each dense block in the network.
In a standard DenseNet, each dense block concatenates the feature maps produced by all previous blocks, resulting in a large number of feature maps that can be computationally expensive to process. In DenseNet-Attention, attention mechanisms are used to selectively weight and combine the feature maps based on their importance to the task at hand.
There are several variations of DenseNet-Attention, but one common approach is to use channel attention and spatial attention. Channel attention weights each channel of the feature map based on its importance to the task, while spatial attention weights each spatial location of the feature map based on its importance to the task.
DenseNet-Attention has been shown to achieve state-of-the-art performance on a variety of computer vision tasks, including image classification, object detection, and semantic segmentation.
相关推荐
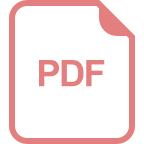
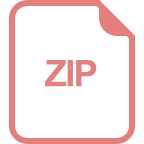
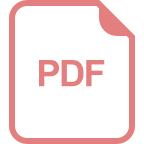












