解释def adaPoint(box, pro): box_pro = box if pro != 1.0: box_pro = box / pro box_pro = np.trunc(box_pro) return box_pro # 四边形顶点排序,[top-left, top-right, bottom-right, bottom-left] def orderPoints(pts): rect = np.zeros((4, 2), dtype="float32") s = pts.sum(axis=1) rect[0] = pts[np.argmin(s)] rect[2] = pts[np.argmax(s)] diff = np.diff(pts, axis=1) rect[1] = pts[np.argmin(diff)] rect[3] = pts[np.argmax(diff)] return rect # 计算长宽 def pointDistance(a, b): return int(np.sqrt(np.sum(np.square(a - b)))) # 透视变换 def warpImage(image, box): w, h = pointDistance(box[0], box[1]), pointDistance(box[1], box[2]) dst_rect = np.array([[0, 0], [w - 1, 0], [w - 1, h - 1], [0, h - 1]], dtype='float32') M = cv2.getPerspectiveTransform(box, dst_rect) warped = cv2.warpPerspective(image, M, (w, h)) return warped
时间: 2023-11-28 08:06:41 浏览: 163
这是一个用于透视变换的函数,主要包括以下几个步骤:
1. adaPoint函数:根据给定的比例pro,将四边形的顶点坐标进行适当的缩放,并将坐标转换为整数类型;
2. orderPoints函数:对四边形的顶点进行排序,使其按照[top-left, top-right, bottom-right, bottom-left]的顺序排列;
3. pointDistance函数:计算两个点之间的欧氏距离;
4. warpImage函数:通过透视变换将原始图像image转换为目标四边形box所确定的矩形区域内的图像。具体实现方法是:
(a) 计算目标矩形的宽w和高h,以及目标矩形的四个顶点坐标dst_rect;
(b) 使用cv2.getPerspectiveTransform函数计算从原始四边形box到目标矩形dst_rect的透视变换矩阵M;
(c) 使用cv2.warpPerspective函数对原始图像image进行透视变换,得到目标矩形内的图像warped。
透视变换常用于图像校正、文档扫描等领域。
相关问题
def __init__(self, mean: Sequence[Number] = None, std: Sequence[Number] = None, pad_size_divisor: int = 1, pad_value: Union[float, int] = 0, pad_mask: bool = False, mask_pad_value: int = 0, pad_seg: bool = False, seg_pad_value: int = 255, bgr_to_rgb: bool = False, rgb_to_bgr: bool = False, boxtype2tensor: bool = True, non_blocking: Optional[bool] = False, batch_augments: Optional[List[dict]] = None): super().__init__( mean=mean, std=std, pad_size_divisor=pad_size_divisor, pad_value=pad_value, bgr_to_rgb=bgr_to_rgb, rgb_to_bgr=rgb_to_bgr, non_blocking=non_blocking) if batch_augments is not None: self.batch_augments = nn.ModuleList( [MODELS.build(aug) for aug in batch_augments]) else: self.batch_augments = None self.pad_mask = pad_mask self.mask_pad_value = mask_pad_value self.pad_seg = pad_seg self.seg_pad_value = seg_pad_value self.boxtype2tensor = boxtype2tensor什么意思
这段代码是一个数据预处理器(DataPreprocessor)类的初始化方法(__init__),用于创建数据预处理器的实例。下面是对各个参数的解释:
- mean: 均值(mean)是一个数值序列,用于对图像进行均值减法。默认为 None。
- std: 标准差(std)是一个数值序列,用于对图像进行标准化处理。默认为 None。
- pad_size_divisor: pad_size_divisor 是一个整数,指定图像的尺寸除以该数的余数为 0,以便进行图像填充。默认为 1。
- pad_value: pad_value 是一个浮点数或整数,用于指定填充图像的像素值。默认为 0。
- pad_mask: pad_mask 是一个布尔值,指示是否对掩膜(mask)进行填充。默认为 False。
- mask_pad_value: mask_pad_value 是一个整数,用于指定填充掩膜的像素值。默认为 0。
- pad_seg: pad_seg 是一个布尔值,指示是否对分割图(segmentation)进行填充。默认为 False。
- seg_pad_value: seg_pad_value 是一个整数,用于指定填充分割图的像素值。默认为 255。
- bgr_to_rgb: bgr_to_rgb 是一个布尔值,指示是否将图像从 BGR 通道顺序转换为 RGB 通道顺序。默认为 False。
- rgb_to_bgr: rgb_to_bgr 是一个布尔值,指示是否将图像从 RGB 通道顺序转换为 BGR 通道顺序。默认为 False。
- boxtype2tensor: boxtype2tensor 是一个布尔值,指示是否将边界框的类型转换为张量。默认为 True。
- non_blocking: non_blocking 是一个可选的布尔值,指示是否以非阻塞方式加载数据。默认为 False。
- batch_augments: batch_augments 是一个可选的字典列表,用于定义批量增强操作。默认为 None。
在初始化方法中,首先调用父类的初始化方法(super().__init__)来设置均值、标准差、填充相关的参数和通道顺序转换的参数。然后根据传入的 batch_augments 参数,构建批量增强操作的模块列表,并将其保存到 self.batch_augments 属性中。最后,根据传入的参数设置是否进行掩膜和分割图的填充,以及是否将边界框类型转换为张量。
总之,这个初始化方法用于创建数据预处理器的实例,并设置相应的参数和属性,以便在数据预处理过程中进行图像的均值减法、标准化、填充、通道转换等操作,并支持批量增强和边界框类型转换。
total_obj_num_distance_dict = {10: 0, 20: 0, 50: 0, 100: 0, 'nocare': 0} tolerance_num_point = {0.015: 0, 0.03: 0, 0.07: 0, 0.10: 0, 1000: 0} tolerance_num_vanish_point = {0.015: 0, 0.03: 0, 0.07: 0, 0.10: 0, 1000: 0} tolerance_num_via_box = {0.015: 0, 0.03: 0, 0.07: 0, 0.10: 0, 1000: 0} tolerance_num_combine = {0.015: 0, 0.03: 0, 0.07: 0, 0.10: 0, 1000: 0} distance_error_sum_point = {0.015: 0, 0.03: 0, 0.07: 0, 0.10: 0, 1000: 0} distance_error_sum_vanish_point = {0.015: 0, 0.03: 0, 0.07: 0, 0.10: 0, 1000: 0} distance_error_sum_via_box = {0.015: 0, 0.03: 0, 0.07: 0, 0.10: 0, 1000: 0} distance_error_sum_combine = {0.015: 0, 0.03: 0, 0.07: 0, 0.10: 0, 1000: 0}整理代码
可以使用字典的 `items()` 方法来遍历字典的键值对,然后按照需要的格式整理代码。以下是一个示例代码:
```python
def format_dict_code(dictionary):
code = ""
for key, value in dictionary.items():
code += f"{key}: {value}, "
code = "{" + code.rstrip(", ") + "}"
return code
total_obj_num_distance_dict_code = format_dict_code(total_obj_num_distance_dict)
tolerance_num_point_code = format_dict_code(tolerance_num_point)
tolerance_num_vanish_point_code = format_dict_code(tolerance_num_vanish_point)
tolerance_num_via_box_code = format_dict_code(tolerance_num_via_box)
tolerance_num_combine_code = format_dict_code(tolerance_num_combine)
distance_error_sum_point_code = format_dict_code(distance_error_sum_point)
distance_error_sum_vanish_point_code = format_dict_code(distance_error_sum_vanish_point)
distance_error_sum_via_box_code = format_dict_code(distance_error_sum_via_box)
distance_error_sum_combine_code = format_dict_code(distance_error_sum_combine)
print(f"total_obj_num_distance_dict = {total_obj_num_distance_dict_code}")
print(f"tolerance_num_point = {tolerance_num_point_code}")
print(f"tolerance_num_vanish_point = {tolerance_num_vanish_point_code}")
print(f"tolerance_num_via_box = {tolerance_num_via_box_code}")
print(f"tolerance_num_combine = {tolerance_num_combine_code}")
print(f"distance_error_sum_point = {distance_error_sum_point_code}")
print(f"distance_error_sum_vanish_point = {distance_error_sum_vanish_point_code}")
print(f"distance_error_sum_via_box = {distance_error_sum_via_box_code}")
print(f"distance_error_sum_combine = {distance_error_sum_combine_code}")
```
这段代码将会输出整理好的代码,你可以根据需要将其复制到你的程序中。
阅读全文
相关推荐
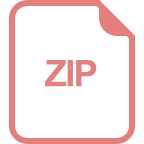
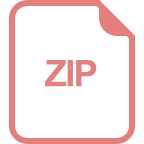
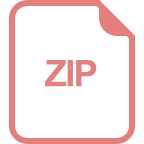














