_, x = np.nonzero(np.bitwise_not(mask))
时间: 2023-12-03 09:02:38 浏览: 91
这行代码的作用是找到二进制掩码中为 0 的位置,并将这些位置保存在变量 x 中。具体来说,它首先使用 np.bitwise_not 函数将掩码中的 1 和 0 取反,即将为 0 的位置变为 1,为 1 的位置变为 0,然后使用 np.nonzero 函数找到所有非零元素的位置,也就是 1 的位置,最后再将这些位置保存在变量 x 中。这个操作通常用于对数组进行遮罩处理,从而实现只对某些位置进行操作或者排除某些位置的目的。
相关问题
import numpy as np import pandas as pd def read_asc(filepath): asc_file = pd.read_csv(filepath, skiprows=4, encoding="gbk", engine='python', sep=' ', delimiter=None, index_col=False, header=None, skipinitialspace=True) file = np.array(asc_file) data = read_message(file) filter_step_size(data) def read_message(file): mask = file[:, 4] == "107" data = file[mask] return data def filter_step_size(data): diff = np.diff(data[:, 0]) mask = np.logical_and(0.090 < diff, diff < 0.110) success_sum = np.count_nonzero(mask) fail_sum = len(mask) - success_sum result = np.column_stack((data[:-1, 0], data[1:, 0], diff)) result = result[~mask] print("步长通过数: {}".format(success_sum)) print("步长未通过数: {}".format(fail_sum)) print("未通过前一项值:未通过后一项值:差值:") print(result) if __name__ == '__main__': read_asc("E:\package\databin 7-11-2023 9-12-31 am Messages File.asc") 这段代码在运行时报错”Unable to allocate 1.63 GiB for an array with shape (4475770, 49) and data type object“,怎么修改优化
这个错误是由于 NumPy 数组中存在大量的对象类型(`object`)数据导致的。对象类型的数据通常需要更多的内存空间来存储,因此可能会导致内存不足的错误。
为了解决这个问题,你可以尝试以下优化措施:
1. 检查数据类型:查看你的数据文件中是否有非数值类型的列。如果有,可以尝试将其转换为适当的数值类型(如整数、浮点数等)来减少内存使用。
2. 限制读取的列数:在 `read_asc` 函数中,你可以尝试只读取需要使用的列,而不是将整个文件都读入内存。这样可以减少内存占用。
3. 分块读取数据:如果数据文件太大,无法一次性读入内存,可以考虑使用 Pandas 的 `read_csv` 函数的 `chunksize` 参数来分块读取数据,并在每一块中进行处理。
下面是修改后的代码示例:
```python
import numpy as np
import pandas as pd
def read_asc(filepath):
usecols = [0, 4] # 仅读取第0列和第4列
chunksize = 100000 # 每次读取100000行数据
for chunk in pd.read_csv(filepath, skiprows=4, encoding="gbk", engine='python', sep=' ', delimiter=None,
index_col=False, header=None, skipinitialspace=True, usecols=usecols, chunksize=chunksize):
file = np.array(chunk)
data = read_message(file)
filter_step_size(data)
def read_message(file):
mask = file[:, 1] == "107"
data = file[mask]
return data
def filter_step_size(data):
diff = np.diff(data[:, 0].astype(float)) # 将第0列转换为浮点数类型
mask = np.logical_and(0.090 < diff, diff < 0.110)
success_sum = np.count_nonzero(mask)
fail_sum = len(mask) - success_sum
result = np.column_stack((data[:-1, 0], data[1:, 0], diff))
result = result[~mask]
print("步长通过数: {}".format(success_sum))
print("步长未通过数: {}".format(fail_sum))
print("未通过前一项值:未通过后一项值:差值:")
print(result)
if __name__ == '__main__':
read_asc("E:\package\databin 7-11-2023 9-12-31 am Messages File.asc")
```
通过以上优化,我们限制了读取的列数,使用了分块读取数据的方式,并将第0列转换为浮点数类型,以减少内存占用。希望这能够解决你遇到的问题。如果问题仍然存在,请提供更多关于数据文件的信息,以便我能够给出更准确的解决方案。
解释代码features_poly = poly_transformer.get_feature_names(features) features_nonzero = np.array(features_poly)[lr.coef_ != 0] coef_nonzero = lr.coef_[lr.coef_ != 0]
- `poly_transformer.get_feature_names(features)`:这行代码使用 `poly_transformer` 对象对输入特征 `features` 进行多项式转换,并返回转换后的特征名称列表。
- `features_nonzero = np.array(features_poly)[lr.coef_ != 0]`:这行代码创建 `features_nonzero` 数组,其中包含了那些与非零系数相关的多项式特征。`np.array(features_poly)` 将多项式特征名称列表转换为 NumPy 数组,然后 `lr.coef_ != 0` 返回了一个大小与回归模型系数数组相同的布尔数组,指示哪些系数不为零。通过将这个布尔数组作为索引应用于特征名称数组,我们得到了与非零系数相关的特征名称数组。
- `coef_nonzero = lr.coef_[lr.coef_ != 0]`:这行代码创建 `coef_nonzero` 数组,其中包含了那些非零系数的回归模型系数。与上一行代码类似,`lr.coef_ != 0` 返回了一个布尔数组,其中指示哪些系数不为零。通过将这个布尔数组作为索引应用于 `lr.coef_`,我们得到了一个包含非零系数的数组 `coef_nonzero`,其大小与 `features_nonzero` 相同。
阅读全文
相关推荐
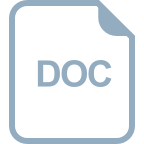
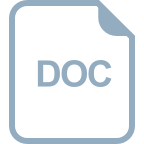
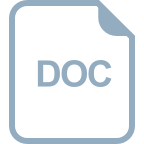











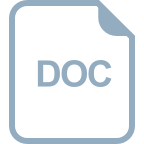
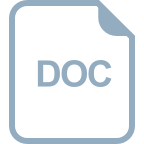