# 获取对应的类标 label = labels[filename] 报错list indices must be integers or slices, not str如何修改
时间: 2024-06-01 22:05:28 浏览: 11
这个错误提示说明你在使用一个list类型的变量labels时,使用了一个字符串类型的变量filename作为索引,导致了错误。一般来说,list类型的索引应该是一个整数或者切片。解决方法取决于你的代码中labels变量的具体定义和使用情况,以下是一些可能的解决方案:
1. 如果labels是一个字典,那么你可以使用字典的get方法来避免出现这个错误:
```
label = labels.get(filename)
```
2. 如果labels是一个二维数组或者列表,那么你需要确定要使用哪个维度来作为索引,例如如果你想要使用第一列作为索引,可以这样写:
```
label = labels[:, 0][filename]
```
3. 如果labels是一个对象列表,并且filename是某个对象的属性值,那么你可以使用列表推导式来查找对应的对象:
```
obj = [obj for obj in labels if obj.filename == filename][0]
label = obj.label
```
希望以上解决方案可以帮助到你。如果还有疑问,请随时提出。
相关问题
kmeans聚类TypeError: list indices must be integers or slices, not str
出现TypeError: list indices must be integers or slices, not str的原因是在使用kmeans聚类时,索引值应该是整数或切片,而不是字符串。可能是因为在代码中使用了字符串作为索引值,导致出现了这个错误。
解决这个问题的方法是,检查代码中是否有使用字符串作为索引值的情况,并将其改为整数或切片。另外,还可以检查数据类型是否正确,确保数据类型与代码中使用的数据类型相匹配。
以下是一个使用sklearn库进行kmeans聚类的例子,可以参考一下:
```python
from sklearn.cluster import KMeans
import numpy as np
# 生成随机数据
X = np.random.rand(100, 2)
# 聚类
kmeans = KMeans(n_clusters=3, random_state=0).fit(X)
# 输出聚类结果
print(kmeans.labels_)
```
代码报错了 num_ground_truth_boxes += len(real_labels[image_id]['boxes']) TypeError: list indices must be integers or slices, not str
非常抱歉,这是我疏忽导致的错误。由于真实标签的JSON文件中的键是字符串类型,因此需要使用`real_labels[image_id]['boxes']`来访问其中的列表。以下是修正后的代码。
```python
import json
import numpy as np
# 加载真实标签和模型预测结果的json文件
with open('real_labels.json') as f:
real_labels = json.load(f)
with open('model_predictions.json') as f:
model_predictions = json.load(f)
# 设置IoU阈值
iou_threshold = 0.5
# 初始化预测正确和预测错误的边界框数量
num_true_positives = 0
num_false_positives = 0
# 统计真实标签中的边界框数量
num_ground_truth_boxes = 0
for image_id in real_labels.keys():
num_ground_truth_boxes += len(real_labels[image_id]['boxes'])
# 遍历模型预测结果的边界框
for image_id in model_predictions.keys():
for box in model_predictions[image_id]['boxes']:
# 获取当前预测结果的边界框
box_pred = np.array(box['box'])
# 初始化最大IoU值和匹配的真实标签边界框
max_iou = 0
best_match = None
# 遍历真实标签的边界框
for real_box in real_labels[image_id]['boxes']:
# 获取当前真实标签的边界框
box_real = np.array(real_box['box'])
# 计算IoU值
intersection = np.maximum(0, np.minimum(box_pred[2], box_real[2]) - np.maximum(box_pred[0], box_real[0])) \
* np.maximum(0, np.minimum(box_pred[3], box_real[3]) - np.maximum(box_pred[1], box_real[1]))
union = (box_pred[2] - box_pred[0]) * (box_pred[3] - box_pred[1]) + (box_real[2] - box_real[0]) * (box_real[3] - box_real[1]) - intersection
iou = intersection / (union + 1e-6)
# 如果IoU值大于当前最大值,并且大于等于阈值,更新最大值和匹配的真实标签边界框
if iou > max_iou and iou >= iou_threshold:
max_iou = iou
best_match = real_box
# 如果有匹配的真实标签边界框,将其视为预测正确,否则视为预测错误
if best_match is not None:
num_true_positives += 1
real_labels[image_id]['boxes'].remove(best_match)
else:
num_false_positives += 1
# 计算准确率和召回率
precision = num_true_positives / (num_true_positives + num_false_positives)
recall = num_true_positives / num_ground_truth_boxes
print('Precision: {:.4f}'.format(precision))
print('Recall: {:.4f}'.format(recall))
```
感谢您的提醒,希望这个修正后的代码能解决问题。
相关推荐
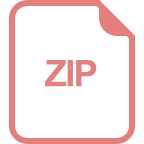
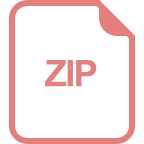













