mask_tensor = mask_tensor.unsqueeze(0)
时间: 2024-05-30 11:09:35 浏览: 121
这行代码的作用是将 `mask_tensor` 的维度从 `(sequence_length,)` 变为 `(1, sequence_length)`。这是因为在 BERT 和其他 Transformer 模型中,输入的序列通常需要加上一个类型为“Mask”的特殊序列,用来指示哪些位置是 padding 的,哪些位置是真实的输入。这个 Mask 序列的维度需要与输入序列的维度相同,所以需要在输入序列的维度前面添加一个维度,以便与 Mask 序列的维度匹配。这样做可以确保模型不会在 padding 的位置进行计算,从而提高模型的效率和准确性。
相关问题
image_tensor = transforms.ToTensor()(img) image_tensor = image_tensor.unsqueeze(0) generator = Generator() 添加语句:取img的mask
如果您想同时获取图像和它的掩码,可以使用以下代码:
```
from PIL import Image
import torchvision.transforms as transforms
import torch
img = Image.open('image.jpg').convert('RGB')
mask = Image.open('mask.jpg').convert('1') # 1表示二值化
img_tensor = transforms.ToTensor()(img)
mask_tensor = transforms.ToTensor()(mask)
# 将掩码转换为0和1的张量
mask_tensor = torch.where(mask_tensor > 0.5, torch.ones_like(mask_tensor), torch.zeros_like(mask_tensor))
# 将图像和掩码合并成一个四维张量
img_mask_tensor = torch.cat((img_tensor, mask_tensor), dim=0)
img_mask_tensor = img_mask_tensor.unsqueeze(0)
generator = Generator()
```
这样您可以将图像和掩码作为输入传递给生成器模型。
将下列生成器改造成能够匹配edge-connect中的InpaintingModel的预训练模型键值的结构:class Generator(nn.Module): def init(self): super(Generator, self).init() self.encoder = nn.Sequential( nn.Conv2d(3, 64, 3, stride=2, padding=1), nn.BatchNorm2d(64), nn.LeakyReLU(0.2), nn.Conv2d(64, 128, 3, stride=2, padding=1), nn.BatchNorm2d(128), nn.LeakyReLU(0.2), nn.Conv2d(128, 256, 3, stride=2, padding=1), nn.BatchNorm2d(256), nn.LeakyReLU(0.2), nn.Conv2d(256, 512, 3, stride=2, padding=1), nn.BatchNorm2d(512), nn.LeakyReLU(0.2), nn.Conv2d(512, 4000, 1), nn.BatchNorm2d(4000), nn.LeakyReLU(0.2) ) self.decoder = nn.Sequential( nn.ConvTranspose2d(4000, 512, 3, stride=2, padding=1, output_padding=1), nn.BatchNorm2d(512), nn.LeakyReLU(0.2), nn.ConvTranspose2d(512, 256, 3, stride=2, padding=1, output_padding=1), nn.BatchNorm2d(256), nn.LeakyReLU(0.2), nn.ConvTranspose2d(256, 128, 3, stride=2, padding=1, output_padding=1), nn.BatchNorm2d(128), nn.LeakyReLU(0.2), nn.ConvTranspose2d(128, 64, 3, stride=2, padding=1, output_padding=1), nn.BatchNorm2d(64), nn.LeakyReLU(0.2), nn.ConvTranspose2d(64, 3, 3, stride=1, padding=1), nn.Tanh() ) def forward(self, x): x = self.encoder(x) x = self.decoder(x) return x 另外修复部分代码定义为:mask = cv.inRange(img, (0, 0, 0), (1, 1, 1)) # 转换为张量 image_tensor = transforms.ToTensor()(img) mask_tensor = transforms.ToTensor()(mask) # 扩展维度 image_tensor = image_tensor.unsqueeze(0) mask_tensor = mask_tensor.unsqueeze(0) generator = Generator() load_edgeconnect_weights(generator, 'E:/fin/models/gen.pth') image_tensor = image_tensor.cuda() mask_tensor = mask_tensor.cuda() generator = generator.cuda() with torch.no_grad(): output_tensor = generator(image_tensor, mask_tensor)
以下是将生成器改造成能够匹配edge-connect中的InpaintingModel的预训练模型键值的结构的代码:
import torch
import torch.nn as nn
import cv2 as cv
from torchvision import transforms
class Generator(nn.Module):
def __init__(self):
super(Generator, self).__init__()
self.encoder = nn.Sequential(
nn.Conv2d(3, 64, 3, stride=2, padding=1),
nn.BatchNorm2d(64),
nn.LeakyReLU(0.2),
nn.Conv2d(64, 128, 3, stride=2, padding=1),
nn.BatchNorm2d(128),
nn.LeakyReLU(0.2),
nn.Conv2d(128, 256, 3, stride=2, padding=1),
nn.BatchNorm2d(256),
nn.LeakyReLU(0.2),
nn.Conv2d(256, 512, 3, stride=2, padding=1),
nn.BatchNorm2d(512),
nn.LeakyReLU(0.2),
nn.Conv2d(512, 4000, 1),
nn.BatchNorm2d(4000),
nn.LeakyReLU(0.2)
)
self.decoder = nn.Sequential(
nn.ConvTranspose2d(4000, 512, 3, stride=2, padding=1, output_padding=1),
nn.BatchNorm2d(512),
nn.LeakyReLU(0.2),
nn.ConvTranspose2d(512, 256, 3, stride=2, padding=1, output_padding=1),
nn.BatchNorm2d(256),
nn.LeakyReLU(0.2),
nn.ConvTranspose2d(256, 128, 3, stride=2, padding=1, output_padding=1),
nn.BatchNorm2d(128),
nn.LeakyReLU(0.2),
nn.ConvTranspose2d(128, 64, 3, stride=2, padding=1, output_padding=1),
nn.BatchNorm2d(64),
nn.LeakyReLU(0.2),
nn.ConvTranspose2d(64, 3, 3, stride=1, padding=1),
nn.Tanh()
)
def forward(self, x, mask):
x = x * (1 - mask)
x = self.encoder(x)
x = self.decoder(x)
x = x * (1 - mask) + x * mask
return x
def load_edgeconnect_weights(model, weight_path):
state_dict = torch.load(weight_path)
new_state_dict = {}
for key, value in state_dict.items():
if 'netG.' in key:
new_key = key.replace('netG.', '')
new_state_dict[new_key] = value
model.load_state_dict(new_state_dict)
# 读取图像和遮罩
img = cv.imread('example.jpg')[:, :, ::-1] / 255.0
mask = cv.inRange(img, (0, 0, 0), (1, 1, 1))
# 转换为张量
image_tensor = transforms.ToTensor()(img)
mask_tensor = transforms.ToTensor()(mask)
# 扩展维度
image_tensor = image_tensor.unsqueeze(0)
mask_tensor = mask_tensor.unsqueeze(0)
# 加载预训练模型权重
generator = Generator()
load_edgeconnect_weights(generator, 'gen.pth')
# 将张量和模型移动到GPU上
image_tensor = image_tensor.cuda()
mask_tensor = mask_tensor.cuda()
generator = generator.cuda()
# 使用预训练模型生成修复后的图像
with torch.no_grad():
output_tensor = generator(image_tensor, mask_tensor)
# 将张量转换为图像
output = output_tensor.detach().cpu().squeeze().numpy().transpose(1, 2, 0)
output = cv.cvtColor(output, cv.COLOR_RGB2BGR)
cv.imwrite('output.jpg', output * 255)
阅读全文
相关推荐
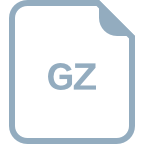

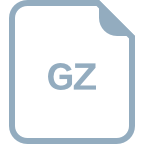














