pycolmap.IncrementalMapperOptions()
时间: 2023-06-21 22:04:18 浏览: 308
`pycolmap.IncrementalMapperOptions()` 是用于设置 Colmap 中的增量式三维重建的选项的类。它包含了一些参数,可以通过设置这些参数来调整增量式三维重建的行为,例如设置图像匹配算法、三维点云筛选阈值等。以下是一些常用的参数:
- `min_num_matches`:最小匹配数,用于筛选匹配数量过少的图像。
- `min_track_length`:最小轨迹长度,用于筛选轨迹长度过短的点。
- `retriangulation_enabled`:是否启用重投影三角化,用于增量式重建中的点云筛选。
- `retriangulation_max_reproj_error`:重投影误差阈值,用于筛选重投影误差过大的点。
使用时,可以创建一个 `pycolmap.IncrementalMapperOptions()` 对象,并通过设置其属性来调整参数。例如:
```
options = pycolmap.IncrementalMapperOptions()
options.min_num_matches = 30
options.min_track_length = 2
options.retriangulation_enabled = True
options.retriangulation_max_reproj_error = 4.0
```
然后将该对象传递给 `pycolmap.IncrementalMapper()` 函数以启动增量式重建。
相关问题
mapper_options = pycolmap.IncrementalMapperOptions() mapper_options.extract_colors = False mapper_options.min_model_size = 3
这段代码是使用pycolmap库中的IncrementalMapperOptions类创建一个对象mapper_options。其中,extract_colors属性被设置为False,表示在执行增量式重建时不提取颜色信息。min_model_size属性被设置为3,表示只有当模型中至少有3个点时才进行增量式重建。这些选项可以根据需要进行修改。
详细解释一下这段代码,每一句都要进行注解:tgt = f'/kaggle/working/{dataset}-{scene}' # Generate a simple reconstruction with SIFT (https://en.wikipedia.org/wiki/Scale-invariant_feature_transform). if not os.path.isdir(tgt): os.makedirs(f'{tgt}/bundle') os.system(f'cp -r {src}/images {tgt}/images') database_path = f'{tgt}/database.db' sift_opt = pycolmap.SiftExtractionOptions() sift_opt.max_image_size = 1500 # Extract features at low resolution could significantly reduce the overall accuracy sift_opt.max_num_features = 8192 # Generally more features is better, even if behond a certain number it doesn't help incresing accuracy sift_opt.upright = True # rotation invariance device = 'cpu' t = time() pycolmap.extract_features(database_path, f'{tgt}/images', sift_options=sift_opt, verbose=True) print(len(os.listdir(f'{tgt}/images'))) print('TIMINGS --- Feature extraction', time() - t) t = time() matching_opt = pycolmap.SiftMatchingOptions() matching_opt.max_ratio = 0.85 # Ratio threshold significantly influence the performance of the feature extraction method. It varies depending on the local feature but also on the image type # matching_opt.max_distance = 0.7 matching_opt.cross_check = True matching_opt.max_error = 1.0 # The ransac error threshold could help to exclude less accurate tie points pycolmap.match_exhaustive(database_path, sift_options=matching_opt, device=device, verbose=True) print('TIMINGS --- Feature matching', time() - t) t = time() mapper_options = pycolmap.IncrementalMapperOptions() mapper_options.extract_colors = False mapper_options.min_model_size = 3 # Sometimes you want to impose the first image pair for initialize the incremental reconstruction mapper_options.init_image_id1 = -1 mapper_options.init_image_id2 = -1 # Choose which interior will be refined during BA mapper_options.ba_refine_focal_length = True mapper_options.ba_refine_principal_point = True mapper_options.ba_refine_extra_params = True maps = pycolmap.incremental_mapping(database_path=database_path, image_path=f'{tgt}/images', output_path=f'{tgt}/bundle', options=mapper_options) print('TIMINGS --- Mapping', time() - t)
这段代码主要是使用 PyCOLMAP 库实现对图像的特征提取、特征匹配和增量式三维重建。具体解释如下:
```tgt = f'/kaggle/working/{dataset}-{scene}'```
定义了一个字符串变量 tgt,表示输出路径。
```if not os.path.isdir(tgt):```
如果输出路径不存在,则创建该路径。
```os.makedirs(f'{tgt}/bundle')```
在输出路径下创建子目录 bundle。
```os.system(f'cp -r {src}/images {tgt}/images')```
将源目录 src 中的 images 目录复制到输出路径下的 images 目录中。
```database_path = f'{tgt}/database.db'```
定义一个字符串变量 database_path,表示 PyCOLMAP 库中使用的数据库文件路径。
```sift_opt = pycolmap.SiftExtractionOptions()```
创建一个 SIFT 特征提取选项对象。
```sift_opt.max_image_size = 1500```
设置 SIFT 特征提取选项对象的最大图像尺寸为 1500×1500 像素。
```sift_opt.max_num_features = 8192```
设置 SIFT 特征提取选项对象的最大特征点数为 8192 个。
```sift_opt.upright = True```
设置 SIFT 特征提取选项对象的旋转不变性为 True,即不考虑图像旋转。
```device = 'cpu'```
定义一个字符串变量 device,表示计算设备类型。
```pycolmap.extract_features(database_path, f'{tgt}/images', sift_options=sift_opt, verbose=True)```
调用 PyCOLMAP 库中的 extract_features 函数,对输出路径下的图像进行 SIFT 特征提取,并将特征保存到数据库文件中。
```print(len(os.listdir(f'{tgt}/images')))```
输出输出路径下的图像数量。
```print('TIMINGS --- Feature extraction', time() - t)```
输出特征提取所花费的时间。
```matching_opt = pycolmap.SiftMatchingOptions()```
创建一个 SIFT 特征匹配选项对象。
```matching_opt.max_ratio = 0.85```
设置 SIFT 特征匹配选项对象的最大匹配比率为 0.85。
```matching_opt.max_distance = 0.7```
设置 SIFT 特征匹配选项对象的最大匹配距离为 0.7。
```matching_opt.cross_check = True```
设置 SIFT 特征匹配选项对象的交叉匹配为 True,即同时匹配两幅图像。
```matching_opt.max_error = 1.0```
设置 SIFT 特征匹配选项对象的最大误差为 1.0。
```pycolmap.match_exhaustive(database_path, sift_options=matching_opt, device=device, verbose=True)```
调用 PyCOLMAP 库中的 match_exhaustive 函数,对数据库文件中的特征进行 SIFT 特征匹配,并将匹配结果保存到数据库文件中。
```print('TIMINGS --- Feature matching', time() - t)```
输出特征匹配所花费的时间。
```mapper_options = pycolmap.IncrementalMapperOptions()```
创建一个增量式三维重建选项对象。
```mapper_options.extract_colors = False```
设置增量式三维重建选项对象的颜色提取为 False,即不提取图像颜色信息。
```mapper_options.min_model_size = 3```
设置增量式三维重建选项对象的最小模型大小为 3。
```mapper_options.init_image_id1 = -1```
设置增量式三维重建选项对象的第一张图像的 ID 为 -1,表示不指定。
```mapper_options.init_image_id2 = -1```
设置增量式三维重建选项对象的第二张图像的 ID 为 -1,表示不指定。
```mapper_options.ba_refine_focal_length = True```
设置增量式三维重建选项对象的相机内参的优化为 True。
```mapper_options.ba_refine_principal_point = True```
设置增量式三维重建选项对象的相机主点的优化为 True。
```mapper_options.ba_refine_extra_params = True```
设置增量式三维重建选项对象的额外参数的优化为 True。
```maps = pycolmap.incremental_mapping(database_path=database_path, image_path=f'{tgt}/images', output_path=f'{tgt}/bundle', options=mapper_options)```
调用 PyCOLMAP 库中的 incremental_mapping 函数,对数据库文件中的匹配结果进行增量式三维重建,并将重建结果保存到输出路径下的 bundle 目录中。
```print('TIMINGS --- Mapping', time() - t)```
输出增量式三维重建所花费的时间。
阅读全文
相关推荐
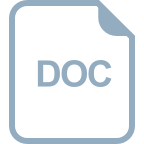















