请解释以下代码 class MeanShift(nn.Conv2d): def __init__( self, rgb_range, rgb_mean=(0.4488, 0.4371, 0.4040), rgb_std=(1.0, 1.0, 1.0), sign=-1): super(MeanShift, self).__init__(3, 3, kernel_size=1) std = torch.Tensor(rgb_std) self.weight.data = torch.eye(3).view(3, 3, 1, 1) / std.view(3, 1, 1, 1) self.bias.data = sign * rgb_range * torch.Tensor(rgb_mean) / std for p in self.parameters(): p.requires_grad = False
时间: 2023-03-08 16:17:20 浏览: 109
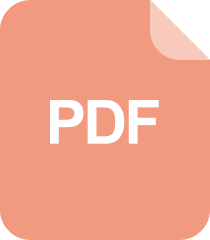
TensorFlow tf.nn.conv2d_transpose是怎样实现反卷积的
这段代码的功能是实现 MeanShift 算法,它是一种非参数估计技术,用于估计均值和标准差,并应用于图像处理等领域中。它使用一个3x3的卷积核,根据输入的rgb_mean和rgb_std,计算出权重和偏置,最终将像素调整到0附近,实现均值归一化。
阅读全文
相关推荐
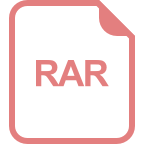
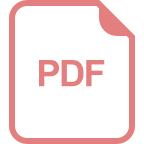















