FeatureAffine2D* feature_affine = new FeatureAffine2D(subset_radius_x, subset_radius_y, cpu_thread_number);
时间: 2024-09-13 13:14:56 浏览: 49
`FeatureAffine2D` 是 OpenCV 中的一个类,它用于估计图像序列之间的仿射(线性)光流,即两个连续帧之间像素点的运动。这段代码创建了一个新的 `FeatureAffine2D` 对象,参数包括:
- `subset_radius_x`: 估计光流时使用的子区域半径(在x方向上),通常用来限制搜索范围以提高计算效率。
- `subset_radius_y`: 类似的,在y方向上的子区域半径。
- `cpu_thread_number`: 指定用于并行计算的CPU线程数量。
具体初始化代码示例如下:
```cpp
// 创建 FeatureAffine2D 对象
cv::FeatureAffine2D feature_affine(subset_radius_x, subset_radius_y, cpu_thread_number);
// 使用该对象对两帧图像进行仿射光流估计
cv::Mat opticalFlow; // 假设这是要处理的两帧图像
feature_affine.compute(prevFrame, nextFrame, opticalFlow); // prevFrame 和 nextFrame 分别代表前一帧和当前帧
```
这里假设 `prevFrame` 和 `nextFrame` 是输入的图像矩阵,`opticalFlow` 会存储估计得到的光流信息。
相关问题
解释这段代码:int8_t rx = input[0 * point_cnt + a]; int8_t ry = input[1 * point_cnt + a]; int8_t rw = input[2 * point_cnt + a]; int8_t rh = input[3 * point_cnt + a]; float box_x = sigmoid(deqnt_affine_to_f32(rx, zp, scale)) * 2.0 - 0.5; float box_y = sigmoid(deqnt_affine_to_f32(ry, zp, scale)) * 2.0 - 0.5; float box_w = sigmoid(deqnt_affine_to_f32(rw, zp, scale)) * 2.0; float box_h = sigmoid(deqnt_affine_to_f32(rh, zp, scale)) * 2.0;
这段代码用于将输入的检测框坐标进行处理,从 int8_t 类型转换为 float 类型,并进行 sigmoid 反函数的计算。具体解释如下:
1. `rx`、`ry`、`rw`、`rh` 分别表示输入数组 `input` 中的四个元素,通过索引计算得到。
2. 将 `rx`、`ry`、`rw`、`rh` 分别作为参数传入 `deqnt_affine_to_f32` 函数进行逆量化操作,并转换为 float 类型的数值。
3. 对 `box_x` 和 `box_y` 进行 sigmoid 反函数的计算,将结果乘以 2.0 并减去 0.5,将值映射到 (-0.5, 1.5) 的区间内。
4. 对 `box_w` 和 `box_h` 进行 sigmoid 反函数的计算,将结果乘以 2.0,将值映射到 (0, 2.0) 的区间内。
通过这样的处理,可以将输入的 int8_t 类型的检测框坐标转换为 float 类型,并进行逆量化和反函数的计算,得到实际的检测框位置和大小。
解释代码:static int process(int8_t* input, int* anchor, int grid_h, int grid_w, int height, int width, int stride, std::vector<float>& boxes, std::vector<float>& objProbs, std::vector<int>& classId, float threshold, int32_t zp, float scale) { int validCount = 0; int grid_len = grid_h * grid_w; float thres = unsigmoid(threshold); int8_t thres_i8 = qnt_f32_to_affine(thres, zp, scale); for (int a = 0; a < 3; a++) { for (int i = 0; i < grid_h; i++) { for (int j = 0; j < grid_w; j++) { int8_t box_confidence = input[(PROP_BOX_SIZE * a + 4) * grid_len + i * grid_w + j]; if (box_confidence >= thres_i8) { int offset = (PROP_BOX_SIZE * a) * grid_len + i * grid_w + j; int8_t* in_ptr = input + offset; float box_x = sigmoid(deqnt_affine_to_f32(*in_ptr, zp, scale)) * 2.0 - 0.5; float box_y = sigmoid(deqnt_affine_to_f32(in_ptr[grid_len], zp, scale)) * 2.0 - 0.5; float box_w = sigmoid(deqnt_affine_to_f32(in_ptr[2 * grid_len], zp, scale)) * 2.0; float box_h = sigmoid(deqnt_affine_to_f32(in_ptr[3 * grid_len], zp, scale)) * 2.0; box_x = (box_x + j) * (float)stride; box_y = (box_y + i) * (float)stride; box_w = box_w * box_w * (float)anchor[a * 2]; box_h = box_h * box_h * (float)anchor[a * 2 + 1]; box_x -= (box_w / 2.0); box_y -= (box_h / 2.0); int8_t maxClassProbs = in_ptr[5 * grid_len]; int maxClassId = 0; for (int k = 1; k < OBJ_CLASS_NUM; ++k) { int8_t prob = in_ptr[(5 + k) * grid_len]; if (prob > maxClassProbs) { maxClassId = k; maxClassProbs = prob; } } if (maxClassProbs>thres_i8){ objProbs.push_back(sigmoid(deqnt_affine_to_f32(maxClassProbs, zp, scale))* sigmoid(deqnt_affine_to_f32(box_confidence, zp, scale))); classId.push_back(maxClassId); validCount++; boxes.push_back(box_x); boxes.push_back(box_y); boxes.push_back(box_w); boxes.push_back(box_h); } } } } } return validCount; }
这段代码实现了一个目标检测的后处理函数,与之前的代码相比,增加了对目标置信度和类别概率的判断。具体解释如下:
函数参数和功能与之前的代码相同,不再重复解释。
修改部分:
1. 在检测框的置信度 `box_confidence` 大于等于阈值 `thres_i8` 的条件下,再对目标置信度 `maxClassProbs` 进行判断。
2. 如果 `maxClassProbs` 大于阈值 `thres_i8`,则表示该检测框是一个有效的目标。
3. 将经过 sigmoid 函数转换后的目标置信度和检测框置信度相乘,作为最终的目标置信度。
4. 将最终的目标置信度、类别编号和有效目标计数记录到对应的向量中。
5. 将检测框的位置和大小添加到 `boxes` 向量中。
通过这样的修改,可以实现在进行目标检测后处理时,根据目标置信度和类别概率的阈值进行筛选,将有效的目标的位置、大小、置信度和类别信息存储在相应的向量中。
阅读全文
相关推荐


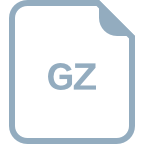














