concat2 = torch.cat([convt1,conv4],dim=1)
时间: 2024-05-19 07:13:20 浏览: 139
This line of code uses the PyTorch function `torch.cat()` to concatenate two tensors along dimension 1. The tensors being concatenated are `convt1` and `conv4`.
The resulting tensor, `concat2`, will have the same shape as `convt1` and `conv4`, except that their sizes along dimension 1 will be added together.
For example, if `convt1` has shape `(3, 64, 32, 32)` and `conv4` has shape `(3, 128, 32, 32)`, then `concat2` will have shape `(3, 192, 32, 32)`.
相关问题
def forward(self, x): x = self.downsample_conv(x) x0 = self.split_conv0(x) x1 = self.split_conv1(x) x1 = self.blocks_conv(x1) x = torch.cat([x1, x0], dim=1) x = self.concat_conv(x) return x这段代码的含义
这段代码定义了一个前向传播的函数,其中输入的参数 `x` 是一个张量,代表着网络模型的输入。在函数中,首先通过一个下采样卷积层 `downsample_conv` 对输入 `x` 进行下采样,将其尺寸减小。然后,将下采样后的特征张量 `x` 分别输入到两个卷积层 `split_conv0` 和 `split_conv1` 中,得到两个特征张量 `x0` 和 `x1`。接下来,对 `x1` 进行多次残差块计算,通过 `blocks_conv` 中的 Residual Block 层来提取和增强特征。然后,将 `x0` 和 `x1` 进行拼接,得到一个新的特征张量 `x`。最后,将 `x` 输入到合并卷积层 `concat_conv` 中,对特征进行融合和加工,最终得到网络模型的输出结果。整个函数的作用就是将输入张量通过网络模型的各个层进行前向传播计算,并返回最终的输出结果。
用tensorflow的layers.Layer模块改写 class SpatialAttention(nn.Module): def init(self): super(SpatialAttention, self).init() self.conv1 = nn.Conv2d(2, 1, 3, padding = 1, bias=False) self.sigmoid = nn.Sigmoid() def forward(self, x): avg_out = torch.mean(x, dim = 1, keepdim = True) max_out, _ = torch.max(x, dim = 1, keepdim = True) x = torch.cat([avg_out, max_out], dim = 1) x = self.conv1(x) return self.sigmoid(x)
以下是使用TensorFlow的layers.Layer模块改写的代码:
```python
import tensorflow as tf
class SpatialAttention(tf.keras.layers.Layer):
def __init__(self):
super(SpatialAttention, self).__init__()
self.conv1 = tf.keras.layers.Conv2D(1, 3, padding='same', use_bias=False)
self.sigmoid = tf.keras.layers.Activation('sigmoid')
def call(self, inputs):
avg_out = tf.reduce_mean(inputs, axis=1, keepdims=True)
max_out = tf.reduce_max(inputs, axis=1, keepdims=True)
x = tf.concat([avg_out, max_out], axis=1)
x = self.conv1(x)
return self.sigmoid(x)
```
在TensorFlow的layers.Layer模块中,我们使用`__init__()`方法来初始化层的参数,使用`call()`方法来定义层的前向传播逻辑。`Conv2D`和`Activation`分别对应PyTorch中的`nn.Conv2d`和`nn.Sigmoid`。`reduce_mean`和`reduce_max`分别对应PyTorch中的`torch.mean`和`torch.max`。`concat`用于在给定维度上连接不同的张量。
阅读全文
相关推荐
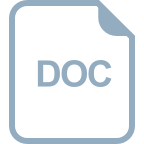
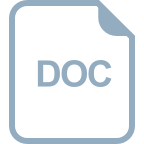
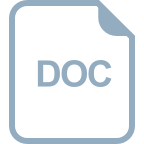













