a_flag = a_flag.masked_fill(a_flag != 0., float(-10000.0))
时间: 2024-04-01 12:35:14 浏览: 23
这是一个使用 PyTorch 的函数 masked_fill() 对 tensor a_flag 进行赋值的操作,结果仍然存储在变量 a_flag 中。具体来说,这个函数会将 a_flag 中所有不等于 0 的元素赋值为 -10000.0,而等于 0 的元素不变。这个操作通常是为了将某些值屏蔽掉,比如在自然语言处理中将 padding 的部分掩盖掉,或者在注意力机制中将不重要的部分掩盖掉。
相关问题
这段代码有什么错误def forward(self,x): num_nodes = x.size(1) # sub_graph size batch_size = x.size(0) W = torch.cat([self.W] * batch_size, dim=0) representation = torch.matmul(x, W) r_sum = torch.sum(representation, dim=-1, keepdim=False) b = torch.zeros([batch_size, num_nodes]) b = Variable(b) one = torch.ones_like(r_sum) zero = torch.zeros_like(r_sum) label = torch.clone(r_sum) label = torch.where(label == 0, one, zero) b.data.masked_fill_(label.bool(), -float('inf')) num_iterations = 3 for i in range(num_iterations): c = torch.nn.functional.softmax(b, dim=-1) weight_coeff = c.unsqueeze(dim=1) representation_global = torch.matmul(weight_coeff, representation) representation_global_all = torch.cat([representation_global] * num_nodes, dim=1) representation_similarity = torch.nn.functional.cosine_similarity(representation, representation_global_all, dim=-1) representation_similarity.data.masked_fill_(label.bool(), -float('inf')) b = representation_similarity return representation_global.squeeze(dim=1)
这段代码中存在一个错误。在for循环中,代码更新了变量b,但是在更新后没有再次进行softmax操作,导致后续的计算结果不正确。因此,需要在for循环中,在更新b后再次进行softmax操作,如下所示:
```
for i in range(num_iterations):
c = torch.nn.functional.softmax(b, dim=-1)
weight_coeff = c.unsqueeze(dim=1)
representation_global = torch.matmul(weight_coeff, representation)
representation_global_all = torch.cat([representation_global] * num_nodes, dim=1)
representation_similarity = torch.nn.functional.cosine_similarity(representation, representation_global_all, dim=-1)
representation_similarity.data.masked_fill_(label.bool(), -float('inf'))
b = torch.nn.functional.softmax(representation_similarity, dim=-1) # 进行softmax操作
```
这样修改后,该段代码的功能应该能够正常运行。
.masked_fill
.masked_fill()是PyTorch张量的一个方法,用于根据给定的掩码(mask)填充张量中的值。
具体来说,.masked_fill(mask, value)方法将张量中与掩码(mask)中对应位置为True的元素替换为给定的值(value),并返回替换后的新张量。
例如,假设有一个形状为(3, 3)的张量x:
```
x = torch.tensor([[1, 2, 3],
[4, 5, 6],
[7, 8, 9]])
```
我们想要将x中大于5的元素替换为0,可以定义一个相同形状的掩码(mask):
```
mask = torch.tensor([[False, False, False],
[False, False, True],
[True, True, True]])
```
然后使用.masked_fill()方法进行替换:
```
new_x = x.masked_fill(mask, 0)
```
最终得到的new_x为:
```
tensor([[1, 2, 3],
[4, 5, 0],
[0, 0, 0]])
```
可以看到,x中大于5的元素被替换为了0,而其他位置的元素保持不变。
相关推荐
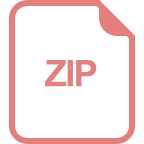













