帮我用python实现ViT
时间: 2023-07-12 19:16:31 浏览: 67
好的,以下是用Python实现ViT的代码:
首先,需要安装一些必要的库,包括PyTorch、torchvision和einops。可以使用以下命令进行安装:
```
pip install torch torchvision einops
```
接下来,可以使用以下代码定义ViT模型:
```python
import torch
import torch.nn as nn
import torch.nn.functional as F
from einops.layers.torch import Rearrange
class ViT(nn.Module):
def __init__(self, image_size, patch_size, num_classes, dim, depth, heads, mlp_dim):
super(ViT, self).__init__()
self.patch_size = patch_size
num_patches = (image_size // patch_size) ** 2
patch_dim = 3 * patch_size ** 2
self.patch_embedding = nn.Sequential(
Rearrange('b c (h p1) (w p2) -> b (h w) (p1 p2 c)', p1=patch_size, p2=patch_size),
nn.Linear(patch_dim, dim),
)
self.transformer = nn.ModuleList([
nn.TransformerEncoderLayer(d_model=dim, nhead=heads, dim_feedforward=mlp_dim)
for _ in range(depth)
])
self.classification_head = nn.Linear(dim, num_classes)
def forward(self, x):
x = self.patch_embedding(x)
b, n, _ = x.shape
x = x.permute(1, 0, 2)
for transformer in self.transformer:
x = transformer(x)
x = x.permute(1, 0, 2)
x = x.mean(dim=1)
x = self.classification_head(x)
return x
```
在此代码中,我们首先定义了一个 patch_embedding 层,用于将输入图像的块展平并传入一个全连接层。然后,我们定义了一个 Transformer 编码器,该编码器由多个 TransformerEncoderLayer 组成。最后,我们添加了一个分类头,用于预测输入图像的类别。
接下来,可以使用以下代码进行模型的实例化和训练:
```python
import torch.optim as optim
import torchvision.datasets as datasets
import torchvision.transforms as transforms
# Define hyperparameters
image_size = 224
patch_size = 16
num_classes = 10
dim = 256
depth = 6
heads = 8
mlp_dim = 512
lr = 1e-3
batch_size = 128
num_epochs = 10
# Initialize the model
model = ViT(image_size=image_size, patch_size=patch_size, num_classes=num_classes, dim=dim, depth=depth, heads=heads, mlp_dim=mlp_dim)
# Load the CIFAR-10 dataset
train_dataset = datasets.CIFAR10(root='data', train=True, transform=transforms.ToTensor(), download=True)
test_dataset = datasets.CIFAR10(root='data', train=False, transform=transforms.ToTensor(), download=True)
# Create data loaders
train_loader = torch.utils.data.DataLoader(train_dataset, batch_size=batch_size, shuffle=True)
test_loader = torch.utils.data.DataLoader(test_dataset, batch_size=batch_size, shuffle=False)
# Define the loss function and optimizer
criterion = nn.CrossEntropyLoss()
optimizer = optim.Adam(model.parameters(), lr=lr)
# Train the model
for epoch in range(num_epochs):
running_loss = 0.0
for i, data in enumerate(train_loader, 0):
inputs, labels = data
optimizer.zero_grad()
outputs = model(inputs)
loss = criterion(outputs, labels)
loss.backward()
optimizer.step()
running_loss += loss.item()
if i % 100 == 99:
print('[%d, %5d] loss: %.3f' % (epoch + 1, i + 1, running_loss / 100))
running_loss = 0.0
# Test the model
correct = 0
total = 0
with torch.no_grad():
for data in test_loader:
images, labels = data
outputs = model(images)
_, predicted = torch.max(outputs.data, 1)
total += labels.size(0)
correct += (predicted == labels).sum().item()
print('Accuracy of the network on the 10000 test images: %d %%' % (100 * correct / total))
```
在此代码中,我们首先定义了模型的超参数,然后使用 CIFAR-10 数据集进行训练和测试。在训练过程中,我们使用交叉熵损失函数和 Adam 优化器进行优化。在测试过程中,我们计算了模型的准确率。
这就是使用 Python 实现 ViT 的完整代码。
相关推荐
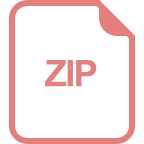
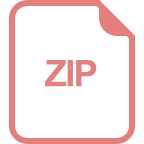














