解释 if len(det): # Rescale boxes from img_size to im0 size det[:, :4] = scale_coords(img.shape[2:], det[:, :4], im0.shape).round()
时间: 2024-04-03 07:33:50 浏览: 26
这段代码的作用是将预测框的坐标从输入图像尺寸 `img_size` 转换为原始图像尺寸 `im0 size`,以便后续的可视化。
`len(det)` 表示当前预测框列表不为空,即当前图像中存在预测框。如果存在预测框,则需要进行坐标转换。
`scale_coords()` 是一个 YOLOv5 工具函数,用于将预测框的坐标从 `img_size` 转换为 `im0 size`。其中,`img.shape[2:]` 表示输入图像的尺寸,`det[:, :4]` 表示预测框的坐标信息。处理结果是一个更新后的预测框信息张量,其中预测框的坐标已经从 `img_size` 转换为 `im0 size`。
这里使用了 `.round()` 方法对预测框的坐标进行四舍五入,以保证坐标值为整数。
相关问题
if len(det): # Rescale boxes from img_size to im0 size这个可以怎么改
如果您想更改这一行代码,可以使用以下方法修改:
```python
if len(det):
# Rescale boxes from img_size to original image size
det[:, :4] = scale_coords(img.shape[2:], det[:, :4], im0.shape).round()
```
在这段代码中,`scale_coords` 函数的作用是将检测框的坐标从输入图像的大小(`img_size`)缩放到原始图像的大小(`im0`),以便在原始图像上显示检测结果。
如果您想更改缩放的方式,可以修改 `scale_coords` 函数的实现。以下是 `scale_coords` 函数的示例代码:
```python
def scale_coords(img1_shape, coords, img0_shape):
# img1_shape: (height, width)
# coords: [[x1, y1, x2, y2, conf, cls], ...]
# img0_shape: (height, width)
# 计算缩放比例
gain = max(img1_shape) / max(img0_shape)
pad = (img1_shape[1] - img0_shape[1] * gain) / 2, (img1_shape[0] - img0_shape[0] * gain) / 2
# 对检测框坐标进行缩放和平移
coords[:, [0, 2]] -= pad[0]
coords[:, [1, 3]] -= pad[1]
coords[:, :4] /= gain
# 对检测框坐标进行裁剪
coords[:, :4] = clip_coords(coords[:, :4], img0_shape)
return coords
```
在这个示例代码中,`scale_coords` 函数首先计算缩放比例 `gain` 和平移量 `pad`,然后对检测框坐标进行缩放和平移操作。您可以根据您的需求修改这个函数的实现,以实现不同的缩放方式。
if len(det): # Rescale boxes from img_size to im0 size这个把注释掉的那行怎么加进去
如果您想将注释掉的那行代码添加回去,可以将以下代码:
```python
if len(det):
# Rescale boxes from img_size to original image size
det[:, :4] = scale_coords(img.shape[2:], det[:, :4], im0.shape).round()
```
改为以下代码:
```python
if len(det):
# Rescale boxes from img_size to original image size
det[:, :4] = scale_coords(img.shape[2:], det[:, :4], im0.shape).round()
# Annotate detections
for *xyxy, conf, cls in reversed(det):
c = int(cls) # integer class
label = f'{names[c]} {conf:.2f}'
plot_one_box(xyxy, im0, label=label, color=colors(c, True), line_thickness=3)
```
这样就可以将注释掉的那行代码添加回去,同时保留原有代码中的绘制检测框的操作。修改后的代码会将目标检测结果绘制在原始图像上,并显示在屏幕上。
相关推荐
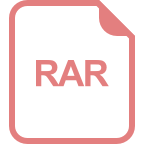
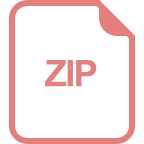
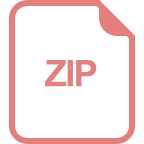












