1、实现稀疏矩阵(采用三元组表示)的基本运算目的:领会稀疏矩阵三元组存储结构及其基本算法设计。内容:假设n*n的稀疏矩阵A采用三元组表示,设计一个程序实现以下功能。(1)生成以下两个稀疏矩阵的三元组a和b。(2)输出a转置矩阵的三元组。(3)输出a+b的三元组。
时间: 2024-11-23 18:40:07 浏览: 71
实现稀疏矩阵(三元组表示)的基本运算目的是为了高效地处理大规模的数据,特别是那些大部分元素为零的情况,因为在标准密集矩阵存储中会浪费大量空间。以下是针对三元组表示(通常包含行索引、列索引和值)的操作:
1. **生成稀疏矩阵**:创建一个函数,接受矩阵的维度n作为输入,随机生成一定数量的非零元素,将其对应的行、列索引和值作为一个三元组列表存储。
```python
def generate_sparse_matrix(n):
non_zeros = random.randint(1, n * n // 10) # 随机选取非零元素的数量
matrix = []
for _ in range(non_zeros):
row = random.randint(0, n - 1)
col = random.randint(0, n - 1)
value = random.randint(-100, 100) # 随机数值
matrix.append((row, col, value))
return matrix
```
2. **转置矩阵**:对已有的矩阵,遍历每个元素,交换行索引和列索引,并保持原始值不变。
```python
def transpose_triples(matrix):
transposed = [(col, row, value) for (row, col, value) in matrix]
return transposed
```
3. **矩阵加法**:对于两个稀疏矩阵a和b,遍历它们的三元组,如果对应位置的行索引和列索引相同,则将值相加;否则,跳过。
```python
def sparse_add(a, b):
result = []
for triple_a in a:
for triple_b in b:
if triple_a[0] == triple_b[0] and triple_a[1] == triple_b[1]:
result.append((triple_a[0], triple_a[1], triple_a[2] + triple_b[2]))
break
return result
```
阅读全文
相关推荐
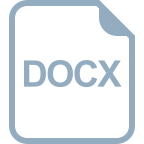
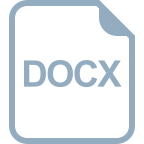
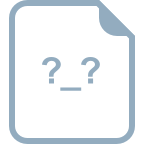















