def forward_up_features(self, x, x_downsample): for inx, layer_up in enumerate(self.layers_up): if inx == 0: x = layer_up(x) else: x = torch.cat([x,x_downsample[3-inx]],-1) x = self.concat_back_dim[inx](x) x = layer_up(x) x = self.norm_up(x) # B L C return x 以上代码的流程
时间: 2024-01-06 21:05:11 浏览: 109
以上代码是一个前向传播函数的实现,用于处理上采样特征。下面是代码的流程解释:
1. 输入参数:x是输入特征,x_downsample是下采样特征。
2. 使用for循环遍历self.layers_up中的每一层,其中self.layers_up是一个包含多个上采样层的列表。
3. 在循环中,如果inx为0,即第一次循环,将x输入到layer_up中进行上采样。
4. 如果inx不为0,即非第一次循环,将x与x_downsample[3-inx]进行拼接,其中3-inx用于索引x_downsample列表中对应的下采样特征。
5. 对拼接后的特征x使用self.concat_back_dim[inx]进行处理,这可能是一个维度恢复操作。
6. 将处理后的特征x输入到layer_up中进行上采样。
7. 循环结束后,将得到的特征x通过self.norm_up进行标准化处理。
8. 返回上采样后的特征x(大小为B L C),其中B为批量大小,L为序列长度,C为通道数。
总结起来,该代码实现了对输入特征进行多层上采样操作,并对上采样后的特征进行标准化处理,并返回最终的上采样特征。
相关问题
# New module: utils.pyimport torchfrom torch import nnclass ConvBlock(nn.Module): """A convolutional block consisting of a convolution layer, batch normalization layer, and ReLU activation.""" def __init__(self, in_chans, out_chans, drop_prob): super().__init__() self.conv = nn.Conv2d(in_chans, out_chans, kernel_size=3, padding=1) self.bn = nn.BatchNorm2d(out_chans) self.relu = nn.ReLU(inplace=True) self.dropout = nn.Dropout2d(p=drop_prob) def forward(self, x): x = self.conv(x) x = self.bn(x) x = self.relu(x) x = self.dropout(x) return x# Refactored U-Net modelfrom torch import nnfrom utils import ConvBlockclass UnetModel(nn.Module): """PyTorch implementation of a U-Net model.""" def __init__(self, in_chans, out_chans, chans, num_pool_layers, drop_prob, pu_args=None): super().__init__() PUPS.__init__(self, *pu_args) self.in_chans = in_chans self.out_chans = out_chans self.chans = chans self.num_pool_layers = num_pool_layers self.drop_prob = drop_prob # Calculate input and output channels for each ConvBlock ch_list = [chans] + [chans * 2 ** i for i in range(num_pool_layers - 1)] in_chans_list = [in_chans] + [ch_list[i] for i in range(num_pool_layers - 1)] out_chans_list = ch_list[::-1] # Create down-sampling layers self.down_sample_layers = nn.ModuleList() for i in range(num_pool_layers): self.down_sample_layers.append(ConvBlock(in_chans_list[i], out_chans_list[i], drop_prob)) # Create up-sampling layers self.up_sample_layers = nn.ModuleList() for i in range(num_pool_layers - 1): self.up_sample_layers.append(ConvBlock(out_chans_list[i], out_chans_list[i + 1] // 2, drop_prob)) self.up_sample_layers.append(ConvBlock(out_chans_list[-1], out_chans_list[-1], drop_prob)) # Create final convolution layer self.conv2 = nn.Sequential( nn.Conv2d(out_chans_list[-1], out_chans_list[-1] // 2, kernel_size=1), nn.Conv2d(out_chans_list[-1] // 2, out_chans, kernel_size=1), nn.Conv2d(out_chans, out_chans, kernel_size=1), ) def forward(self, x): # Down-sampling path encoder_outs = [] for layer in self.down_sample_layers: x = layer(x) encoder_outs.append(x) x = nn.MaxPool2d(kernel_size=2)(x) # Bottom layer x = self.conv(x) # Up-sampling path for i, layer in enumerate(self.up_sample_layers): x = nn.functional.interpolate(x, scale_factor=2, mode='bilinear', align_corners=True) x = torch.cat([x, encoder_outs[-(i + 1)]], dim=1) x = layer(x) # Final convolution layer x = self.conv2(x) return x
这里给出了一个 PyTorch 实现的 U-Net 模型,并且对代码进行了重构:
1. 将 ConvBlock 提取到了 utils.py 中,以便在 U-Net 中复用。
2. 在 U-Net 模型的 forward 方法中,使用了 ModuleList 对 down-sampling 和 up-sampling 层进行了封装,使得代码更加简洁和易于扩展。
3. 使用了 nn.functional.interpolate 对 feature map 进行了上采样,避免了使用 nn.ConvTranspose2d 带来的一些问题。
4. 在最后的卷积层中,使用了 nn.Sequential 对多个卷积层进行了封装,使得代码更加简洁。
这些重构都是为了提高代码的可读性、可维护性和可扩展性,使得代码更加清晰,易于理解和修改。
DenseNet网络代码
以下是一个简单的DenseNet网络实现代码示例:
```python
import torch
import torch.nn as nn
class DenseBlock(nn.Module):
def __init__(self, in_channels, growth_rate, num_layers):
super(DenseBlock, self).__init__()
self.layers = nn.ModuleList()
for i in range(num_layers):
self.layers.append(nn.Sequential(
nn.BatchNorm2d(in_channels + i * growth_rate),
nn.ReLU(inplace=True),
nn.Conv2d(in_channels + i * growth_rate, growth_rate,
kernel_size=3, stride=1, padding=1, bias=False)
))
def forward(self, x):
features = [x]
for layer in self.layers:
new_features = layer(torch.cat(features, dim=1))
features.append(new_features)
return torch.cat(features, dim=1)
class TransitionLayer(nn.Module):
def __init__(self, in_channels, out_channels):
super(TransitionLayer, self).__init__()
self.downsample = nn.Sequential(
nn.BatchNorm2d(in_channels),
nn.Conv2d(in_channels, out_channels, kernel_size=1, stride=1, bias=False),
nn.AvgPool2d(kernel_size=2, stride=2)
)
def forward(self, x):
return self.downsample(x)
class DenseNet(nn.Module):
def __init__(self, growth_rate=32, block_config=(6, 12, 24, 16), num_classes=1000):
super(DenseNet, self).__init__()
self.features = nn.Sequential(
nn.Conv2d(3, 64, kernel_size=7, stride=2, padding=3, bias=False),
nn.BatchNorm2d(64),
nn.ReLU(inplace=True),
nn.MaxPool2d(kernel_size=3, stride=2, padding=1)
)
num_features = 64
for i, num_layers in enumerate(block_config):
block = DenseBlock(num_features, growth_rate, num_layers)
self.features.add_module('denseblock%d' % (i + 1), block)
num_features = num_features + num_layers * growth_rate
if i != len(block_config) - 1:
trans = TransitionLayer(num_features, num_features // 2)
self.features.add_module('transition%d' % (i + 1), trans)
num_features = num_features // 2
self.avgpool = nn.AdaptiveAvgPool2d((1, 1))
self.classifier = nn.Linear(num_features, num_classes)
def forward(self, x):
features = self.features(x)
out = self.avgpool(features)
out = out.view(features.size(0), -1)
out = self.classifier(out)
return out
```
在这个实现中,我们定义了三个类:`DenseBlock`,`TransitionLayer`和`DenseNet`。`DenseBlock`实现了DenseNet中的密集块,`TransitionLayer`实现了DenseNet中的过渡层,而`DenseNet`则定义了整个网络的结构。
在`DenseNet`中,我们首先定义了一个序列模块,用于实现DenseNet的前几个卷积层和池化层。然后,我们循环遍历`block_config`中的元素,并在每个元素中定义一个密集块。如果这不是最后一个元素,则定义一个过渡层,将特征图的通道数减半,以便在下一个密集块中使用。最后,我们使用自适应平均池化层和线性分类器对特征图进行全局池化和分类。
这个实现代码可以自由修改以适应不同的数据集和任务。
阅读全文
相关推荐
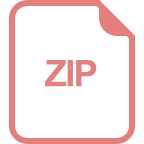
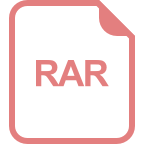
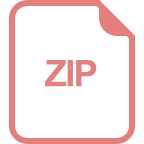













