def build_vocab(file_path, tokenizer, max_size, min_freq): vocab_dic = {} with open(file_path, 'r', encoding='UTF-8') as f: for line in tqdm(f): lin = line.strip() if not lin: continue content = lin.split('\t')[0] for word in tokenizer(content): vocab_dic[word] = vocab_dic.get(word, 0) + 1 vocab_list = sorted([_ for _ in vocab_dic.items() if _[1] >= min_freq], key=lambda x: x[1], reverse=True)[:max_size] vocab_dic = {word_count[0]: idx for idx, word_count in enumerate(vocab_list)} vocab_dic.update({UNK: len(vocab_dic), PAD: len(vocab_dic) + 1}) return vocab_dic
时间: 2024-04-28 12:20:59 浏览: 169
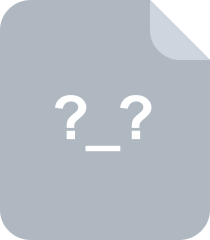
orb_vocab.dbow2
这段代码定义了一个函数 `build_vocab`,用于根据训练数据构建词表。具体来说,函数接受四个参数:
- `file_path`:训练数据文件的路径;
- `tokenizer`:用于将文本分割成单词或字的函数;
- `max_size`:词表的最大大小;
- `min_freq`:词汇在训练数据中出现的最小次数。
函数首先定义了一个空字典 `vocab_dic`,用于存储词汇及其出现次数。然后,函数使用 Python 内置的 `open` 函数打开训练数据文件,逐行读取文件内容,并将每行内容按照指定的分割函数 `tokenizer` 进行分割,统计分割后得到的每个词汇在训练数据中出现的次数,并将其存储到 `vocab_dic` 中。
接着,函数对 `vocab_dic` 中的词汇进行按出现次数降序排序,并选取出现次数最高的 `max_size` 个词汇作为词表。如果某个词汇在训练数据中出现次数小于 `min_freq`,则将其从词表中删除。函数将词表中的每个词汇与其在词表中的索引建立映射,并将其存储到一个新的字典 `vocab_dic` 中。在这里,函数还添加了两个特殊的词汇 `UNK` 和 `PAD`,用于表示未知词汇和填充词汇,这两个词汇的索引分别为词表的大小和词表大小加一。
最后,函数返回构建好的词表 `vocab_dic`。
阅读全文
相关推荐
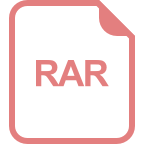
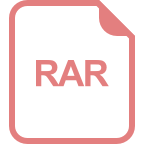




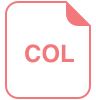
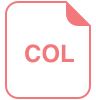
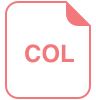
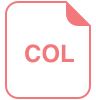
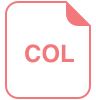
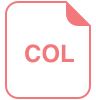
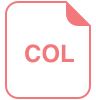
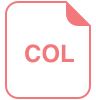
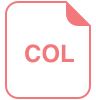
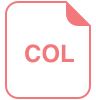
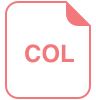