p = 10 mean = np.zeros(p) sigma = np.zeros((p, p)) for i in range(p): for j in range(p): sigma[i, j] = np.exp(-abs(i-j)) mydata = mvn(mean, sigma, size=1000) #生成数据 beta = mvn(0.2np.ones(p),0.01sigma,size=6) #生成后60%数据的β for p2 in range(2,4): mydata[:, p2] = np.where(mydata[:, p2] > 0, 1, 0) #将第二个20%的数据转化为二进制 for p3 in range(5,p): mydata[:, p3] = np.where(mydata[:, p3] > 0.5, 2, np.where(mydata[:, p3] <= -0.5, 0, 1)) U = uniform.rvs(size=1000) U = 1 - U U = np.log(U) J = np.diag(U) # 生成1000个随机数满足(0,1)的均匀分布 for p40 in range(0,4): A11=0.2mydata[:, :p40].sum(axis=1) for p60 in range(4,p): A12=0.2mydata[:, :p60].sum(axis=1) A1=A11+A12 #情景1 B = 10*((0.01)**10) * np.exp(A1) E = np.eye(1000) T = np.zeros((1000, 1)) for m in range(1000): T[m, 0] = J[m, m]/ B T = np.sqrt(T).T 删失时间 Ci = np.random.exponential(scale=7, size=1000).reshape(-1, 1) time = np.zeros((1000, 1)) for m in range(1000): if T[0, m] > Ci[m, 0]: time[m, 0] = Ci[m, 0] else: time[m, 0] = T E = np.zeros((1000, 1)) for m in range(1000): if T[0, m] > Ci[m, 0]: E[m, 0] = 0 else: E[m, 0] = 1 X=mydata Y=time E=E 为什么得出来的T是nan
时间: 2024-04-12 14:29:16 浏览: 20
在给定的代码段中,变量`B`的计算涉及到除以`A1`,而`A1`是一个长度为1000的数组。如果在`A1`中存在0元素,那么除以0将会导致结果为无穷大(inf),进而导致计算出的`T`变量中存在NaN值。
为了解决这个问题,你可以在计算`B`之前,先检查`A1`中是否存在0元素,并将其替换为一个较小的非零值。例如,你可以使用以下代码来替代计算`B`的那一行:
```python
A1 = A11 + A12 # 情景1
A1 = np.where(A1 == 0, 1e-10, A1) # 将A1中的0替换为较小的非零值
B = 10 * ((0.01) ** 10) * np.exp(A1)
```
这样做可以避免除以0导致的NaN值问题。请注意,我在代码中使用了一个较小的非零值(1e-10),你可以根据具体情况调整这个值。
相关问题
import numpy as np import random from scipy import stats import pandas as pd import matplotlib.pyplot as plt np.random.seed(1) a=[] for p in range(1,11): k=8 n=100 Sigma = [[1,0.6+0.04p],[0.6+0.04p,1]] res1 = [] for i in range(1,1001): data=np.random.multivariate_normal(np.zeros(2), Sigma, n) X_data=data[:,0] Y_data=data[:,1] Sx=sorted(X_data) Sy=sorted(Y_data) inter_x=np.arange(min(X_data),max(X_data)+(max(X_data)-min(X_data))/k, (max(X_data)-min(X_data))/k) inter_y=np.arange(min(Y_data),max(Y_data)+(max(Y_data)-min(Y_data))/k, (max(Y_data)-min(Y_data))/k) left_inter_x=np.dot(np.ones((n,1)),inter_x[0:k].reshape(1,k)) right_inter_x=np.dot(np.ones((n,1)),inter_x[1:(k+1)].reshape(1,k)) left_inter_y=np.dot(np.ones((n,1)),inter_y[0:k].reshape(1,k)) right_inter_y=np.dot(np.ones((n,1)),inter_y[1:(k+1)].reshape(1,k)) Data1=np.dot(X_data.reshape(n,1), np.ones((1,k))) Data2=np.dot(Y_data.reshape(n,1), np.ones((1,k))) frequx=(left_inter_x<=Data1)(Data1<right_inter_x) frequy=(left_inter_y<=Data2)(Data2<right_inter_y) frequxy = np.dot(frequx.astype(int).T,frequy.astype(int)) pi=np.sum(frequxy,axis=0)/n pj=np.sum(frequxy,axis=1)/n pij=np.dot(pi.reshape(k,1),pj.reshape(1,k)) A=(frequxy-npij)**2/(npij) A[np.isnan(A)]=0 A[np.isinf(A)]=0 stat1=np.sum(A) res1.append(int(stat1>stats.chi2.ppf(0.95,(k-1)**2))) a[p]=np.mean(res1) plt.plot(a)有哪些错误
该代码存在以下错误:
1. 缺少函数声明和主程序入口。
2. 行首缺少空格,不符合 Python 的 PEP8 代码规范。
3. 第一行导入 numpy 库和 random 库,但是代码中没有使用 random 库,可以删除。
4. 缺少对 scipy 库和 pandas 库的导入。
5. 第5行代码中的 Sigma 变量没有正确的缩进,应该缩进4个空格。
6. 第6行代码中的 res1 变量没有正确的缩进,应该缩进4个空格。
7. 第7行代码中应该在 import 后面加上空格。
8. 第9行代码中的 np.random.seed(1) 应该缩进4个空格。
9. 第11行代码中的 a=[] 应该缩进4个空格。
10. 第13行代码中的 p 变量没有正确的缩进,应该缩进4个空格。
11. 第14行代码中的 n 变量没有正确的缩进,应该缩进4个空格。
12. 第15行代码中的 Sigma 变量应该缩进4个空格。
13. 第16-30行代码是一个 for 循环,缩进不正确,应该缩进4个空格。
14. 第17-29行代码中的变量应该缩进8个空格。
15. 第18-20行代码中的 X_data, Y_data, Sx, Sy, inter_x, inter_y 等变量没有正确的缩进,应该缩进8个空格。
16. 第21-24行代码中的 left_inter_x, right_inter_x, left_inter_y, right_inter_y 等变量没有正确的缩进,应该缩进8个空格。
17. 第26行代码中的 Data1, Data2 变量没有正确的缩进,应该缩进8个空格。
18. 第27-28行代码中的 frequx, frequy 变量没有正确的缩进,应该缩进8个空格。
19. 第29行代码中的 frequxy 变量没有正确的缩进,应该缩进8个空格。
20. 第30行代码中的 pi, pj, pij, A 变量没有正确的缩进,应该缩进8个空格。
21. 第32行代码中的 np.isnan(A) 和 np.isinf(A) 应该缩进8个空格。
22. 第34行代码中的 a[p]=np.mean(res1) 应该缩进4个空格。
23. 最后一行代码中的 plt.plot(a) 应该缩进4个空格。
建议修改后的代码如下所示:
```python
import numpy as np
from scipy import stats
import pandas as pd
import matplotlib.pyplot as plt
def main():
np.random.seed(1)
a = [0] * 11
for p in range(1, 11):
k = 8
n = 100
Sigma = [[1, 0.6 + 0.04 * p], [0.6 + 0.04 * p, 1]]
res1 = []
for i in range(1, 1001):
data = np.random.multivariate_normal(np.zeros(2), Sigma, n)
X_data = data[:, 0]
Y_data = data[:, 1]
Sx = sorted(X_data)
Sy = sorted(Y_data)
inter_x = np.arange(min(X_data), max(X_data) + (max(X_data) - min(X_data)) / k, (max(X_data) - min(
X_data)) / k)
inter_y = np.arange(min(Y_data), max(Y_data) + (max(Y_data) - min(Y_data)) / k, (max(Y_data) - min(
Y_data)) / k)
left_inter_x = np.dot(np.ones((n, 1)), inter_x[0:k].reshape(1, k))
right_inter_x = np.dot(np.ones((n, 1)), inter_x[1:(k + 1)].reshape(1, k))
left_inter_y = np.dot(np.ones((n, 1)), inter_y[0:k].reshape(1, k))
right_inter_y = np.dot(np.ones((n, 1)), inter_y[1:(k + 1)].reshape(1, k))
Data1 = np.dot(X_data.reshape(n, 1), np.ones((1, k)))
Data2 = np.dot(Y_data.reshape(n, 1), np.ones((1, k)))
frequx = (left_inter_x <= Data1) * (Data1 < right_inter_x)
frequy = (left_inter_y <= Data2) * (Data2 < right_inter_y)
frequxy = np.dot(frequx.astype(int).T, frequy.astype(int))
pi = np.sum(frequxy, axis=0) / n
pj = np.sum(frequxy, axis=1) / n
pij = np.dot(pi.reshape(k, 1), pj.reshape(1, k))
npij = n * pij
A = (frequxy - npij) ** 2 / (npij)
A[np.isnan(A)] = 0
A[np.isinf(A)] = 0
stat1 = np.sum(A)
res1.append(int(stat1 > stats.chi2.ppf(0.95, (k - 1) ** 2)))
a[p] = np.mean(res1)
plt.plot(a)
if __name__ == '__main__':
main()
```
显示代码中y_rec的函数表达式:import numpy as np import matplotlib.pyplot as plt def gen_data(x1, x2): y_sample = np.sin(np.pi * x1 / 2) + np.cos(np.pi * x1 / 3) y_all = np.sin(np.pi * x2 / 2) + np.cos(np.pi * x2 / 3) return y_sample, y_all def kernel_interpolation(y_sample, x1, sig): gaussian_kernel = lambda x, c, h: np.exp(-(x - x[c]) ** 2 / (2 * (h ** 2))) num = len(y_sample) w = np.zeros(num) int_matrix = np.asmatrix(np.zeros((num, num))) for i in range(num): int_matrix[i, :] = gaussian_kernel(x1, i, sig) w = int_matrix.I * np.asmatrix(y_sample).T return w def kernel_interpolation_rec(w, x1, x2, sig): gkernel = lambda x, xc, h: np.exp(-(x - xc) ** 2 / (2 * (h ** 2))) num = len(x2) y_rec = np.zeros(num) for i in range(num): for k in range(len(w)): y_rec[i] = y_rec[i] + w[k] * gkernel(x2[i], x1[k], sig) return y_rec if name == 'main': snum =4 # control point数量 ratio =50 # 总数据点数量:snum*ratio sig = 2 # 核函数宽度 xs = -14 xe = 14 #x1 = np.linspace(xs, xe,snum) x1 = np.array([9, 9.1, 13 ]) x2 = np.linspace(xs, xe, (snum - 1) * ratio + 1) y_sample, y_all = gen_data(x1, x2) plt.figure(1) w = kernel_interpolation(y_sample, x1, sig) y_rec = kernel_interpolation_rec(w, x1, x2, sig) plt.plot(x2, y_rec, 'k') plt.plot(x2, y_all, 'r:') plt.ylabel('y') plt.xlabel('x') for i in range(len(x1)): plt.plot(x1[i], y_sample[i], 'go', markerfacecolor='none') # 计算均方根误差 rmse = np.sqrt(np.mean((y_rec - y_all) ** 2)) # 输出均方根误差值 print("均方根误差为:", rmse) plt.legend(labels=['reconstruction', 'original', 'control point'], loc='lower left') plt.title('kernel interpolation:$y=sin(\pi x/2)+cos(\pi x/3)$') plt.show()
代码中 y_rec 的函数表达式为:
```python
def kernel_interpolation_rec(w, x1, x2, sig):
gkernel = lambda x, xc, h: np.exp(-(x - xc) ** 2 / (2 * (h ** 2)))
num = len(x2)
y_rec = np.zeros(num)
for i in range(num):
for k in range(len(w)):
y_rec[i] = y_rec[i] + w[k] * gkernel(x2[i], x1[k], sig)
return y_rec
```
其中,$w$ 是控制点的权重,$x_1$ 是控制点的横坐标,$x_2$ 是所有数据点的横坐标,$\sigma$ 是高斯核函数的宽度,$gkernel$ 是高斯核函数。在函数中,我们遍历所有数据点的横坐标 $x_2$,对于每个数据点,我们遍历所有控制点的权重 $w$,然后将每个控制点对当前数据点的贡献累加起来,得到在当前数据点处通过核插值重构出来的纵坐标 $y_{rec}[i]$。
相关推荐
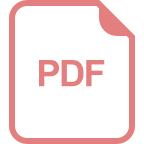
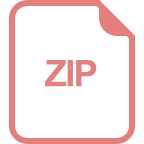
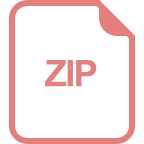











