解释cnn_model.compile
时间: 2024-01-13 19:24:55 浏览: 25
在Keras中,使用compile函数对模型进行编译,指定优化器、损失函数和评估指标等参数。在这个例子中,`cnn_model.compile`用于编译卷积神经网络模型。其中,参数optimizer指定了优化器的类型,如SGD、Adam等;参数loss指定了损失函数的类型;参数metrics指定了评估指标的类型,如accuracy、precision、recall等。此外,还可以设置其他的编译参数,如学习率、权重衰减系数等。编译模型后,就可以通过fit函数对模型进行训练,得到最终的模型参数。
相关问题
解释cnn_model.compile(loss='categorical_crossentropy', optimizer = SGD(learning_rate=1e-3,momentum=0.9),#SGD(lr=1e-3,momentum=0.9) metrics=['accuracy'])
这行代码是用来编译一个卷积神经网络(Convolutional Neural Network, CNN)模型的。下面是这行代码中每个参数的解释:
- `loss='categorical_crossentropy'`:这是损失函数的名称。在多分类问题中,交叉熵是一种广泛使用的损失函数。分类交叉熵(categorical_crossentropy)是一种在分类问题中使用的交叉熵损失函数。
- `optimizer=SGD(learning_rate=1e-3, momentum=0.9)`:这是优化器的名称和参数。在神经网络中,优化器用于最小化损失函数。这里使用的是随机梯度下降(Stochastic Gradient Descent, SGD)优化器。learning_rate参数定义了学习率,即每次更新权重时应该乘以的因子。momentum参数定义了动量,即应该如何使用之前的梯度来更新权重。
- `metrics=['accuracy']`:这是评估指标的名称。在训练过程中,我们需要一些指标来评估模型的性能。这里使用的是准确率(accuracy)作为评估指标。
def create_LSTM_model(): # instantiate the model model = Sequential() X_train = X_train.reshape((X_train.shape[0], n_steps, 1, n_length, n_features)) model.add(Input(shape=(X_train.shape[1], X_train.shape[2]))) # cnn1d Layers model.add(ConvLSTM2D(filters=64, kernel_size=(1,3), activation='relu', input_shape=(n_steps, 1, n_length, n_features))) model.add(Flatten()) model.add(RepeatVector(n_outputs)) model.add(MaxPooling1D()) # 添加lstm层 model.add(LSTM(64, activation = 'relu', return_sequences=True)) model.add(Dropout(0.5)) #添加注意力层 model.add(LSTM(64, activation = 'relu', return_sequences=False)) # 添加dropout model.add(Dropout(0.5)) model.add(Dense(128)) # 输出层 model.add(Dense(1, name='Output')) # 编译模型 model.compile(optimizer='adam', loss='mse', metrics=['mae']) return model # lstm network model = create_LSTM_model() # summary print(model.summary())修改该代码,解决UnboundLocalError Traceback (most recent call last) <ipython-input-21-b28a080066f3> in <module> 46 return model 47 # lstm network ---> 48 model = create_LSTM_model() 49 # summary 50 print(model.summary()) <ipython-input-21-b28a080066f3> in create_LSTM_model() 12 # instantiate the model 13 model = Sequential() ---> 14 X_train = X_train.reshape((X_train.shape[0], n_steps, 1, n_length, n_features)) 15 model.add(Input(shape=(X_train.shape[1], X_train.shape[2]))) 16 UnboundLocalError: local variable 'X_train' referenced before assignment问题
这个错误是因为 `X_train` 变量在函数内部被重新定义,但在 `X_train.reshape()` 被调用时,该变量尚未被赋值。解决方法是将 `X_train` 作为参数传递给函数,并在调用函数时将其传递。修改后的代码如下:
```
def create_LSTM_model(X_train):
# instantiate the model
model = Sequential()
X_train = X_train.reshape((X_train.shape[0], n_steps, 1, n_length, n_features))
model.add(Input(shape=(X_train.shape[1], X_train.shape[2])))
# cnn1d Layers
model.add(ConvLSTM2D(filters=64, kernel_size=(1,3), activation='relu', input_shape=(n_steps, 1, n_length, n_features)))
model.add(Flatten())
model.add(RepeatVector(n_outputs))
model.add(MaxPooling1D())
# 添加lstm层
model.add(LSTM(64, activation='relu', return_sequences=True))
model.add(Dropout(0.5))
#添加注意力层
model.add(LSTM(64, activation='relu', return_sequences=False))
# 添加dropout
model.add(Dropout(0.5))
model.add(Dense(128))
# 输出层
model.add(Dense(1, name='Output'))
# 编译模型
model.compile(optimizer='adam', loss='mse', metrics=['mae'])
return model
# lstm network
X_train = ...
model = create_LSTM_model(X_train)
# summary
print(model.summary())
```
相关推荐
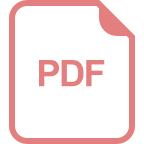










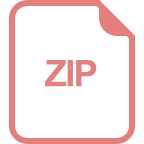