time_ctrl = ctrl.ControlSystem(rule) wash_time = ctrl.ControlSystemSimulation(time_ctrl)
时间: 2024-04-22 09:28:06 浏览: 5
根据你的代码,假设 `rule` 是一个规则集合(rule set),你可以通过以下代码创建名为 `time_ctrl` 的控制系统:
```python
time_ctrl = ctrl.ControlSystem(rule)
```
接下来,你可以使用 `time_ctrl` 创建一个名为 `wash_time` 的控制系统模拟对象,以便对控制系统进行模拟和输出计算:
```python
wash_time = ctrl.ControlSystemSimulation(time_ctrl)
```
现在,你可以使用 `wash_time` 对象来模拟控制系统并获取输出结果。请注意,上述代码是基于 Python 控制系统库(control system library)的示例,实际的使用方法可能因库的不同而有所变化。确保根据所使用的库的文档正确导入和使用相应的模块和类。
相关问题
current_time = datetime.datetime.day()
current_time = datetime.datetime.day()这段代码的目的是获取当前日期的天数。然而,这段代码是错误的,因为datetime.datetime.day()是一个方法,而不是属性。正确的方法是使用datetime.datetime.now().day来获取当前日期的天数。
#### 引用[.reference_title]
- *1* [c# datetime._C#| DateTime.Day属性与示例](https://blog.csdn.net/cumubi7453/article/details/107803576)[target="_blank" data-report-click={"spm":"1018.2226.3001.9630","extra":{"utm_source":"vip_chatgpt_common_search_pc_result","utm_medium":"distribute.pc_search_result.none-task-cask-2~all~insert_cask~default-1-null.142^v91^control_2,239^v3^insert_chatgpt"}} ] [.reference_item]
- *2* *3* [c# datetime._C#| DateTime.TimeOfDay属性(带示例)](https://blog.csdn.net/cumudi0723/article/details/107793933)[target="_blank" data-report-click={"spm":"1018.2226.3001.9630","extra":{"utm_source":"vip_chatgpt_common_search_pc_result","utm_medium":"distribute.pc_search_result.none-task-cask-2~all~insert_cask~default-1-null.142^v91^control_2,239^v3^insert_chatgpt"}} ] [.reference_item]
[ .reference_list ]
acceleration_cmd_closeloop = speed_pid_controller_.Control(speed_controller_input_limited, ts); debug->set_pid_saturation_status( speed_pid_controller_.IntegratorSaturationStatus()); if (enable_leadlag) { acceleration_cmd_closeloop = speed_leadlag_controller_.Control(acceleration_cmd_closeloop, ts); debug->set_leadlag_saturation_status( speed_leadlag_controller_.InnerstateSaturationStatus()); }
这段代码对闭环加速度命令值进行控制计算。
首先,使用speed_pid_controller_.Control()函数计算出速度PID控制器的输出值,并将其赋值给acceleration_cmd_closeloop。该函数使用speed_controller_input_limited和时间间隔ts作为参数进行控制计算。
接下来,通过调用speed_pid_controller_.IntegratorSaturationStatus()获取速度PID控制器的积分饱和状态,并将其设置到debug变量的pid_saturation_status字段中,用于调试或记录。
然后,如果enable_leadlag为true,表示启用了Leadlag控制,将使用speed_leadlag_controller_.Control()函数对acceleration_cmd_closeloop进行进一步的控制计算。该函数使用acceleration_cmd_closeloop和时间间隔ts作为参数进行控制计算,并将结果重新赋值给acceleration_cmd_closeloop。
最后,通过调用speed_leadlag_controller_.InnerstateSaturationStatus()获取速度Leadlag控制器的内部状态饱和状态,并将其设置到debug变量的leadlag_saturation_status字段中,用于调试或记录。
相关推荐
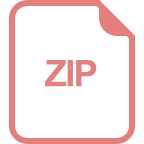
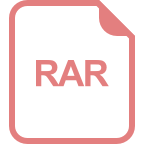













