y_data = [random.randint(100, 300) for i in range(6)]
时间: 2023-11-29 13:05:01 浏览: 49
这段代码是用列表推导式创建了一个包含随机整数元素的列表。这个列表中的元素是在100到300之间的随机整数,列表的长度为6。具体来说,这个列表中的元素是通过调用 Python 标准库中的 random 模块中的 randint 函数生成的。每次调用 randint 函数时,它会随机生成一个在100到300之间的整数,并将其作为列表的一个元素。最终得到的列表中有6个这样的元素。
相关问题
import cv2 import random import numpy as np img=cv2.imread("D:/CV_data/test/resize/1.jpg") percent =rand_point_percent=0.03 num = int(percent * img.shape[0] * img.shape[1]) for i in range(num): rand_x = random.randint(0, img.shape[0] - 1) rand_y = random.randint(0, img.shape[1] - 1) if random.randint(0, 1) == 0: img[rand_x, rand_y] = 0 else: img[rand_x, rand_y] = 255 cv2.imshow("output",img) cv2.waitKey() cv2.destroyAllWindows()
这段代码使用了OpenCV库进行图像处理。首先,通过cv2.imread函数读取了一张图片。然后,定义了一个变量percent表示随机点的比例,rand_point_percent表示随机点的比例。接着,计算了需要生成的随机点的数量num。然后,通过循环生成num个随机点,其中rand_x和rand_y分别表示随机点的横坐标和纵坐标。通过random.randint函数生成0到img.shape[0]-1和0到img.shape[1]-1之间的随机整数作为坐标值。如果random.randint(0, 1)的结果为0,则将对应位置的像素值设置为0,否则设置为255。最后,通过cv2.imshow函数显示处理后的图像,并通过cv2.waitKey和cv2.destroyAllWindows函数等待并关闭窗口。
请注意确保图像路径正确,并且已经安装了cv2和numpy库。如果有任何问题,请及时提出。
import tensorflow.compat.v1 as tf tf.disable_v2_behavior() import random n = 100 m = 216 x_data = [[random.randint(0, 9) for j in range(m)] for i in range(n)] y_data = [[random.randint(0, 9) for j in range(m)] for i in range(n)] constantV0 = tf.constant(0.0) jacobianmatrix1 = [] for j in range(int(m)): gradfunc = tf.gradients(x_data[:, j],y_data) # 此处xs为功率 print(gradfunc) for k in range(n): jacobianmatrix1.append(gradfunc[0][k, :]) jacobian_matrix2 = jacobianmatrix1 - constantV0
这段代码存在一些问题。首先,在第一行import语句中,应该使用`import tensorflow as tf`或者不使用别名,而不是使用`import tensorflow.compat.v1 as tf`,因为这样会导致后续代码中的一些TensorFlow API无法使用。
其次,在第二行应该加上分号`;`,否则会导致语法错误。
接下来,在第四行,`x_data`和`y_data`应该是TensorFlow的变量,而不是Python的列表。可以使用`tf.Variable`来定义它们。
在第七到第十行的代码中,`gradfunc`是一个TensorFlow的操作,它返回一个张量列表。因此,需要使用`sess.run()`方法来计算它的值。
最后,在第十一行,`jacobianmatrix1`应该是一个TensorFlow的张量,而不是Python的列表。可以使用`tf.stack()`方法将张量列表拼接成一个张量。
修正后的代码如下:
```python
import tensorflow as tf
import random
tf.disable_v2_behavior()
n = 100
m = 216
x_data = tf.Variable([[random.randint(0, 9) for j in range(m)] for i in range(n)])
y_data = tf.Variable([[random.randint(0, 9) for j in range(m)] for i in range(n)])
constantV0 = tf.constant(0.0)
jacobianmatrix1 = []
sess = tf.Session()
for j in range(int(m)):
gradfunc = tf.gradients(x_data[:, j], y_data)[0]
grad_value = sess.run(gradfunc)
for k in range(n):
jacobianmatrix1.append(grad_value[k, :])
jacobian_matrix2 = tf.stack(jacobianmatrix1) - constantV0
```
注意,这段代码只是计算了`jacobian_matrix2`的值,并没有输出或者保存结果。你需要根据需要进行修改。
相关推荐
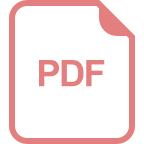
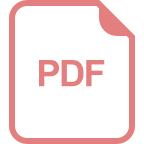
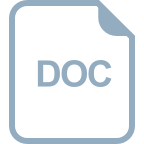













