def target_percent(): trace = go.Pie(labels = ['健康样本','患病样本'], values = data['Outcome'].value_counts(), textfont=dict(size=15), opacity = 0.8, marker=dict(colors=['lightskyblue', 'gold'], line=dict(color='#000000', width=1.5))) layout = dict(title = '样本构成') fig = dict(data = [trace], layout=layout) py.iplot(fig)
时间: 2024-04-27 17:21:11 浏览: 9
这段代码定义了一个函数 `target_percent()`,它使用 Plotly 库中的 `go.Pie()` 函数来绘制一个饼图,该饼图显示数据集中患病样本和健康样本的百分比。`values` 参数使用了 `data['Outcome'].value_counts()`,它返回了一个 Pandas Series,其中包含了 `Outcome` 列中各个取值的计数。`labels` 参数设置了饼图中各个部分的标签,`colors` 参数设置了各个部分的颜色,`textfont` 参数设置了文本的字体大小,`opacity` 参数设置了图形的透明度,`line` 参数设置了饼图中各个部分的边框线的颜色和宽度。最后,函数将饼图及其布局打包成字典,调用 Plotly 的 `iplot()` 函数将其绘制出来。
相关问题
def target_percent(): trace = go.Pie(labels = ['健康样本','患病样本'], values = data['Outcome'].value_counts(), textfont=dict(size=12), opacity = 0.8, marker=dict(colors=['lightskyblue', 'gold'], line=dict(color='#000000', width=2.0)))如何将这段代码的标题设置在中央
要将这段代码的标题设置在中央,你可以使用Plotly的layout属性来实现。具体的做法是在绘制图表之前,先设置一个layout,将它的title属性设置为你想要的标题,并将它的x和y属性都设置为0.5,如下所示:
```python
import plotly.graph_objs as go
def target_percent():
trace = go.Pie(labels = ['健康样本', '患病样本'],
values = data['Outcome'].value_counts(),
textfont=dict(size=12),
opacity = 0.8,
marker=dict(colors=['lightskyblue', 'gold'],
line=dict(color='#000000', width=2.0)))
layout = go.Layout(title='样本分布', title_font=dict(size=20), title_x=0.5, title_y=0.5)
fig = go.Figure(data=[trace], layout=layout)
fig.show()
```
在这个layout中,`title_x`和`title_y`属性都设置为0.5,表示将标题水平和垂直居中。如果你想要更改标题字体大小,可以使用`title_font`属性,将它的size属性设置为你想要的字体大小即可。
def target_percent(): trace = go.Pie(labels = ['healthy','diabetic'], values = data['Outcome'].value_counts(), textfont=dict(size=15), opacity = 0.8, marker=dict(colors=['lightskyblue', 'gold'], line=dict(color='#000000', width=1.5))) layout = dict(title = 'Distribution of Outcome variable') fig = dict(data = [trace], layout=layout) py.iplot(fig) p=sns.heatmap(data.corr(), annot=True,cmap ='hot')
这段代码看起来像是用Python的Plotly库和Seaborn库对数据进行可视化处理。其中,`go.Pie()`函数生成饼图,`data['Outcome'].value_counts()`是对数据中“Outcome”变量的统计,`sns.heatmap()`函数绘制热力图,`data.corr()`是对数据的相关性进行计算。这段代码的作用可能是展示数据的分布情况和相关性。
相关推荐
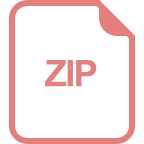
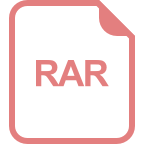
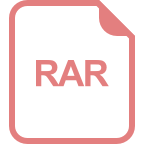














