def __init__(self, in_channels=3, features=[64, 128, 256, 512]): super().__init__() self.initial = nn.Sequential(
时间: 2023-11-14 09:05:32 浏览: 54
这是一个 PyTorch 中的类,用于创建一个卷积神经网络模型。这个模型包含了四个卷积块,每个卷积块包含了卷积层、批归一化层和激活函数层。
- in_channels:输入数据的通道数,对于 RGB 图像,通道数为 3。
- features:一个包含四个元素的列表,分别表示每个卷积块的输出通道数,也就是每个卷积块中卷积层输出的特征图的通道数。
在初始化函数中,首先定义了输入层,它包含了一个卷积层、一个批归一化层和一个激活函数层。接下来,定义了四个卷积块,每个卷积块都包含了卷积层、批归一化层和激活函数层。在每个卷积块中,卷积层的输入通道数等于上一个卷积块的输出通道数,卷积层的输出通道数等于当前卷积块的输出通道数。这样,随着网络的加深,特征图的通道数逐渐增加,可以提取更加复杂的特征。最后,定义了一个平均池化层和一个全连接层,用于对特征图进行降维和分类。
相关问题
把这段代码里的location_embedding_dim去掉class my_GAT(torch.nn.Module): def __init__(self, in_channels, out_channels, n_heads, location_embedding_dim, filters_1, filters_2, dropout): super(my_GAT, self).__init__() self.in_channels = in_channels self.out_channels = out_channels self.n_heads = n_heads location_embedding_dim = 0 self.filters_1 = filters_1 self.filters_2 = filters_2 self.dropout = dropout self.location_embedding_dim = location_embedding_dim self.setup_layers() def setup_layers(self): self.GAT_1 = GATConv(in_channels=self.in_channels,out_channels=self.filters_1, heads=self.n_heads, dropout=0.1) self.GAT_2 = GATConv(in_channels=self.filters_1 * self.n_heads + self.location_embedding_dim, out_channels=self.out_channels, heads=self.n_heads, dropout=0.1, concat=False) def forward(self, edge_indices, features, location_embedding): features = torch.cat((features, location_embedding), dim=-1) features = self.GAT_1(features, edge_indices) features = torch.nn.functional.relu(features) features = torch.nn.functional.dropout(features, p=self.dropout, training=self.training) features = torch.cat((features, location_embedding), dim=-1) features = self.GAT_2(features, edge_indices) return features
这段代码里的location_embedding_dim是一个参数,它表示位置嵌入的维度。如果你想去掉它,可以把它的值设为0,然后在构造函数中把self.location_embedding_dim也设为0即可。具体来说,修改后的代码如下:
```
class my_GAT(torch.nn.Module):
def __init__(self, in_channels, out_channels, n_heads, filters_1, filters_2, dropout):
super(my_GAT, self).__init__()
self.in_channels = in_channels
self.out_channels = out_channels
self.n_heads = n_heads
self.filters_1 = filters_1
self.filters_2 = filters_2
self.dropout = dropout
self.location_embedding_dim = 0
self.setup_layers()
def setup_layers(self):
self.GAT_1 = GATConv(in_channels=self.in_channels,out_channels=self.filters_1, heads=self.n_heads, dropout=0.1)
self.GAT_2 = GATConv(in_channels=self.filters_1 * self.n_heads, out_channels=self.out_channels, heads=self.n_heads, dropout=0.1, concat=False)
def forward(self, edge_indices, features, location_embedding):
features = self.GAT_1(features, edge_indices)
features = torch.nn.functional.relu(features)
features = torch.nn.functional.dropout(features, p=self.dropout, training=self.training)
features = self.GAT_2(features, edge_indices)
return features
```
请帮我编写下面网络的前向传播class UResNet_P(nn.Module):#ResNet_P编码器,参数量0.60M,595355 #def __init__(self): def __init__(self,in_channels=3, out_channels=3, init_features=32): super(UResNet_P,self).__init__() self.edge_detector=Edge_Detector() features = init_features self.pool = nn.MaxPool2d(kernel_size=2, stride=2) self.encoder1 = conv_block(in_channels, features) self.encoder2 = conv_block(features, features * 2) self.encoder3 = conv_block(features * 2, features * 4, dropout=True) self.residual_layer=self.stack_layer(Res_Block,16) self.conv=conv_block(features * 4, features * 8, dropout=True) self.upconv3 = nn.ConvTranspose2d(features * 8, features * 4, kernel_size=2, stride=2) self.decoder3 = conv_block(features * 8, features * 4) self.upconv2 = nn.ConvTranspose2d(features * 4, features * 2, kernel_size=2, stride=2) # 128 => 64 self.decoder2 = conv_block(features * 4, features * 2) self.upconv1 = nn.ConvTranspose2d(features * 2, features, kernel_size=2, stride=2) # 64 => 32 self.decoder1 = conv_block(features * 2, features) self.conv = nn.Conv2d(in_channels=features, out_channels=out_channels, kernel_size=1) self.input = nn.Conv2d(in_channels=3, out_channels=32, kernel_size=3, stride=1, padding=1, bias=False) self.output = nn.Conv2d(in_channels=32, out_channels=3, kernel_size=3, stride=1, padding=1, bias=False) self.relu=nn.ReLU(inplace=True)
好的,这是 `UResNet_P` 网络的前向传播代码:
```python
import torch.nn as nn
class UResNet_P(nn.Module):
def __init__(self, in_channels=3, out_channels=3, init_features=32):
super(UResNet_P, self).__init__()
self.edge_detector = Edge_Detector()
features = init_features
self.pool = nn.MaxPool2d(kernel_size=2, stride=2)
self.encoder1 = conv_block(in_channels, features)
self.encoder2 = conv_block(features, features * 2)
self.encoder3 = conv_block(features * 2, features * 4, dropout=True)
self.residual_layer = self.stack_layer(Res_Block, 16)
self.conv = conv_block(features * 4, features * 8, dropout=True)
self.upconv3 = nn.ConvTranspose2d(features * 8, features * 4, kernel_size=2, stride=2)
self.decoder3 = conv_block(features * 8, features * 4)
self.upconv2 = nn.ConvTranspose2d(features * 4, features * 2, kernel_size=2, stride=2)
self.decoder2 = conv_block(features * 4, features * 2)
self.upconv1 = nn.ConvTranspose2d(features * 2, features, kernel_size=2, stride=2)
self.decoder1 = conv_block(features * 2, features)
self.conv = nn.Conv2d(in_channels=features, out_channels=out_channels, kernel_size=1)
self.input = nn.Conv2d(in_channels=3, out_channels=32, kernel_size=3, stride=1, padding=1, bias=False)
self.output = nn.Conv2d(in_channels=32, out_channels=3, kernel_size=3, stride=1, padding=1, bias=False)
self.relu = nn.ReLU(inplace=True)
def forward(self, x):
edge = self.edge_detector(x)
x = self.input(x)
x = self.relu(x)
e1 = self.encoder1(x)
e2 = self.encoder2(self.pool(e1))
e3 = self.encoder3(self.pool(e2))
r = self.residual_layer(e3)
d = self.conv(torch.cat((e3, self.upconv3(r)), dim=1))
d = self.decoder3(d)
d = self.upconv2(d)
d = self.decoder2(torch.cat((e2, d), dim=1))
d = self.upconv1(d)
d = self.decoder1(torch.cat((e1, d), dim=1))
out = self.conv(d)
out = self.relu(out)
out = self.output(self.pool(out))
return out
```
这个网络包含了很多层,但是大部分都是相同的结构:卷积层 + ReLU 激活函数,或者是反卷积层。我这里只列出了 `conv_block` 和 `Res_Block` 的代码,因为这两个函数较为复杂,其他层都比较简单。你需要自己实现这两个函数,或者是从其他地方获取它们的代码。
相关推荐
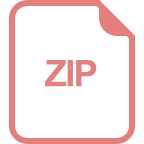
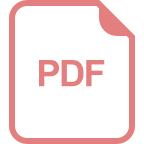
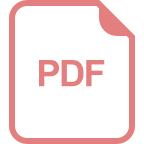













