我按照你的代码出现了这个问题: obs = train_collector.reset_one(i) AttributeError: 'Collector' object has no attribute 'reset_one'
时间: 2023-12-14 14:03:07 浏览: 76
这个错误提示说明在 `Collector` 类中没有 `reset_one` 这个方法,你可以检查一下你的代码,看看是不是写错了方法名或者是没有在 `Collector` 类中定义这个方法。如果确实没有定义这个方法,你可以考虑添加这个方法到 `Collector` 类中,或者使用其他已定义的方法来实现你的需求。如果你不确定该怎么做,可以提供更多代码细节,我可以帮你更好地解决这个问题。
相关问题
def train_model(stock_df, agent, num_episodes): for episode in range(num_episodes): obs = stock_df.iloc[0] state = get_state(obs) done = False total_reward = 0 while not done: action = agent.act(state) next_obs = stock_df.iloc[agent.current_step + 1] next_state = get_state(next_obs) reward = get_reward(action, obs, next_obs) total_reward += reward done = agent.current_step == len(stock_df) - 2 agent.learn(state, action, reward, next_state, done) state = next_state obs = next_obs # 输出每个episode的总奖励 print('Episode:', episode, 'Total Reward:', total_reward) # 逐渐降低探索率 agent.set_exploration_rate(agent.exploration_rate * 0.99)修改代码
这段代码中,训练模型时出现了错误,是因为 QLearningAgent 对象没有 current_step 属性,需要在 QLearningAgent 类中实现该属性。你可以在 QLearningAgent 类中添加 current_step 属性,并在 act 方法中更新该属性。例如:
```
class QLearningAgent:
def __init__(self, state_size, action_size):
self.state_size = state_size
self.action_size = action_size
self.q_table = np.zeros((state_size, action_size))
self.exploration_rate = 1.0
self.current_step = 0 # 新增 current_step 属性
def act(self, state):
if np.random.rand() < self.exploration_rate:
return np.random.choice(self.action_size)
q_values = self.q_table[state]
return np.argmax(q_values)
def learn(self, state, action, reward, next_state, done):
# 更新 Q 表
q_next = self.q_table[next_state]
if done:
q_next = np.zeros(self.action_size)
td_target = reward + GAMMA * np.max(q_next)
td_error = td_target - self.q_table[state, action]
self.q_table[state, action] += ALPHA * td_error
# 更新探索率和当前步数
self.current_step += 1
self.exploration_rate *= EXPLORATION_DECAY
self.exploration_rate = max(EXPLORATION_MIN, self.exploration_rate)
```
在修改后的代码中,我们在 QLearningAgent 类中新增了 current_step 属性,并在 act 方法和 learn 方法中更新该属性。最后,在训练模型时,我们可以使用 QLearningAgent 对象的 current_step 属性来获取当前步数,而不会再出现属性错误。
actor: torch.nn.Module, t_obs = torch.FloatTensor((train_collector.data.obs)[0]).unsqueeze(0).to(args.device) t_act = torch.FloatTensor((train_collector.data.act)[0]).unsqueeze(0).to(args.device) print(t_obs) print(t_act) writer.add_graph(policy.actor, t_obs)出现RuntimeError: Only tensors, lists, tuples of tensors, or dictionary of tensors can be output from traced functions错误,怎么改正代码
这个错误通常是由于`add_graph`方法不能接受`torch.nn.Module`类型的参数,而应该是一个计算图(`torch.onnx.export`)或一组张量。要修复这个错误,可以使用`torch.jit.trace`函数来创建一个跟踪模块,然后将跟踪模块的输入张量作为参数传递给`add_graph`方法。
以下是可能的解决方案,可以将其添加到代码中尝试:
```
# 使用torch.jit.trace创建跟踪模块
traced_actor = torch.jit.trace(policy.actor, (t_obs,))
# 将输入张量传递给add_graph方法
writer.add_graph(traced_actor, (t_obs,))
```
这应该可以解决该错误,允许您将模型添加到TensorBoard中。
阅读全文
相关推荐
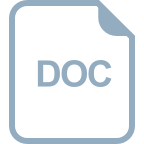
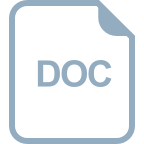
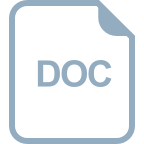














