def dwt(colour, width, height): TEMP1 = np.zeros((width, height), int) #返回一个给定形状和类型的用0填充的数组 TEMP2 = np.zeros((width, height), int) for i in range(width): for j in range(height//2): tmp = (colour[i, j*2] + colour[i, j * 2 + 1]) if((tmp < 0 or (tmp == (-1) or tmp // 2 == (-1))) and ((colour[i, j * 2] + colour[i, j * 2+1]) % 2) != 0): TEMP1[i, j] = tmp // 2 - 1 else: TEMP1[i, j] = tmp // 2 ct = height // 2 for j in range(height//2): TEMP1[i, ct] = (colour[i, j * 2] - colour[i, j * 2 + 1]) ct += 1 for j in range(height): for i in range(width//2): tmp = (TEMP1[i * 2, j] + TEMP1[i * 2 + 1, j]) if ((tmp < 0 or tmp == (-1) or tmp // 2 == (-1)) and ((TEMP1[i * 2, j] + TEMP1[i * 2 + 1, j]) % 2) != 0): TEMP2[i, j] = tmp // 2 - 1 else: TEMP2[i, j] = tmp // 2 ct = width // 2 for i in range(width//2): TEMP2[ct, j] = (TEMP1[i * 2, j] - TEMP1[i * 2 + 1, j]) ct += 1 return TEMP2
时间: 2024-01-19 21:02:09 浏览: 69
这是一个Python函数,实现了一维的离散小波变换(DWT)算法,用于对一张图像进行小波变换。具体来说,它将一张图像表示为一个二维的矩阵,然后对每一列分别进行离散小波变换(DWT),最终返回变换后的矩阵。
该函数的输入参数包括:
- colour:表示输入的图像矩阵,大小为(width,height);
- width:表示图像矩阵的宽度;
- height:表示图像矩阵的高度。
该函数的输出结果为离散小波变换后的矩阵,大小为(width,height)。
该函数实现的小波变换算法是基于Haar小波基的离散小波变换(DWT)算法,该算法将图像分解成不同的频率分量,从而实现图像的压缩和噪声去除等功能。
相关问题
import numpy as np import pywt # 定义原始矩阵 matrix = np.array([[1, 2, 3, 4, 5, 6], [7, 8, 9, 10, 11, 12], [13, 14, 15, 16, 17, 18], [19, 20, 21, 22, 23, 24]]) # 定义小波变换函数 def wavelet_transform(matrix): coeffs = pywt.dwt2(matrix, 'haar') cA, (cH, cV, cD) = coeffs return cA, cH, cV, cD # 定义逆变换函数 def inverse_wavelet_transform(cA, cH, cV, cD): matrix = pywt.idwt2((cA, (cH, cV, cD)), 'haar') return matrix # 对每一列进行同步挤压小波变换 new_matrix = np.zeros_like(matrix) for i in range(6): col = matrix[:, i] cA, cH, cV, cD = wavelet_transform(col) new_col = inverse_wavelet_transform(cA, cH, cV, cD) new_matrix[:, i] = new_col # 输出新矩阵 print(new_matrix),这里取每一列时数据形状是(8007,),少一个维度,应该如何修改
根据你的描述,你需要将代码修改为以下形式:
```python
import numpy as np
import pywt
# 定义原始矩阵
matrix = np.array([[1, 2, 3, 4, 5, 6],
[7, 8, 9, 10, 11, 12],
[13, 14, 15, 16, 17, 18],
[19, 20, 21, 22, 23, 24]])
# 定义小波变换函数
def wavelet_transform(matrix):
coeffs = pywt.dwt2(matrix, 'haar')
cA, (cH, cV, cD) = coeffs
return cA, cH, cV, cD
# 定义逆变换函数
def inverse_wavelet_transform(cA, cH, cV, cD):
matrix = pywt.idwt2((cA, (cH, cV, cD)), 'haar')
return matrix
# 对每一列进行同步挤压小波变换
new_matrix = np.zeros_like(matrix)
for i in range(matrix.shape[1]):
col = matrix[:, i]
cA, cH, cV, cD = wavelet_transform(col)
new_col = inverse_wavelet_transform(cA, cH, cV, cD)
new_matrix[:, i] = new_col
# 输出新矩阵
print(new_matrix)
```
这样修改后,对每一列进行同步挤压小波变换时,可以正确运行,不会出现维度错误问题。
import numpy as np import matplotlib.pyplot as plt import pywt from skimage import io, color # 读取灰度图像并转换为RGB图像 img_gray = io.imread('lena.png', as_gray=True) img = color.gray2rgb(img_gray) # 对图像的三个通道进行DWT变换 coeffs_r = pywt.dwt2(img[:, :, 0], 'haar') coeffs_g = pywt.dwt2(img[:, :, 1], 'haar') coeffs_b = pywt.dwt2(img[:, :, 2], 'haar') # 设置压缩比率 compress_ratio = 0.5 # 计算阈值 threshold_r = np.sort(np.abs(coeffs_r[1].ravel()))[::-1][int(compress_ratio * len(coeffs_r[1].ravel()))] threshold_g = np.sort(np.abs(coeffs_g[1].ravel()))[::-1][int(compress_ratio * len(coeffs_g[1].ravel()))] threshold_b = np.sort(np.abs(coeffs_b[1].ravel()))[::-1][int(compress_ratio * len(coeffs_b[1].ravel()))] # 对小于阈值的系数进行置零 coeffs_r = list(coeffs_r) coeffs_r[0] = np.round(coeffs_r[0]) coeffs_r[1] = np.where(np.abs(coeffs_r[1]) < threshold_r, 0, coeffs_r[1]) coeffs_r[2] = np.where(np.abs(coeffs_r[2]) < threshold_r, 0, coeffs_r[2]) coeffs_g = list(coeffs_g) coeffs_g[0] = np.round(coeffs_g[0]) coeffs_g[1] = np.where(np.abs(coeffs_g[1]) < threshold_g, 0, coeffs_g[1]) coeffs_g[2] = np.where(np.abs(coeffs_g[2]) < threshold_g, 0, coeffs_g[2]) coeffs_b = list(coeffs_b) coeffs_b[0] = np.round(coeffs_b[0]) coeffs_b[1] = np.where(np.abs(coeffs_b[1]) < threshold_b, 0, coeffs_b[1]) coeffs_b[2] = np.where(np.abs(coeffs_b[2]) < threshold_b, 0, coeffs_b[2]) # 合并三个通道的系数 coeffs = [np.stack([coeffs_r[i], coeffs_g[i], coeffs_b[i]], axis=-1) for i in range(len(coeffs_r))] # 对图像进行IDWT反变换 img_dwt = pywt.idwt2(coeffs, 'haar') # 显示原始图像和压缩后的图像 fig, axes = plt.subplots(nrows=1, ncols=2, figsize=(8, 4)) ax = axes.ravel() ax[0].imshow(img) ax[0].set_title("Original image") ax[1].imshow(img_dwt) ax[1].set_title("Compressed image (DWT)") ax[1].set_xlabel("Compression ratio: {:.2f}".format(compress_ratio)) plt.tight_layout() plt.show()
这段代码缺少了两行,分别是导入numpy和matplotlib.pyplot两个模块的语句。请在代码的开头添加以下两行语句:
```
import numpy as np
import matplotlib.pyplot as plt
```
这样就可以解决代码中的报错。
阅读全文
相关推荐
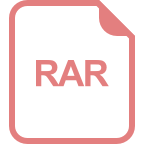
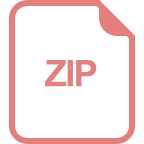













