aug_rot = (np.random.random() * 2 - 1) * self.max_rotation
时间: 2023-03-27 07:04:52 浏览: 67
这是一个关于 Python 代码的问题,我可以回答。这段代码是在进行数据增强时,对图像进行旋转的操作,其中 self.max_rotation 是最大旋转角度。具体实现可以参考 numpy 库中的 random 模块。
相关问题
改进这段代码import cv2 import numpy as np img = cv2.imread('E:\数字图像处理实验\数字图像处理1.jpg') gray = cv2.cvtColor(img, cv2.COLOR_BGR2GRAY) f = np.fft.fft2(gray) fshift = np.fft.fftshift(f) rows, cols = gray.shape crow, ccol = int(rows/2), int(cols/2) tx, ty = 50, 50 M = np.float32([[1, 0, tx], [0, 1, ty]]) fshift_trans = cv2.warpAffine(fshift, M, (cols, rows)) angle = 30 M = cv2.getRotationMatrix2D((ccol, crow), angle, 1) fshift_rot = cv2.warpAffine(fshift, M, (cols, rows)) fshift_center = fshift_trans[crow-100:crow+100, ccol-100:ccol+100] fshift_rot_center = fshift_rot[crow-100:crow+100, ccol-100:ccol+100] img_trans = np.fft.ifft2(np.fft.ifftshift(fshift_center)).real img_trans = np.uint8(img_trans) img_rot = np.fft.ifft2(np.fft.ifftshift(fshift_rot_center)).real img_rot = np.uint8(img_rot) cv2.imshow('gray', gray) cv2.imshow('img_trans', img_trans) cv2.imshow('img_rot', img_rot) cv2.waitKey(0) cv2.destroyAllWindows()
这段代码主要是对灰度图像进行图像平移和旋转,然后对平移和旋转后的图像进行傅里叶变换和傅里叶反变换,最后显示出平移和旋转后的图像。以下是代码改进的建议:
1. 路径中的反斜杠需要进行转义,或者使用正斜杠来代替,以避免出现路径错误。
2. 在使用傅里叶变换和傅里叶反变换时,可以使用numpy.fft.fft2()和numpy.fft.ifft2(),这样可以简化代码。
3. 在使用傅里叶变换和傅里叶反变换时,需要进行数据类型转换,可以使用numpy.uint8()将复数数组转换为无符号8位整数数组。
4. 在使用cv2.imshow()显示图像时,可以使用cv2.waitKey()来等待键盘输入,或者使用cv2.destroyAllWindows()来关闭所有窗口。
改进后的代码如下:
```
import cv2
import numpy as np
img = cv2.imread('E:/数字图像处理实验/数字图像处理1.jpg')
gray = cv2.cvtColor(img, cv2.COLOR_BGR2GRAY)
f = np.fft.fft2(gray)
fshift = np.fft.fftshift(f)
rows, cols = gray.shape
crow, ccol = int(rows/2), int(cols/2)
tx, ty = 50, 50
M = np.float32([[1, 0, tx], [0, 1, ty]])
fshift_trans = cv2.warpAffine(fshift, M, (cols, rows))
angle = 30
M = cv2.getRotationMatrix2D((ccol, crow), angle, 1)
fshift_rot = cv2.warpAffine(fshift, M, (cols, rows))
fshift_center = fshift_trans[crow-100:crow+100, ccol-100:ccol+100]
fshift_rot_center = fshift_rot[crow-100:crow+100, ccol-100:ccol+100]
img_trans = np.fft.ifft2(fshift_center).real
img_trans = np.uint8(img_trans)
img_rot = np.fft.ifft2(fshift_rot_center).real
img_rot = np.uint8(img_rot)
cv2.imshow('gray', gray)
cv2.imshow('img_trans', img_trans)
cv2.imshow('img_rot', img_rot)
cv2.waitKey(0)
cv2.destroyAllWindows()
```
检查这段代码import cv2 import numpy as np img = cv2.imread('E:\数字图像处理实验\数字图像处理1.jpg') gray = cv2.cvtColor(img, cv2.COLOR_BGR2GRAY) f = np.fft.fft2(gray) fshift = np.fft.fftshift(f) rows, cols = gray.shape crow, ccol = int(rows/2), int(cols/2) tx, ty = 50, 50 M = np.float32([[1, 0, tx], [0, 1, ty]]) fshift_trans = cv2.warpAffine(fshift, M, (cols, rows)) angle = 30 M = cv2.getRotationMatrix2D((ccol, crow), angle, 1) fshift_rot = cv2.warpAffine(fshift, M, (cols, rows)) fshift_center = fshift_trans[crow-100:crow+100, ccol-100:ccol+100] fshift_rot_center = fshift_rot[crow-100:crow+100, ccol-100:ccol+100] img_trans = np.fft.ifft2(np.fft.ifftshift(fshift_center)).real img_trans = np.uint8(img_trans) img_rot = np.fft.ifft2(np.fft.ifftshift(fshift_rot_center)).real img_rot = np.uint8(img_rot) cv2.imshow('gray', gray) cv2.imshow('img_trans', img_trans) cv2.imshow('img_rot', img_rot) cv2.waitKey(0) cv2.destroyAllWindows()
这段代码是用于对一张灰度图像进行傅里叶变换,并对变换结果进行平移和旋转操作,最后再通过傅里叶反变换将变换后的图像转换回空间域。
具体步骤如下:
1. 读取一张灰度图像。
2. 对图像进行傅里叶变换。
3. 对变换结果进行平移操作,平移的距离是 (tx, ty)。
4. 对变换结果进行旋转操作,旋转的角度是 angle。
5. 将平移后的结果和旋转后的结果分别截取中心区域。
6. 对截取的结果进行傅里叶反变换,将其转换回空间域。
7. 将反变换后的结果转换为整型。
8. 显示原始图像、平移后的图像和旋转后的图像。
需要注意的是,进行傅里叶变换和反变换时,需要使用 np.fft.fft2 和 np.fft.ifft2 函数。而对变换结果进行平移和旋转时,则需要使用 cv2.warpAffine 函数。
相关推荐
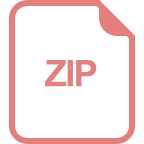
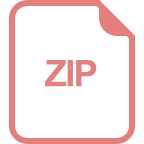













