cv::Mat undistorted_mat = cv::Mat::zeros(1, 3, CV_64FC2); cv::split(undistorted_mat, channels); channels[0] = undistorted_points_temp(cv::Rect(0, 0, 3, 1)); channels[1] = undistorted_points_temp(cv::Rect(0, 1, 3, 1)); cv::merge(channels, undistorted_mat);结果是什么
时间: 2024-04-05 12:34:55 浏览: 13
这段代码的作用是将一个大小为 1x3,数据类型为 CV_64FC2 的全零矩阵 `undistorted_mat` 中的第一个通道和第二个通道分别设置为 `undistorted_points_temp` 中 `(0,0)` 位置开始的大小为 3x1 的矩阵(即矩形区域 `(0,0,3,1)`),并将修改后的两个通道重新合并为一个矩阵 `undistorted_mat`。
具体来说,这段代码首先将 `undistorted_mat` 矩阵分离为两个通道,保存到数组 `channels` 中。然后,通过 `undistorted_points_temp(cv::Rect(0, 0, 3, 1))` 和 `undistorted_points_temp(cv::Rect(0, 1, 3, 1))` 分别获取 `undistorted_points_temp` 中 `(0,0)` 开始的大小为 3x1 的矩形区域,并将它们分别赋值给 `channels` 数组中的第一个通道和第二个通道。最后,使用 `cv::merge` 函数将修改后的两个通道重新合并为一个矩阵 `undistorted_mat`。
因此,执行完这段代码后,`undistorted_mat` 矩阵的第一个通道和第二个通道分别保存了 `undistorted_points_temp` 中 `(0,0)` 开始的大小为 3x1 的矩形区域,而第三个通道仍然是全零矩阵。
相关问题
std::vector<cv::Mat> channels(2); cv::Mat undistorted_points_temp = cv::Mat::ones(3, rows * cols, CV_64FC1); undistorted_points_temp = K.inv() * undistorted_points; cv::Mat undistorted_mat = cv::Mat::zeros(1, rows * cols, CV_64FC2); cv::s
std::vector<cv::Mat> channels(2);
cv::Mat undistorted_points_temp = cv::Mat::ones(3, rows * cols, CV_64FC1);
undistorted_points_temp = K.inv() * undistorted_points;
cv::Mat undistorted_mat = cv::Mat::zeros(1, rows * cols, CV_64FC2);
cv::split(undistorted_points_temp, channels);
cv::merge(channels, undistorted_mat);
以上代码实现了以下功能:
1. 定义了一个名为 channels 的 cv::Mat 类型的 vector,其大小为 2。
2. 创建了一个 3 行 rows*cols 列的 CV_64FC1 类型的矩阵 undistorted_points_temp,并将其所有元素初始化为 1。
3. 通过 K 的逆矩阵与 undistorted_points 相乘,得到一个新的矩阵 undistorted_points_temp。
4. 创建了一行 rows*cols 列的 CV_64FC2 类型的矩阵 undistorted_mat,并将其所有元素初始化为 0。
5. 将 undistorted_points_temp 矩阵拆分成多个通道,存储在名为 channels 的 vector 中。
6. 将 channels 中的通道合并成一个矩阵 undistorted_mat。
std::vector<cv::Mat> channels(2); cv::Mat undistorted_points_temp = cv::Mat::ones(3, rows * cols, CV_64FC1); undistorted_points_temp = K.inv() * undistorted_points; cv::Mat undistorted_mat = cv::Mat::zeros(1, rows * cols, CV_64FC2); cv::split(undistorted_mat, channels); channels[0] = undistorted_points_temp(cv::Rect(0, 0, rows * cols, 1));
这段代码的作用是将一个大小为 3x(rows * cols),数据类型为 CV_64FC1 的矩阵 `undistorted_points_temp` 通过矩阵运算转换成一个大小为 1x(rows * cols),数据类型为 CV_64FC2 的矩阵 `undistorted_mat` 并将其第一个通道设置为 `undistorted_points_temp` 的第一行。
具体来说,这段代码首先定义了一个包含两个元素的 `cv::Mat` 类型的向量 `channels`,其中每个元素都是一个矩阵。这个向量通常用于将一个多通道的矩阵分离为多个单通道矩阵,或者将多个单通道矩阵合并为一个多通道矩阵。
然后,创建一个大小为 3x(rows * cols),数据类型为 CV_64FC1 的矩阵 `undistorted_points_temp`,并将其通过矩阵运算 `K.inv() * undistorted_points` 转换为大小为 1x(rows * cols),数据类型为 CV_64FC2 的矩阵 `undistorted_mat`。其中,`K` 是一个 3x3 的相机内参矩阵,`inv()` 表示矩阵的逆运算,`undistorted_points` 是一个大小为 3x(rows * cols),数据类型为 CV_64FC1 的矩阵。
接下来,使用 `cv::split` 函数将 `undistorted_mat` 矩阵分离为两个单通道矩阵,并将这两个矩阵分别保存到 `channels` 向量中。然后,将 `undistorted_points_temp` 矩阵的第一行(大小为 1x(rows * cols),数据类型为 CV_64FC1)赋值给 `channels` 向量中的第一个通道(大小为 1x(rows * cols),数据类型为 CV_64FC1)。需要注意的是,在这里使用了 `cv::Rect` 类来指定矩阵中的子区域,其中 `(0, 0, rows * cols, 1)` 表示矩形左上角坐标为 (0, 0),宽度为 rows * cols,高度为 1。
执行完这段代码后,`channels` 向量中的第一个元素保存了大小为 1x(rows * cols),类型为 CV_64FC1 的 `undistorted_points_temp` 矩阵的第一行,而第二个元素仍然是全零矩阵。`undistorted_mat` 矩阵的第一个通道也保存了 `undistorted_points_temp` 矩阵的第一行,而第二个通道仍然是全零矩阵。
相关推荐
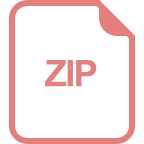
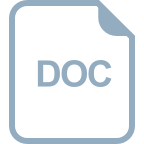
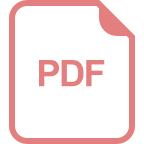
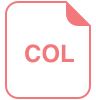

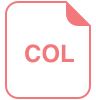









